Approximating maxmin strategies in imperfect recall games using A-loss recall property.
International Journal of Approximate Reasoning(2018)
摘要
Extensive-form games with imperfect recall are an important model of dynamic games where the players are allowed to forget previously known information. Often, imperfect recall games result from an abstraction algorithm that simplifies a large game with perfect recall. Solving imperfect recall games is known to be a hard problem, and thus it is useful to search for a subclass of imperfect recall games which offers sufficient memory savings while being efficiently solvable. The abstraction process can then be guided to result in a game from this class. We focus on a subclass of imperfect recall games called A-loss recall games. First, we provide a complete picture of the complexity of solving imperfect recall and A-loss recall games. We show that the A-loss recall property allows us to compute a best response in polynomial time (computing a best response is NP-hard in imperfect recall games). This allows us to create a practical algorithm for approximating maxmin strategies in two-player games where the maximizing player has imperfect recall and the minimizing player has A-loss recall. This algorithm is capable of solving some games with up to 5⋅109 states in approximately 1 hour. Finally, we demonstrate that the use of imperfect recall abstraction can reduce the size of the strategy representation to as low as 0.03% of the size of the strategy representation in the original perfect recall game without sacrificing the quality of the maxmin strategy obtained by solving this abstraction.
更多查看译文
关键词
Imperfect recall,Abstraction,Maxmin strategy,A-loss recall
AI 理解论文
溯源树
样例
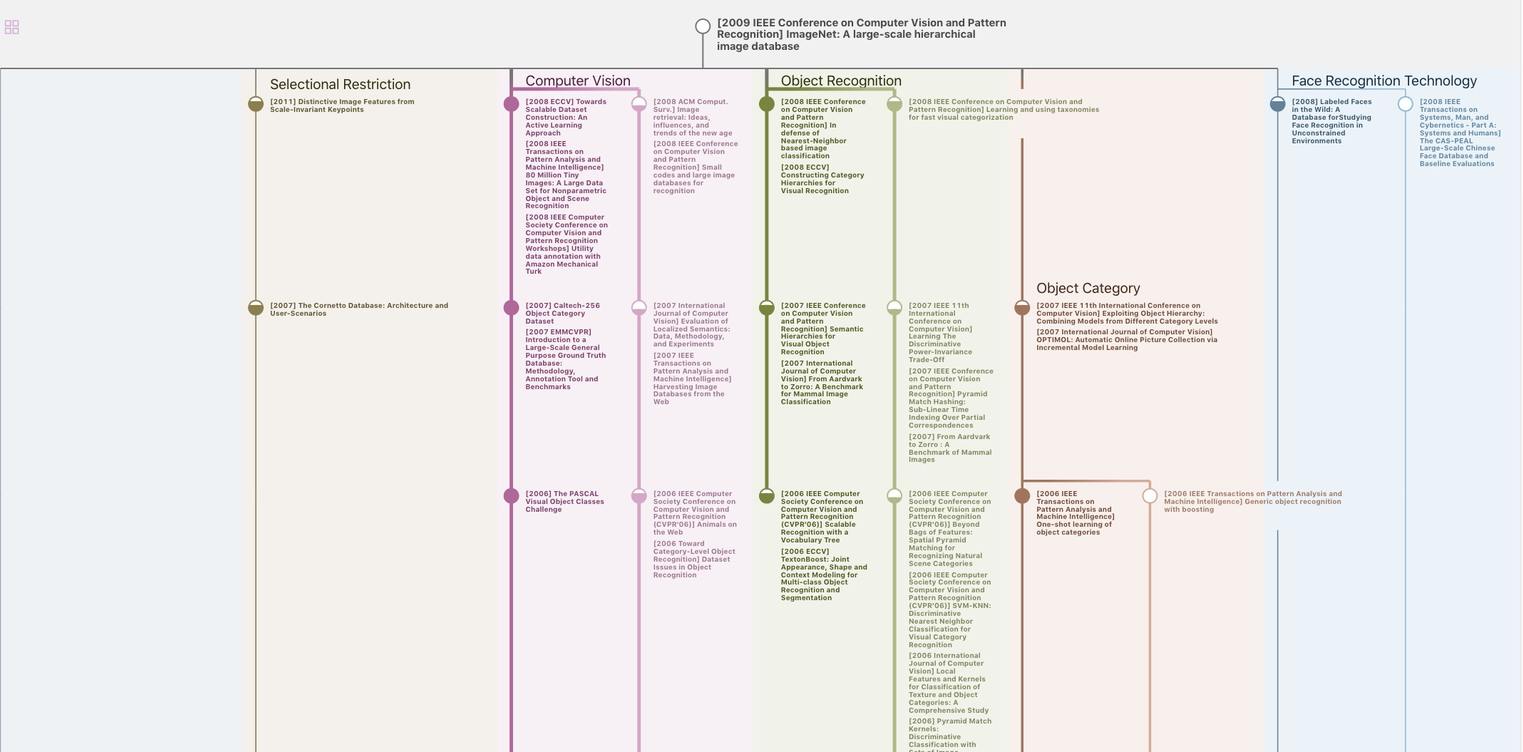
生成溯源树,研究论文发展脉络
Chat Paper
正在生成论文摘要