Information Geometry Of The Family Of Markov Kernels Defined By A Context Tree
2017 IEEE INFORMATION THEORY WORKSHOP (ITW)(2017)
摘要
We prove that a tree model is an exponential family (e-family) of Markov kernels, if and only if it is an FSMX model. The notion of e-family of Markov kernels was first introduced by Nakagawa and Kanaya ('93) in the one-dimensional case. Then, Nagaoka ('05) gave its established form, and Hayashi & Watanabe ('16) discussed it. A tree model is the Markov model defined by a context tree. It is noted by Weinberger et al., ('95) that tree models are classified into two classes; FSMX models and non-FSMX models, depending on the shape of their context trees. The FSMX model is a tree model and a finite state machine. We further show that, for Markov models, the e-family of Markov kernels is equivalent to the asymptotic e-family, which was introduced by Takeuchi & Barron ('98). Note that Takeuchi & Kawabata ('07) proved that non-FSMX tree models are not asymptotic e-families for the binary alphabet case. This paper enhances their result and reveals the information geometrical properties of tree models.
更多查看译文
关键词
information geometry,exponential family,finite state machine,asymptotic e-family,nonFSMX models,Markov model,FSMX model,tree model,context tree,Markov kernels
AI 理解论文
溯源树
样例
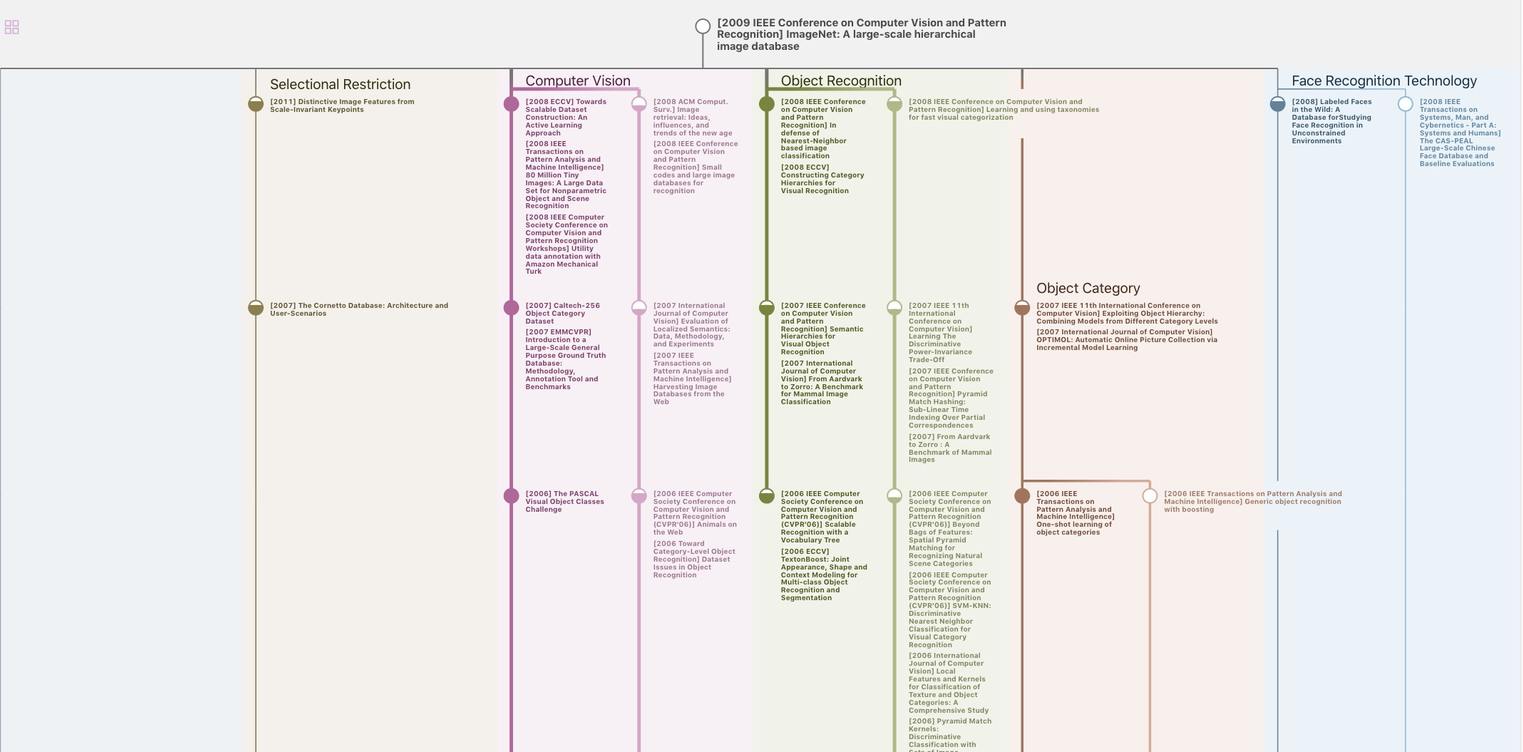
生成溯源树,研究论文发展脉络
Chat Paper
正在生成论文摘要