AnchorViz: Facilitating Classifier Error Discovery through Interactive Semantic Data Exploration.
IUI(2018)
摘要
When building a classifier in interactive machine learning, human knowledge about the target class can be a powerful reference to make the classifier robust to unseen items. The main challenge lies in finding unlabeled items that can either help discover or refine concepts for which the current classifier has no corresponding features (i.e., it has feature blindness). Yet it is unrealistic to ask humans to come up with an exhaustive list of items, especially for rare concepts that are hard to recall. This paper presents AnchorViz, an interactive visualization that facilitates error discovery through semantic data exploration. By creating example-based anchors, users create a topology to spread data based on their similarity to the anchors and examine the inconsistencies between data points that are semantically related. The results from our user study show that AnchorViz helps users discover more prediction errors than stratified random and uncertainty sampling methods.
更多查看译文
关键词
interactive machine learning, visualization, error discovery, semantic data exploration, unlabeled data
AI 理解论文
溯源树
样例
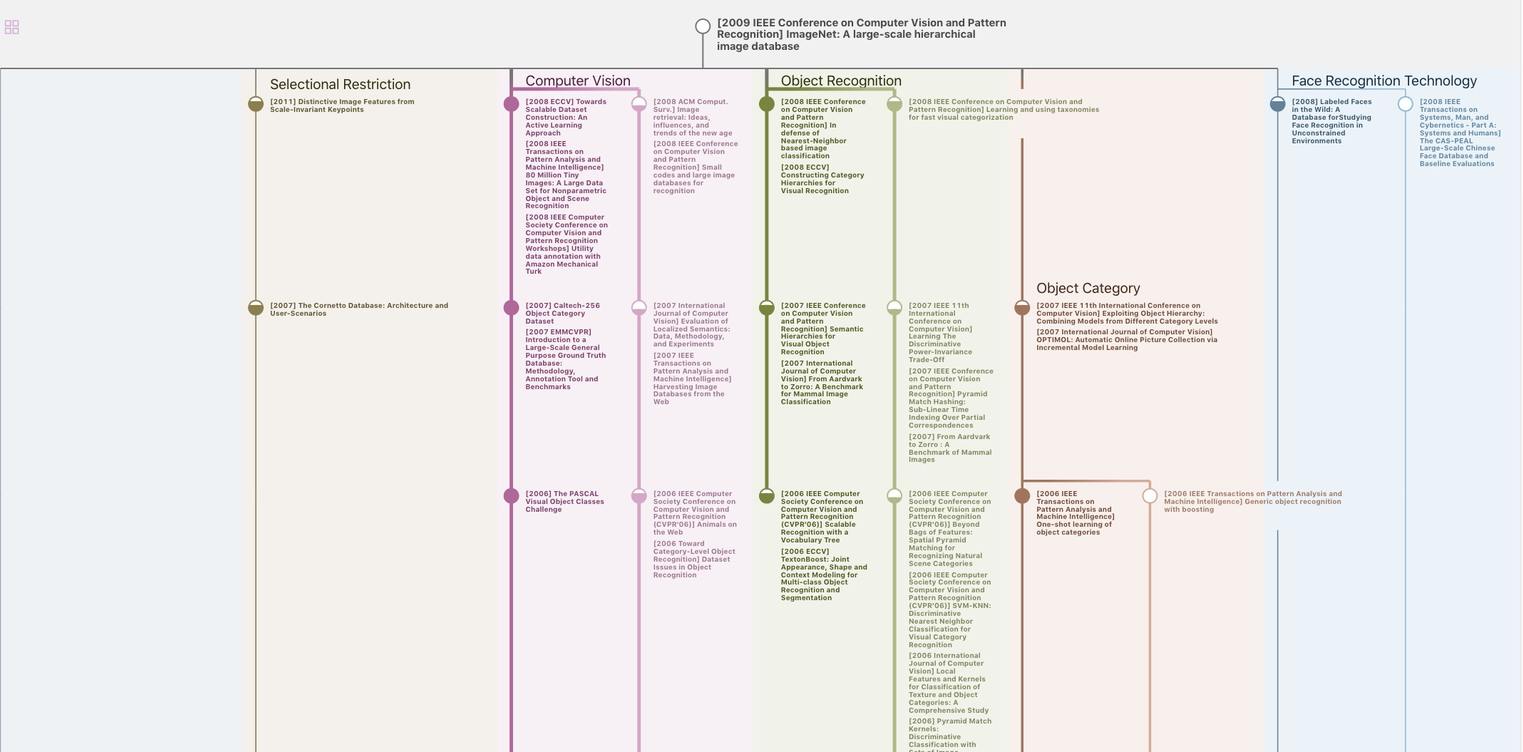
生成溯源树,研究论文发展脉络
Chat Paper
正在生成论文摘要