Accelerating recurrent neural network language model based online speech recognition system
2018 IEEE International Conference on Acoustics, Speech and Signal Processing (ICASSP)(2018)
摘要
This paper presents methods to accelerate recurrent neural network based language models (RNNLMs) for online speech recognition systems. Firstly, a lossy compression of the past hidden layer outputs (history vector) with caching is introduced in order to reduce the number of LM queries. Next, RNNLM computations are deployed in a CPU-GPU hybrid manner, which computes each layer of the model on a more advantageous platform. The added overhead by data exchanges between CPU and GPU is compensated through a frame-wise batching strategy. The performance of the proposed methods evaluated on LibriSpeech test sets indicates that the reduction in history vector precision improves the average recognition speed by 1.23 times with minimum degradation in accuracy. On the other hand, the CPU-GPU hybrid parallelization enables RNNLM based real-time recognition with a four times improvement in speed.
更多查看译文
关键词
Online speech recognition,language model,recurrent neural network,graphic processing unit
AI 理解论文
溯源树
样例
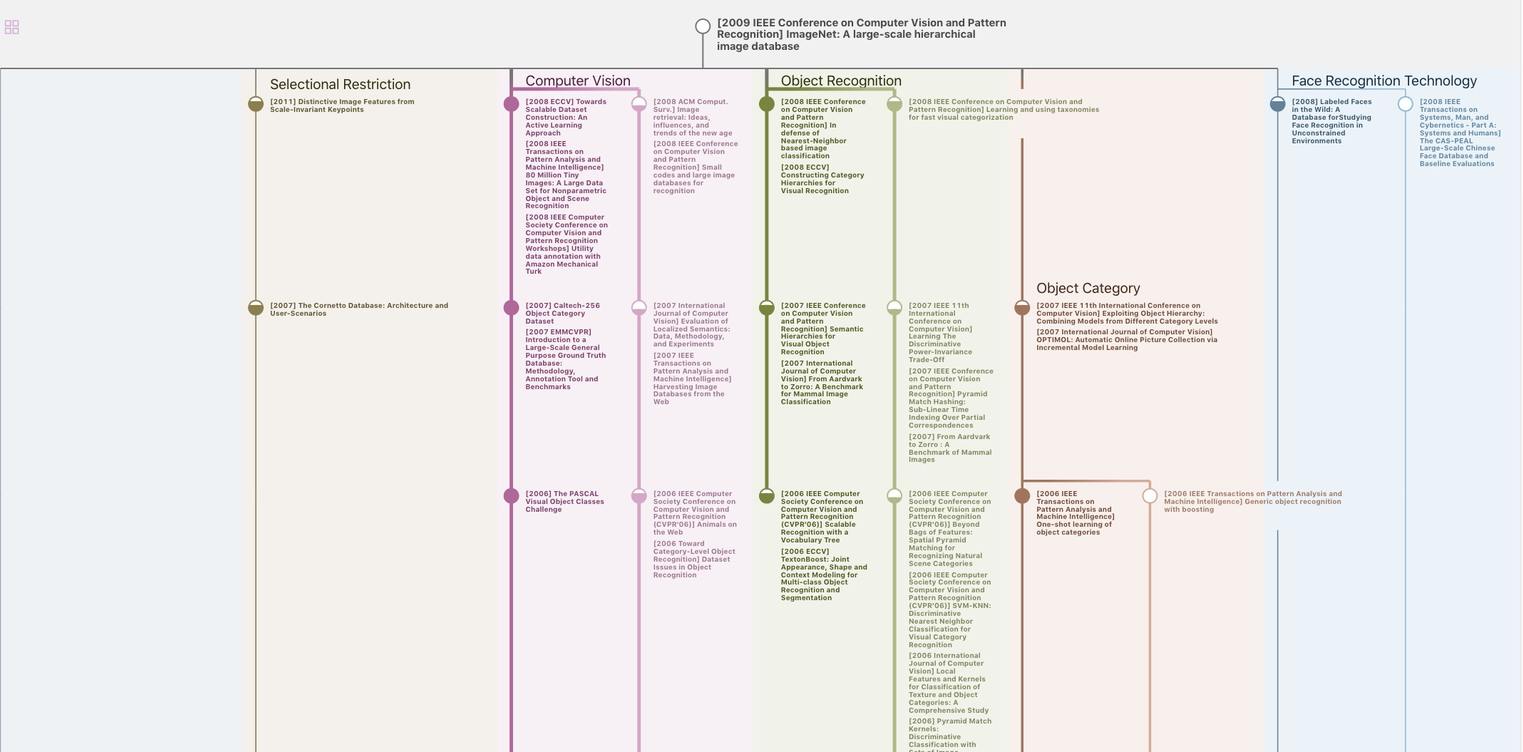
生成溯源树,研究论文发展脉络
Chat Paper
正在生成论文摘要