Hierarchical Learning For Salient Object Detection
2017 INTERNATIONAL CONFERENCE ON SECURITY, PATTERN ANALYSIS, AND CYBERNETICS (SPAC)(2017)
摘要
Most existing methods for salient object detection either depend on simple feature, such as contrast or boundary prior, which is sensitive to background variety, or extract redundant features for robustness, which is time-consuming. In this paper, we propose a hierarchical learning structure to alleviate the demanding feature. The hierarchical learning is based on low rank (LR) decomposition and broad learning system (BLS). LR model with Laplacian constraint is applied to roughly separate foreground from background, which produces several positive and negative super-pixels as example to train a BLS classifier. The classifier is used to determine the final saliency of each super-pixel. Experiments on two public datasets including MSRA10K and ECSSD show that our method achieves state-of-the-art result compared with the other nine methods.
更多查看译文
关键词
salient object detection,redundant features,hierarchical learning structure,broad learning system,LR model,BLS classifier,low-rank decomposition
AI 理解论文
溯源树
样例
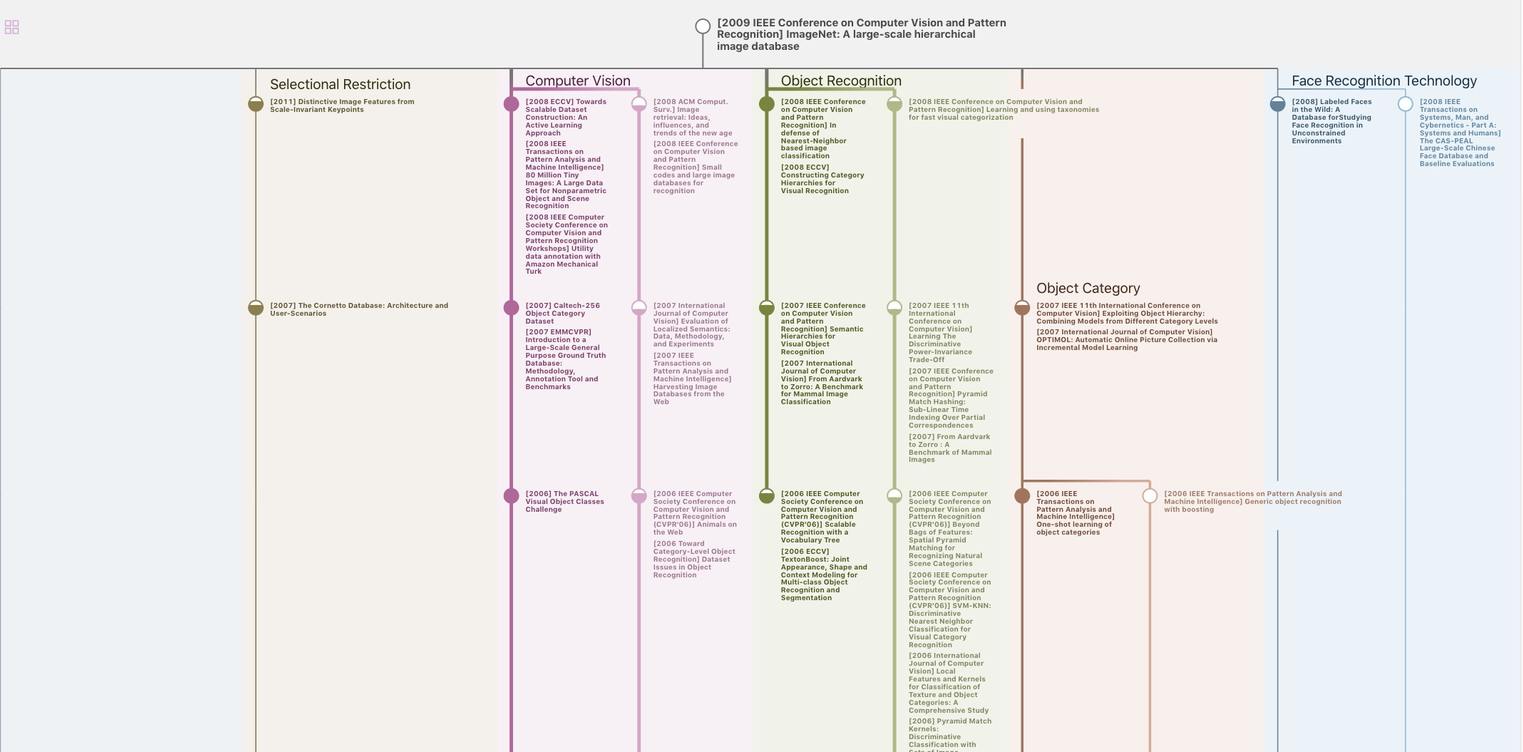
生成溯源树,研究论文发展脉络
Chat Paper
正在生成论文摘要