Robust Transfer Learning for Cross-domain Collaborative Filtering Using Multiple Rating Patterns Approximation.
WSDM 2018: The Eleventh ACM International Conference on Web Search and Data Mining Marina Del Rey CA USA February, 2018(2018)
摘要
Collaborative filtering techniques are a common approach for building recommendations, and have been widely applied in real recommender systems. However, collaborative filtering usually suffers from limited performance due to the sparsity of user-item interaction. To address this issue, auxiliary information is usually used to improve the performance. Transfer learning provides the key idea of using knowledge from auxiliary domains. An assumption of transfer learning in collaborative filtering is that the source domain is a full rating matrix, which may not hold in many real-world applications. In this paper, we investigate how to leverage rating patterns from multiple incomplete source domains to improve the quality of recommender systems. First, by exploiting the transferred learning, we compress the knowledge from the source domain into a cluster-level rating matrix. The rating patterns in the low-level matrix can be transferred to the target domain. Specifically, we design a knowledge extraction method to enrich rating patterns by relaxing the full rating restriction on the source domain. Finally, we propose a robust multiple-rating-pattern transfer learning model for cross-domain collaborative filtering, which is called MINDTL, to accurately predict missing values in the target domain. Extensive experiments on real-world datasets demonstrate that our proposed approach is effective and outperforms several alternative methods.
更多查看译文
AI 理解论文
溯源树
样例
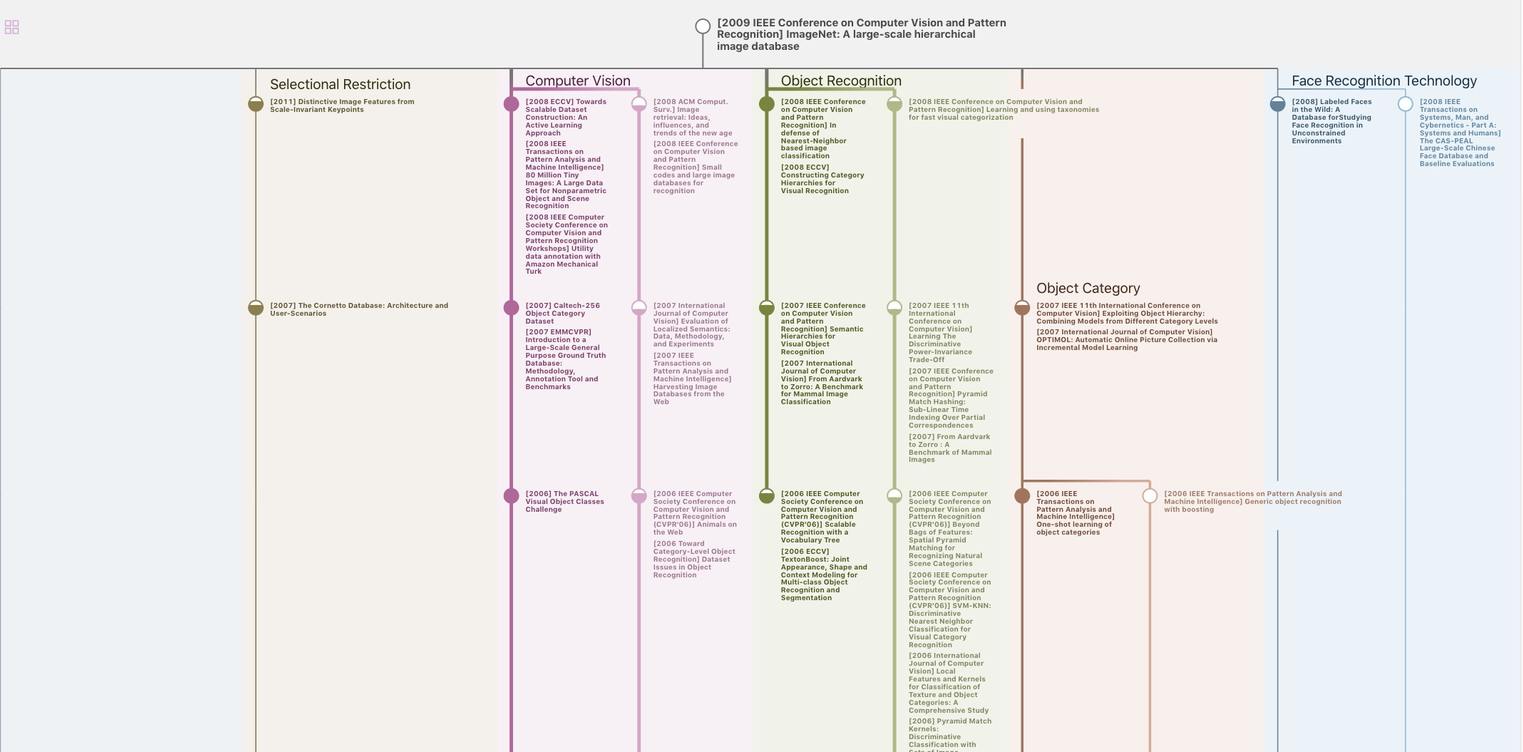
生成溯源树,研究论文发展脉络
Chat Paper
正在生成论文摘要