A Deterministic Protocol for Sequential Asymptotic Learning
2018 IEEE INTERNATIONAL SYMPOSIUM ON INFORMATION THEORY (ISIT)(2018)
摘要
In the classic herding model, agents receive private signals about an underlying binary state of nature, and act sequentially to choose one of two possible actions, after observing the actions of their predecessors. We investigate what types of behaviors lead to asymptotic learning, where agents will eventually converge to the right action in probability. It is known that for rational agents and bounded signals, there will not be asymptotic learning. Does it help if the agents can be cooperative rather than act selfishly? This is simple to achieve if the agents are allowed to use randomized protocols. In this paper, we provide the first deterministic protocol under which asymptotic learning occurs. In addition, our protocol has the advantage of being much simpler than previous protocols.
更多查看译文
关键词
probability,sequential asymptotic learning,deterministic protocol,randomized protocols,bounded signals,rational agents,private signals,classic herding model
AI 理解论文
溯源树
样例
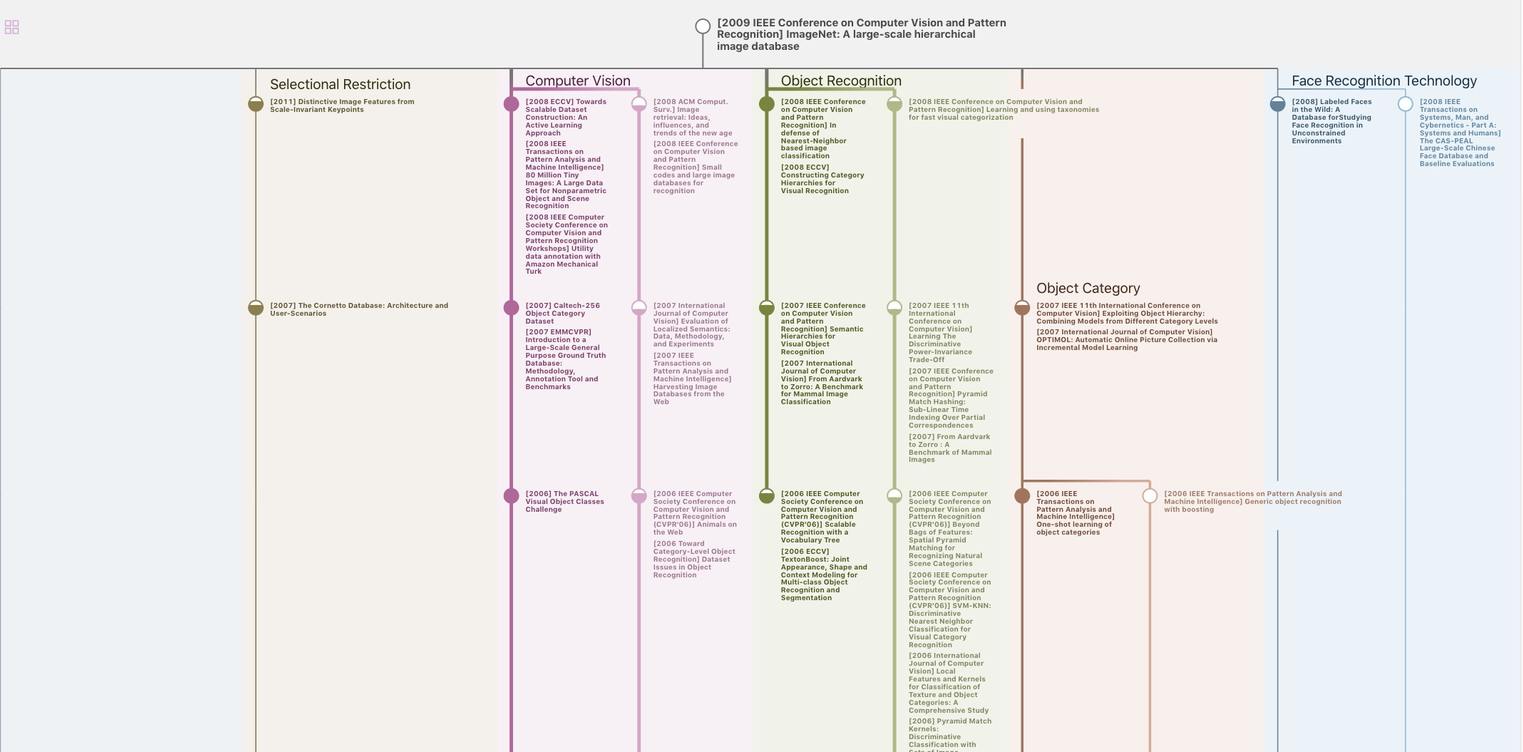
生成溯源树,研究论文发展脉络
Chat Paper
正在生成论文摘要