A Deep Learning-Based Formula Detection Method for PDF Documents
2017 14th IAPR International Conference on Document Analysis and Recognition (ICDAR)(2017)
摘要
In practice, PDF files may be generated by different tools and their character information quality could be different. As a result, the approaches to detecting formulae from PDF documents usually have much different performance on different PDF files. To address this problem, in this paper we combine and refine the Convolutional Neural Network (CNN) and Recurrent Neural Network (RNN) model to detect formulae according to both their character and vision features. Based on the characteristic of PDF documents, we propose a series of strategies to train and optimize deep networks, such as the implicit class down-sampling strategy which can reduce the unbalancedness between formulae and other page elements (e.g., text paragraphs, tables, figures, etc.). The region proposal method is also redesigned to generate moderate formula candidates through combining the bottom-up and top-down layout analysis. The experimental results show that the combination of CNN and RNN can increase the robustness of our proposed detection method. Furthermore, the proposed method outperforms the existing formula detection methods on both a ground-truth dataset and a larger self-built dataset, which would be released and available for research purposes.
更多查看译文
关键词
formula detection,deep learning,PDF documents
AI 理解论文
溯源树
样例
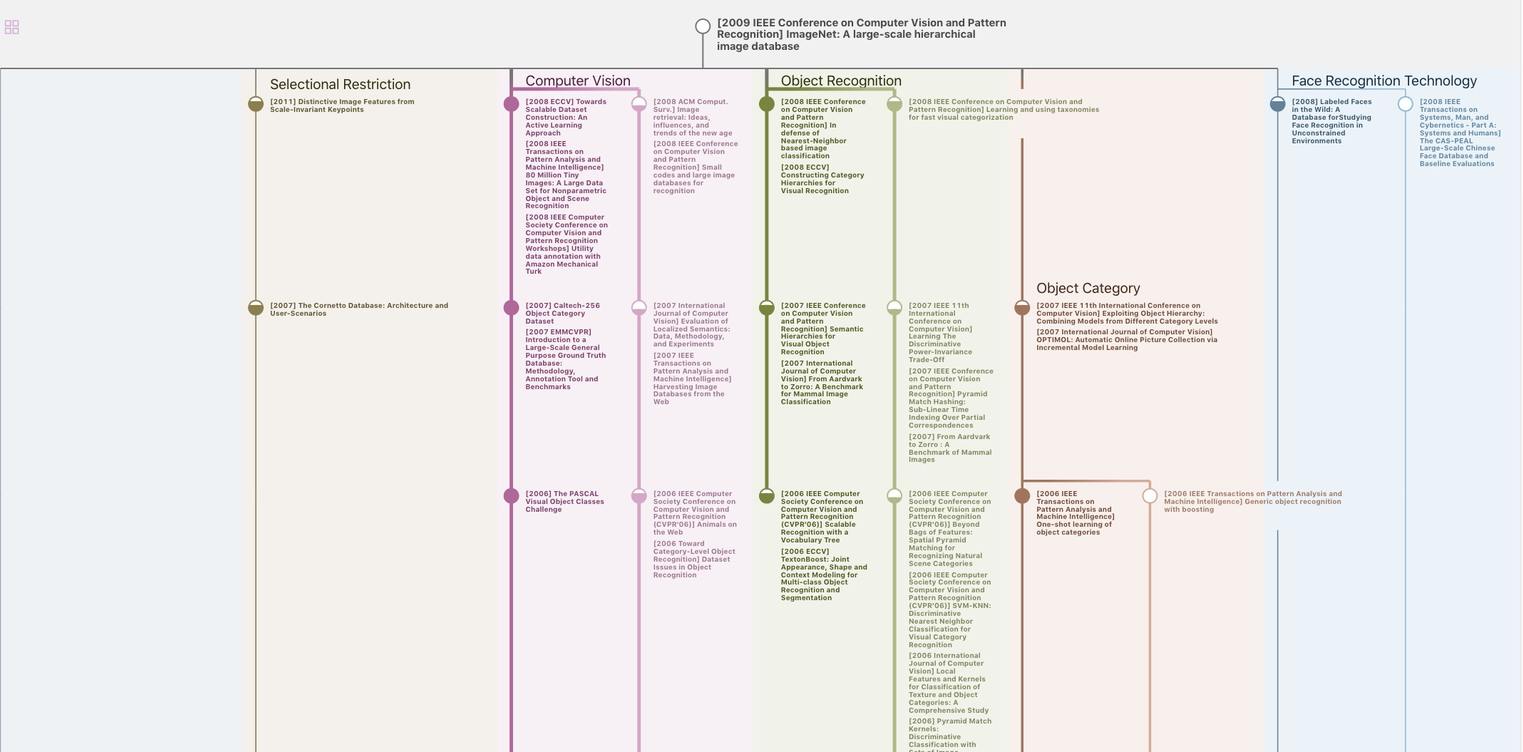
生成溯源树,研究论文发展脉络
Chat Paper
正在生成论文摘要