Linear Modelling of Cerebral Autoregulation System Using Genetic Algorithms.
CIARP(2017)
摘要
Cerebral autoregulation (CA) represents the brain’s capacity to maintain the cerebral blood flow constant, independent of the activities realized by an individual. There are pathologies like Alzheimer, vascular dementia, ischemic stroke, subarachnoid haemorrhage and severe brain injury, where a degradation of CA can be found. Despite limited understanding of its physiological basis, assessment of CA is relevant for diagnosis, monitoring and treating some of these pathologies. CA modelling is done by using mean arterial blood pressure (MABP) as input and cerebral blood flow velocity (CBFV) as output; the standard model used is transfer function analysis, although CA has been modelled with support vector machines (SVM) and other methods. In this work a resistive-capacitive model (R-C) is presented where parameters can be estimated from MABP and CBFV signals through Genetic Algorithms (GA), comparing its discrimination capacity against SVM models. Signals from 16 healthy subjects were used with 5 min of spontaneous variations (SV) and 5 min breathing oxygen with 5% of CO2 (hypercapnia). Results show that both models can capture CA and the degradation induced by hypercapnia. Using the autoregulation index (ARI), the R-C model discriminates with a ROC area of 0.89 against 0.72 from SVM, thus representing a promising alternative to assess CA.
更多查看译文
AI 理解论文
溯源树
样例
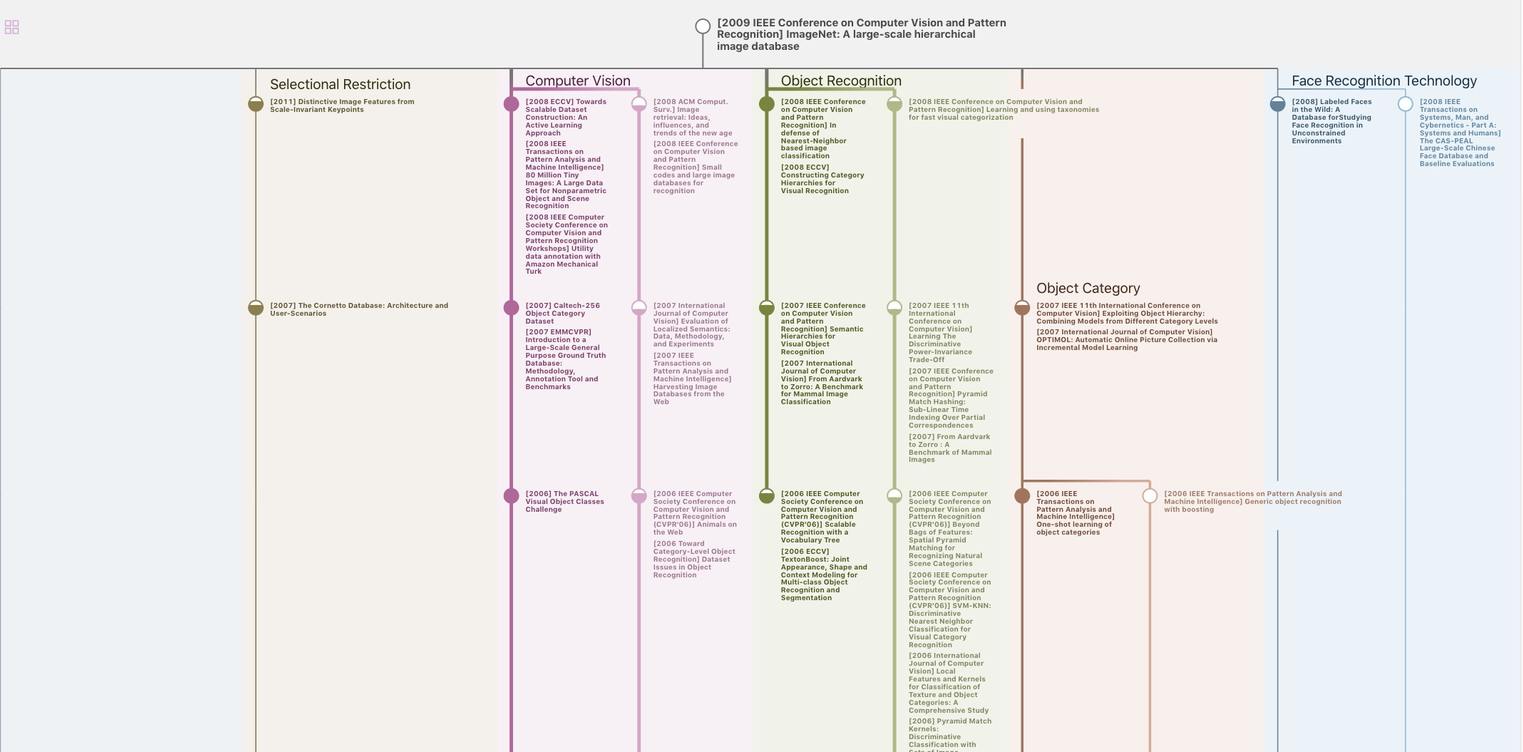
生成溯源树,研究论文发展脉络
Chat Paper
正在生成论文摘要