Single Depth Image Super-Resolution And Denoising Based On Sparse Graphs Via Structure Tensor
2017 24TH IEEE INTERNATIONAL CONFERENCE ON IMAGE PROCESSING (ICIP)(2017)
摘要
The existing single depth image super-resolution (SR) methods suppose that the image to be interpolated is noise free. However, the supposition is invalid in practice because noise will be inevitably introduced in the depth image acquisition process. In this paper, we address the problem of image denoising and SR jointly based on designing sparse graphs that are useful for describing the geometric structures of data domains. In our method, we first cluster similar patches in a noisy depth image and compute an average patch. Different from the majority of the graph Fourier transform (GFT) that assumed an underlying 4-connected graph structure with vertical and horizontal edges only, we select more general sparse graph structures and edges weights based on the difference of the blocks' structure tensors. For the average patch, a graph template with edges orthogonal to the principal gradient is designed. Finally, the graph based transform (GBT) dictionary is learned from the derived correlation graph for signal representation. As shown in our experimental results, the proposed method obtains a lot of improvement in performance.
更多查看译文
关键词
Denoising, depth image, dictionary learning, graph signal processing, super-resolution
AI 理解论文
溯源树
样例
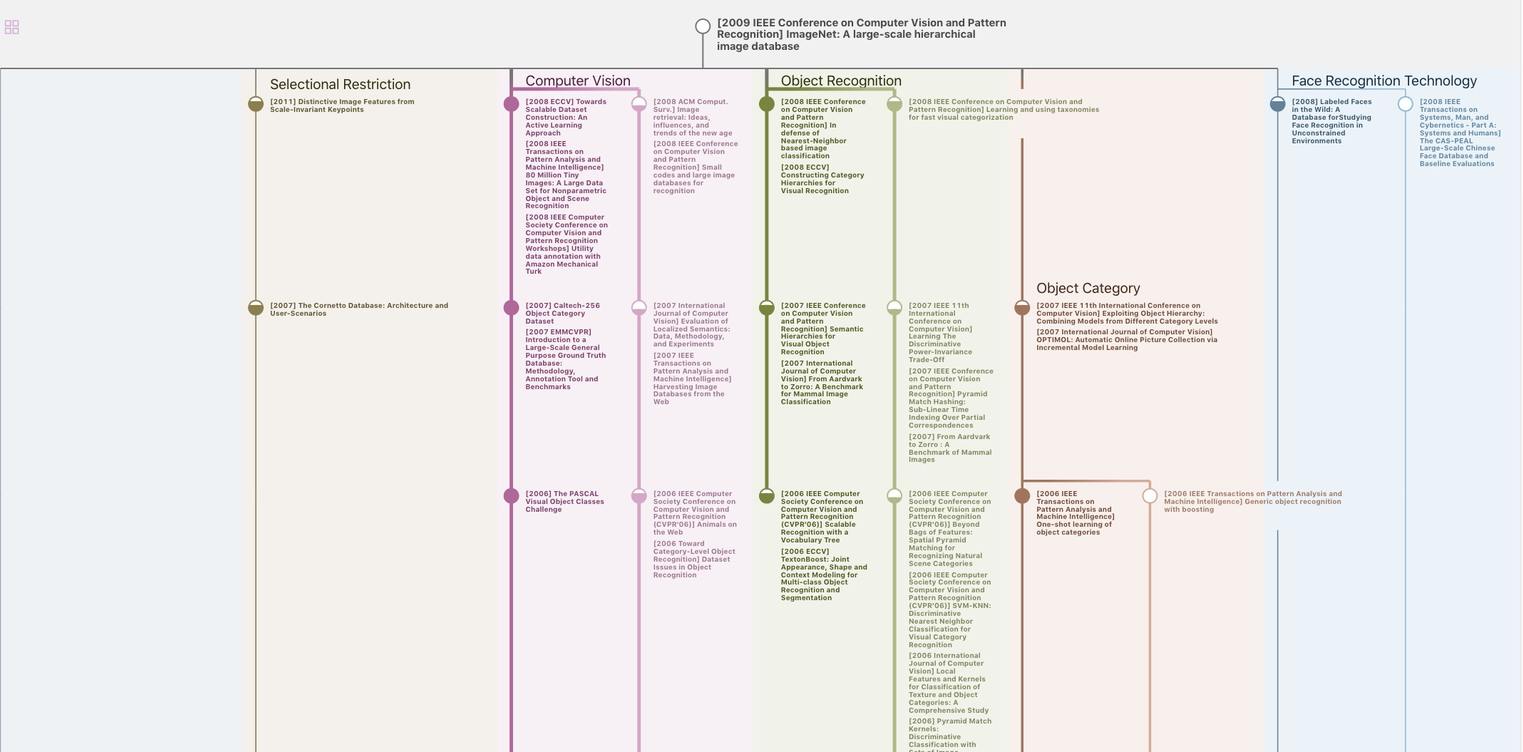
生成溯源树,研究论文发展脉络
Chat Paper
正在生成论文摘要