CrossFire: Cross Media Joint Friend and Item Recommendations.
WSDM 2018: The Eleventh ACM International Conference on Web Search and Data Mining Marina Del Rey CA USA February, 2018(2018)
摘要
Friend and item recommendation on a social media site is an important task, which not only brings conveniences to users but also benefits platform providers. However, recommendation for newly launched social media sites is challenging because they often lack user historical data and encounter data sparsity and cold-start problem. Thus, it is important to exploit auxiliary information to help improve recommendation performances on these sites. Existing approaches try to utilize the knowledge transferred from other mature sites, which often require overlapped users or similar items to ensure an effective knowledge transfer. However, these assumptions may not hold in practice because 1) Overlapped user set is often unavailable and costly to identify due to the heterogeneous user profile, content and network data, and 2) Different schemes to show item attributes across sites cause the attribute values inconsistent, incomplete, and noisy. Thus, how to transfer knowledge when no direct bridge is given between two social media sites remains a challenge. In addition, another auxiliary information we can exploit is the mutual benefit between social relationships and rating preferences within the platform. User-user relationships are widely used as side information to improve item recommendation, whereas how to exploit user-item interactions for friend recommendation is rather limited. To tackle these challenges, we propose aCross media jointF riend andI temRe commendation framework (CrossFire ), which can capture both 1) cross-platform knowledge transfer, and 2) within-platform correlations among user-user relations and user-item interactions. Empirical results on real-world datasets demonstrate the effectiveness of the proposed framework.
更多查看译文
关键词
Cross media recommendation, joint learning, data mining
AI 理解论文
溯源树
样例
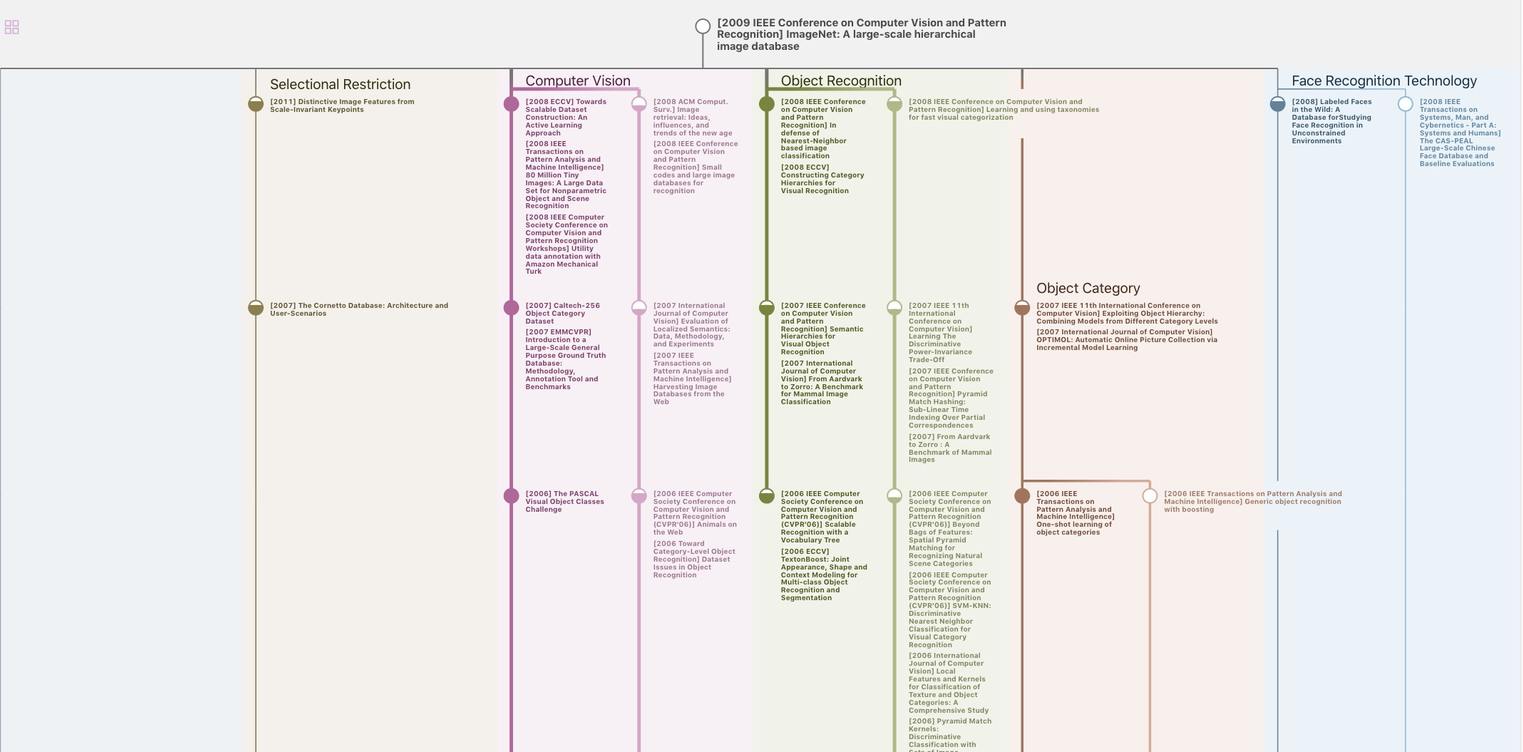
生成溯源树,研究论文发展脉络
Chat Paper
正在生成论文摘要