Latent RANSAC
Computer Vision and Pattern Recognition(2018)
摘要
We present a method that can evaluate a RANSAC hypothesis in constant time, i.e. independent of the size of the data. A key observation here is that correct hypotheses are tightly clustered together in the latent parameter domain. In a manner similar to the generalized Hough transform we seek to find this cluster, only that we need as few as two votes for a successful detection. Rapidly locating such pairs of similar hypotheses is made possible by adapting the recent "Random Grids" range-search technique. We only perform the usual (costly) hypothesis verification stage upon the discovery of a close pair of hypotheses. We show that this event rarely happens for incorrect hypotheses, enabling a significant speedup of the RANSAC pipeline. The suggested approach is applied and tested on three robust estimation problems: camera localization, 3D rigid alignment and 2D-homography estimation. We perform rigorous testing on both synthetic and real datasets, demonstrating an improvement in efficiency without a compromise in accuracy. Furthermore, we achieve state-of-the-art 3D alignment results on the challenging "Redwood" loop-closure challenge.
更多查看译文
关键词
latent RANSAC,RANSAC hypothesis,constant time,latent parameter domain,successful detection,similar hypotheses,incorrect hypotheses,RANSAC pipeline,robust estimation problems,camera localization,3D rigid alignment,2D-homography estimation,rigorous testing,state-of-the-art 3D alignment results,generalized Hough transform,random grids range-search technique,hypothesis verification stage
AI 理解论文
溯源树
样例
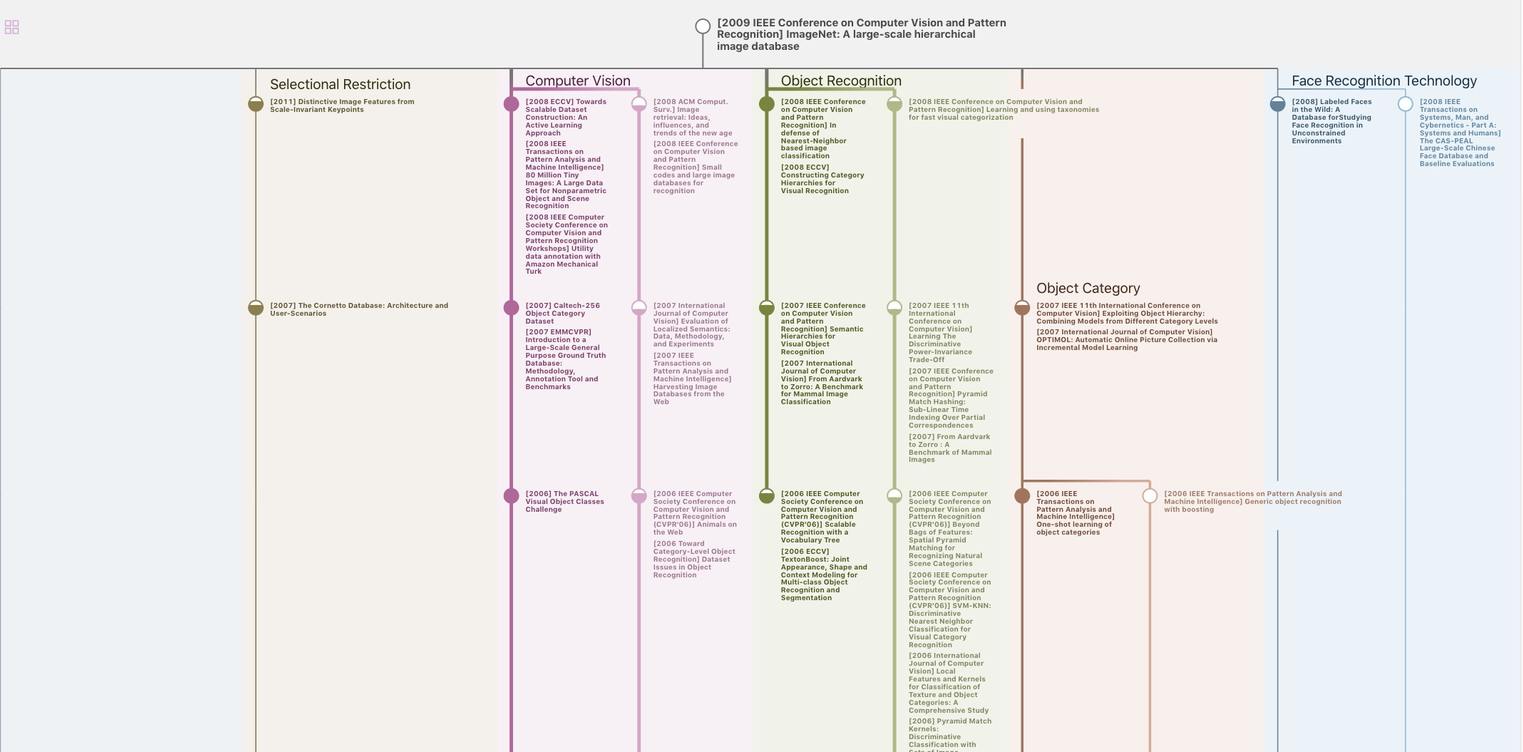
生成溯源树,研究论文发展脉络
Chat Paper
正在生成论文摘要