Filtering mislabeled data for improving time series classification
2017 9th International Workshop on the Analysis of Multitemporal Remote Sensing Images (MultiTemp)(2017)
摘要
The supervised classification of optical image time series allow the production of accurate land cover maps over large areas. However, the precision yielded by learning algorithms strongly depends on the quality of the reference data. The reference databases covering a large geographical area usually contain noisy data with an important number of mislabeled instances. These labeling errors result in longer training time, less accurate classifiers, and ultimately poorer results. To address this issue, we proposed a new iterative learning framework that removes mislabeled data from the training set. Specifically, a preliminary outlier rejection procedure based on the well-known Random Forest algorithm is proposed. The presented strategy is tested with the classification of Sentinel-2 image time series acquired on 2016 by using an out-of-date reference dataset collected on 2014.
更多查看译文
关键词
filtering mislabeled data,improving time series classification,accurate land cover maps,geographical area,random forest algorithm
AI 理解论文
溯源树
样例
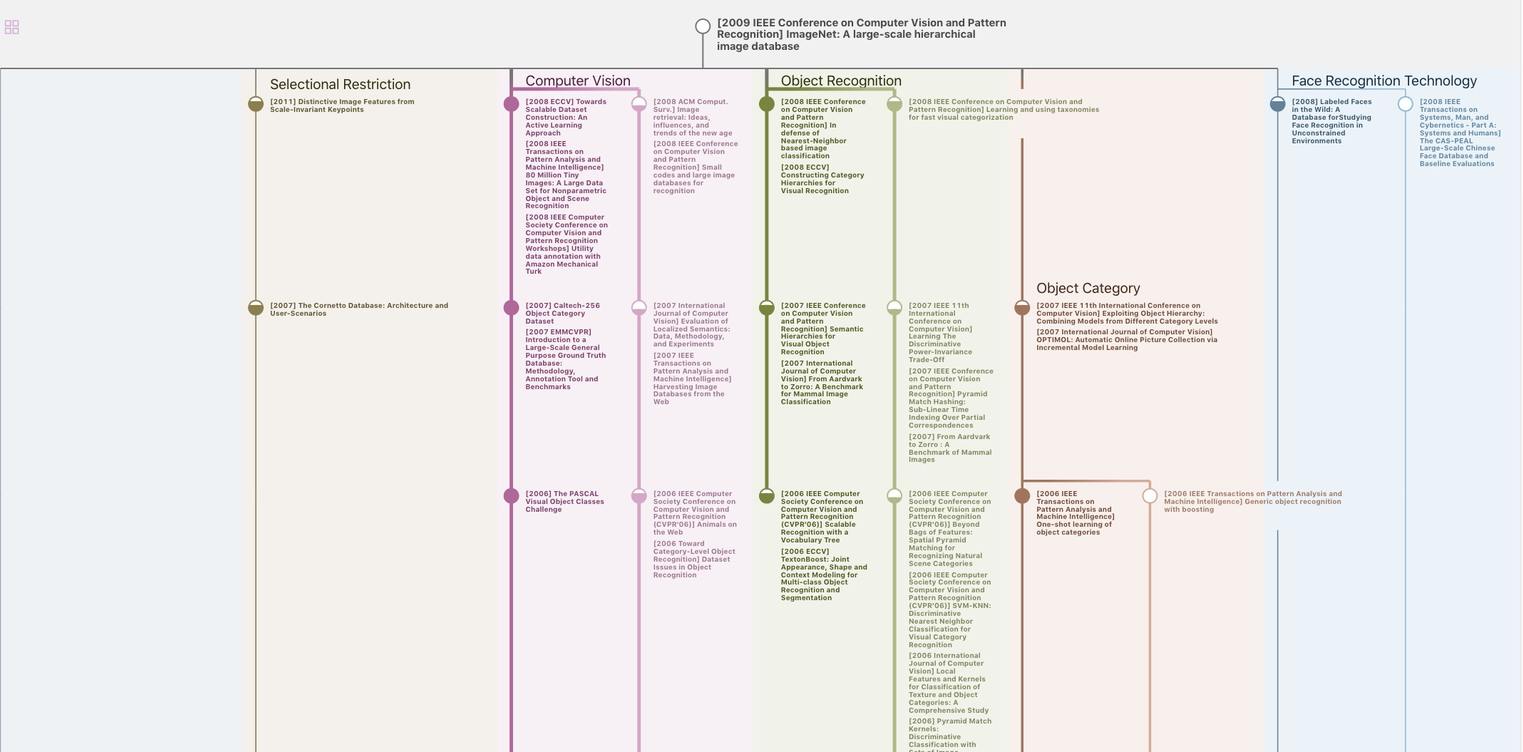
生成溯源树,研究论文发展脉络
Chat Paper
正在生成论文摘要