A deep reinforcement learning framework for optimizing fuel economy of hybrid electric vehicles.
ASP-DAC(2018)
摘要
Hybrid electric vehicles employ a hybrid propulsion system to combine the energy efficiency of electric motor and a long driving range of internal combustion engine, thereby achieving a higher fuel economy as well as convenience compared with conventional ICE vehicles. However, the relatively complicated powertrain structures of HEVs necessitate an effective power management policy to determine the power split between ICE and EM. In this work, we propose a deep reinforcement learning framework of the HEV power management with the aim of improving fuel economy. The DRL technique is comprised of an offline deep neural network construction phase and an online deep Q-learning phase. Unlike traditional reinforcement learning, DRL presents the capability of handling the high dimensional state and action space in the actual decision-making process, making it suitable for the HEV power management problem. Enabled by the DRL technique, the derived HEV power management policy is close to optimal, fully model-free, and independent of a prior knowledge of driving cycles. Simulation results based on actual vehicle setup over real-world and testing driving cycles demonstrate the effectiveness of the proposed framework on optimizing HEV fuel economy.
更多查看译文
关键词
deep reinforcement learning framework,hybrid electric vehicles,hybrid propulsion system,energy efficiency,electric motor,long driving range,internal combustion engine,higher fuel economy,conventional ICE vehicles,effective power management policy,power split,DRL technique,offline deep neural network construction phase,online deep Q-learning phase,high dimensional state,HEV power management problem,actual vehicle setup,HEV power management policy,HEV fuel economy optimization
AI 理解论文
溯源树
样例
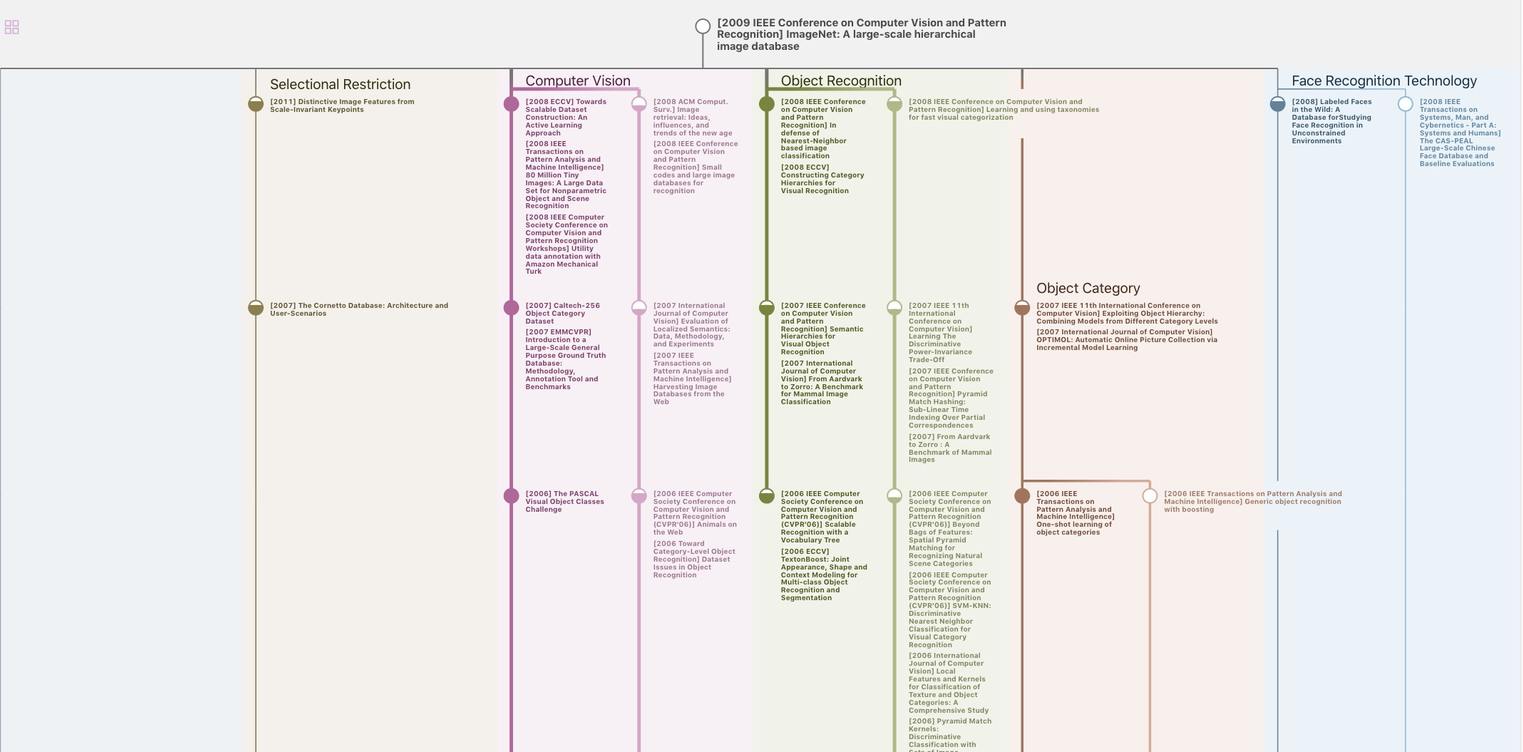
生成溯源树,研究论文发展脉络
Chat Paper
正在生成论文摘要