Residual Recurrent Neural Network With Sparse Training For Offline Arabic Handwriting Recognition
2017 14TH IAPR INTERNATIONAL CONFERENCE ON DOCUMENT ANALYSIS AND RECOGNITION (ICDAR), VOL 1(2017)
摘要
Deep Recurrent Neural Networks (RNN) have been suffering from the overfitting problem due to the model redundancy of the network structures. We propose a novel temporal and spatial residual learning method for RNN, followed with sparse training by weight pruning to gain sparsity in network parameters. For a Long Short-Term Memory (LSTM) network, we explore the combination schemes and parameter settings for temporal and spatial residual learning with sparse training. Experiments are carried out on the IFN/ENIT database. For the character error rate on the testing set e while training with sets a, b, c, d, the previously reported best result is 13.42%, and the proposed configuration of temporal residual learning followed with sparse training achieves the state-of-the-art result 12.06%.
更多查看译文
AI 理解论文
溯源树
样例
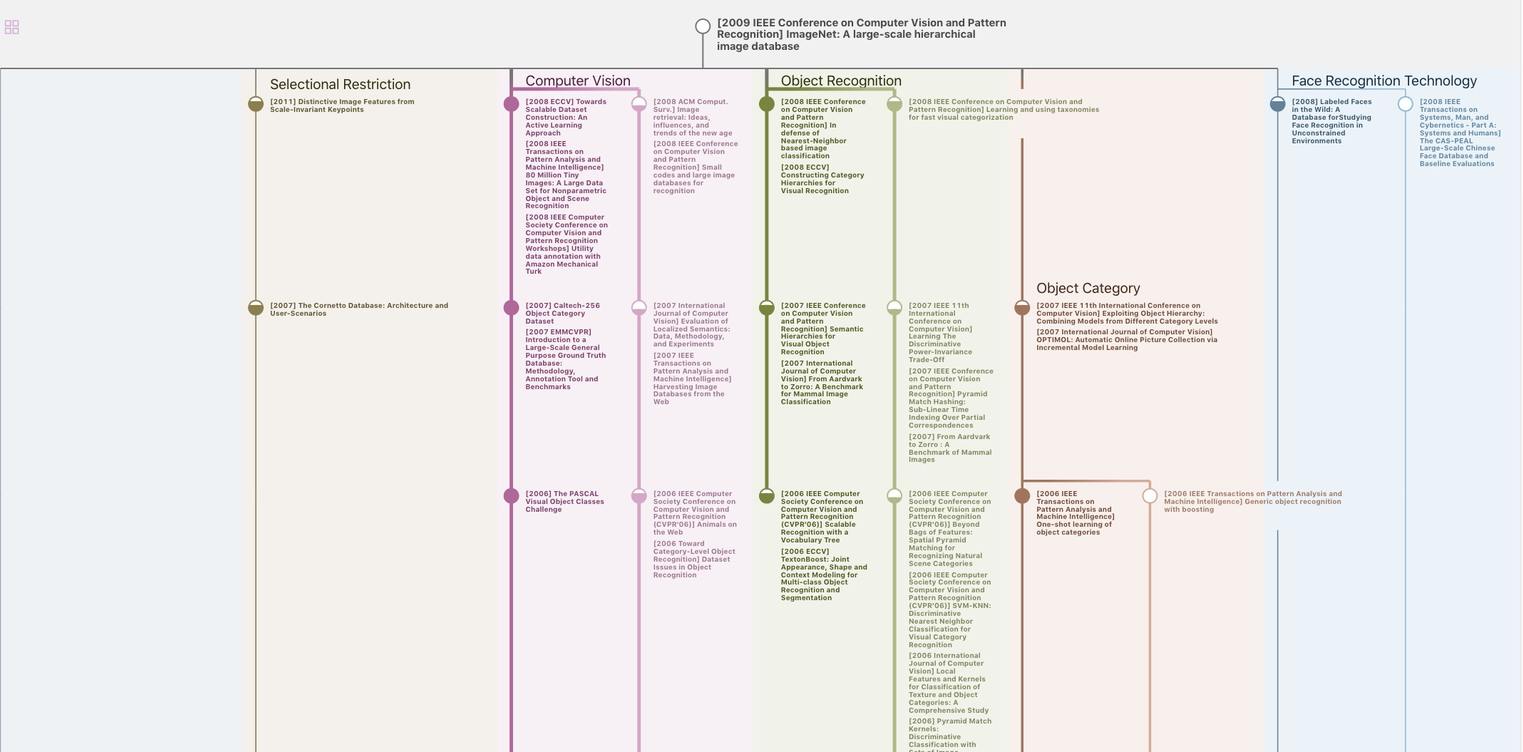
生成溯源树,研究论文发展脉络
Chat Paper
正在生成论文摘要