Predicting Topics in Scholarly Papers.
ADVANCES IN INFORMATION RETRIEVAL (ECIR 2018)(2018)
摘要
In the last few decades, topic models have been extensively used to discover the latent topical structure of large text corpora; however, very little has been done to model the continuation of such topics in the near future. In this paper we present a novel approach for tracking topical changes over time and predicting the topics which would continue in the near future. For our experiments, we used a publicly available corpus of conference papers, since scholarly papers lead the technological advancements and represent an important source of information that can be used to make decisions regarding the funding strategies in the scientific community. The experimental results show that our model outperforms two major baselines for dynamic topic modeling in terms of predictive power.
更多查看译文
关键词
Topic prediction,Topic modeling,Temporal evolution of topics
AI 理解论文
溯源树
样例
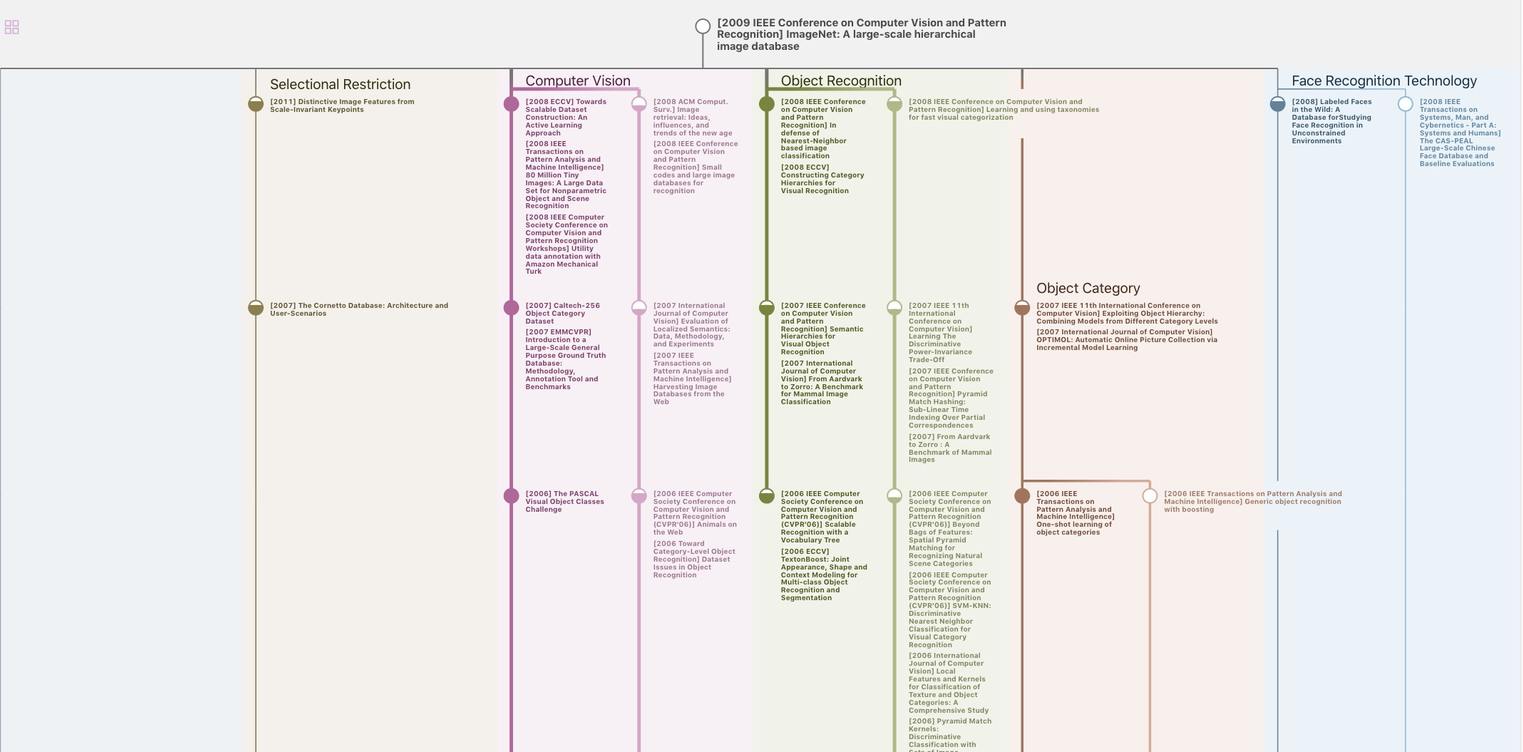
生成溯源树,研究论文发展脉络
Chat Paper
正在生成论文摘要