HAST-IDS: Learning Hierarchical Spatial-Temporal Features Using Deep Neural Networks to Improve Intrusion Detection.
IEEE ACCESS(2018)
摘要
The development of an anomaly-based intrusion detection system (IDS) is a primary research direction in the field of intrusion detection. An IDS learns normal and anomalous behavior by analyzing network traffic and can detect unknown and new attacks. However, the performance of an IDS is highly dependent on feature design, and designing a feature set that can accurately characterize network traffic is still an ongoing research issue. Anomaly-based IDSs also have the problem of a high false alarm rate (FAR), which seriously restricts their practical applications. In this paper, we propose a novel IDS called the hierarchical spatial-temporal features-based intrusion detection system (HAST-IDS), which first learns the low-level spatial features of network traffic using deep convolutional neural networks (CNNs) and then learns high-level temporal features using long short-term memory networks. The entire process of feature learning is completed by the deep neural networks automatically; no feature engineering techniques are required. The automatically learned traffic features effectively reduce the FAR. The standard DARPA1998 and ISCX2012 data sets are used to evaluate the performance of the proposed system. The experimental results show that the HAST-IDS outperforms other published approaches in terms of accuracy, detection rate, and FAR, which successfully demonstrates its effectiveness in both feature learning and FAR reduction.
更多查看译文
关键词
Network intrusion detection,deep neural networks,representation learning
AI 理解论文
溯源树
样例
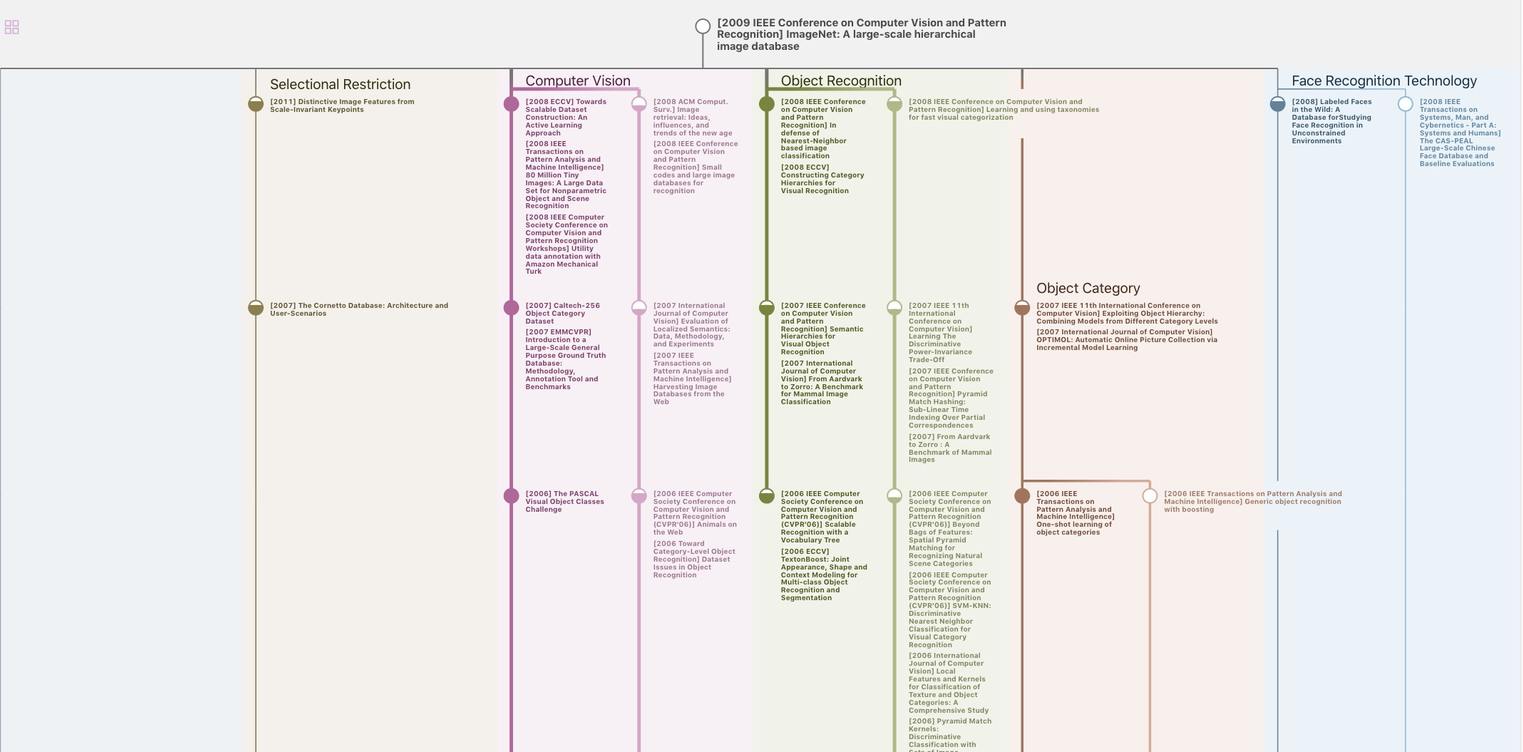
生成溯源树,研究论文发展脉络
Chat Paper
正在生成论文摘要