Expectation Propagation for Approximate Inference: Free Probability Framework
2018 IEEE International Symposium on Information Theory (ISIT)(2018)
Abstract
We study asymptotic properties of expectation propagation (EP) – a method for approximate inference originally developed in the field of machine learning. Applied to generalized linear models, EP iteratively computes a multivariate Gaussian approximation to the exact posterior distribution. The computational complexity of the repeated update of covariance matrices severely limits the application of EP to large problem sizes. In this study, we present a rigorous analysis by means of free probability theory that allows us to overcome this computational bottleneck if specific data matrices in the problem fulfill certain properties of asymptotic freeness. We demonstrate the relevance of our approach on the gene selection problem of a microarray dataset.
MoreTranslated text
Key words
asymptotic properties,posterior distribution,gene selection problem,microarray dataset,covariance matrices,multivariate Gaussian approximation,generalized linear models,machine learning,free probability framework,approximate inference,expectation propagation,free probability theory
AI Read Science
Must-Reading Tree
Example
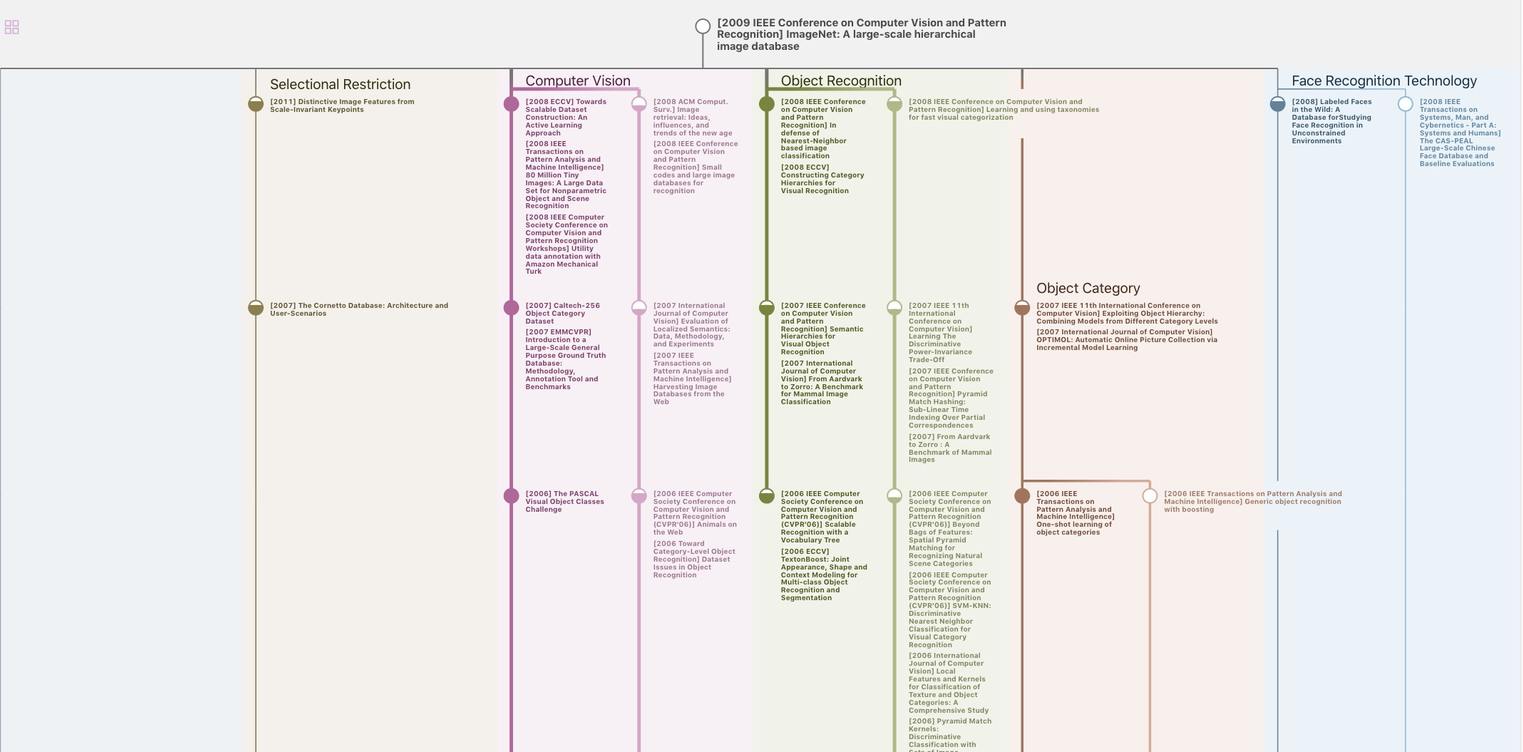
Generate MRT to find the research sequence of this paper
Chat Paper
Summary is being generated by the instructions you defined