Pattern Identification For State Prediction In Dynamic Data Streams
2017 IEEE INTERNATIONAL CONFERENCE ON INTERNET OF THINGS (ITHINGS) AND IEEE GREEN COMPUTING AND COMMUNICATIONS (GREENCOM) AND IEEE CYBER, PHYSICAL AND SOCIAL COMPUTING (CPSCOM) AND IEEE SMART DATA (SMARTDATA)(2017)
摘要
This work proposes a pattern identification and online prediction algorithm for processing Internet of Things (IoT) time-series data. This is achieved by first proposing a new data aggregation and data driven discretisation method that does not require data segment normalisation. We apply a dictionary based algorithm in order to identify patterns of interest along with prediction of the next pattern. The performance of the proposed method is evaluated using synthetic and real-world datasets. The evaluations results shows that our system is able to identify the patterns by up to 85% accuracy which is 16.5% higher than a baseline using the Symbolic Aggregation Approximation (SAX) method.
更多查看译文
关键词
pattern identification,state prediction,dynamic data streams,online prediction algorithm,data aggregation,discretisation method,data segment normalisation,dictionary based algorithm,Internet of Things,IoT time-series data,symbolic aggregation approximation method,SAX method
AI 理解论文
溯源树
样例
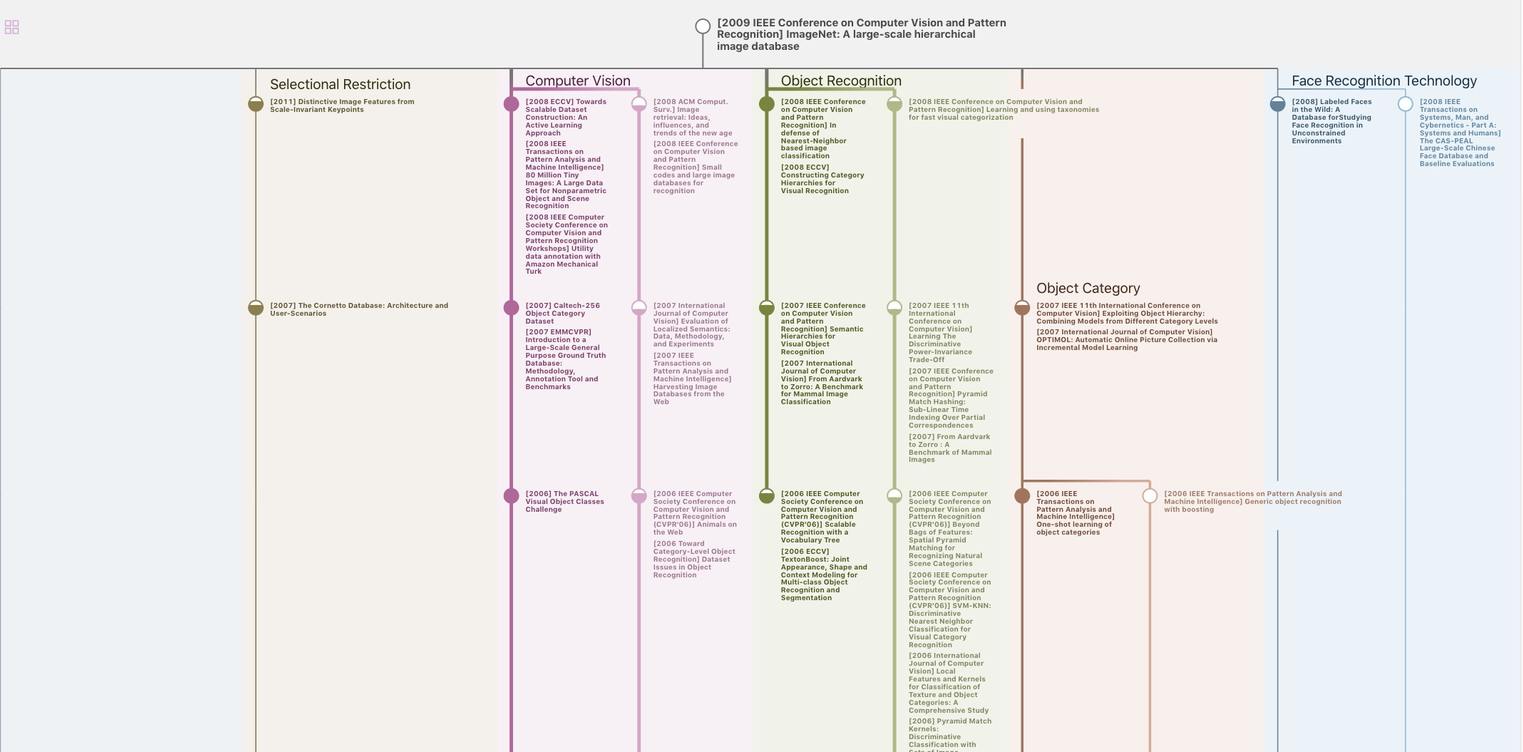
生成溯源树,研究论文发展脉络
Chat Paper
正在生成论文摘要