Non-Intrusive Multi-Modal Estimation of Building Occupancy
SenSys(2017)
摘要
Estimation of building occupancy has emerged as an important research problem with applications ranging from building energy efficiency, control and automation, safety, communication network resource allocation, etc. In this research work, we propose the estimation of occupancy using non-intrusive information that is already available from existing sensing modes, namely, number of WiFi devices, electrical energy demand and water consumption rate. Using data collected from 76 buildings in a university campus, we study the feasibility of multi-modal fusion between the three data sources for estimating fine-grained occupancy. In order to make the estimation model scalable, we propose three different clustering schemes to identify similarity in building characteristics and training per-cluster occupancy estimation models. The presented multi-modal fusion estimation framework achieves a mean absolute percentage error of 13.22% and we find that leveraging all three modalities provide an improvement of 48% in accuracy as compared to WiFi-only occupancy estimation. Our evaluation also shows that clustering buildings greatly increases the scalability of the proposed approach through significant reduction in training overhead, while providing an accuracy comparable to exhaustive, per-building estimation models.
更多查看译文
关键词
Occupancy counting, Smart buildings, Multi-modal sensing
AI 理解论文
溯源树
样例
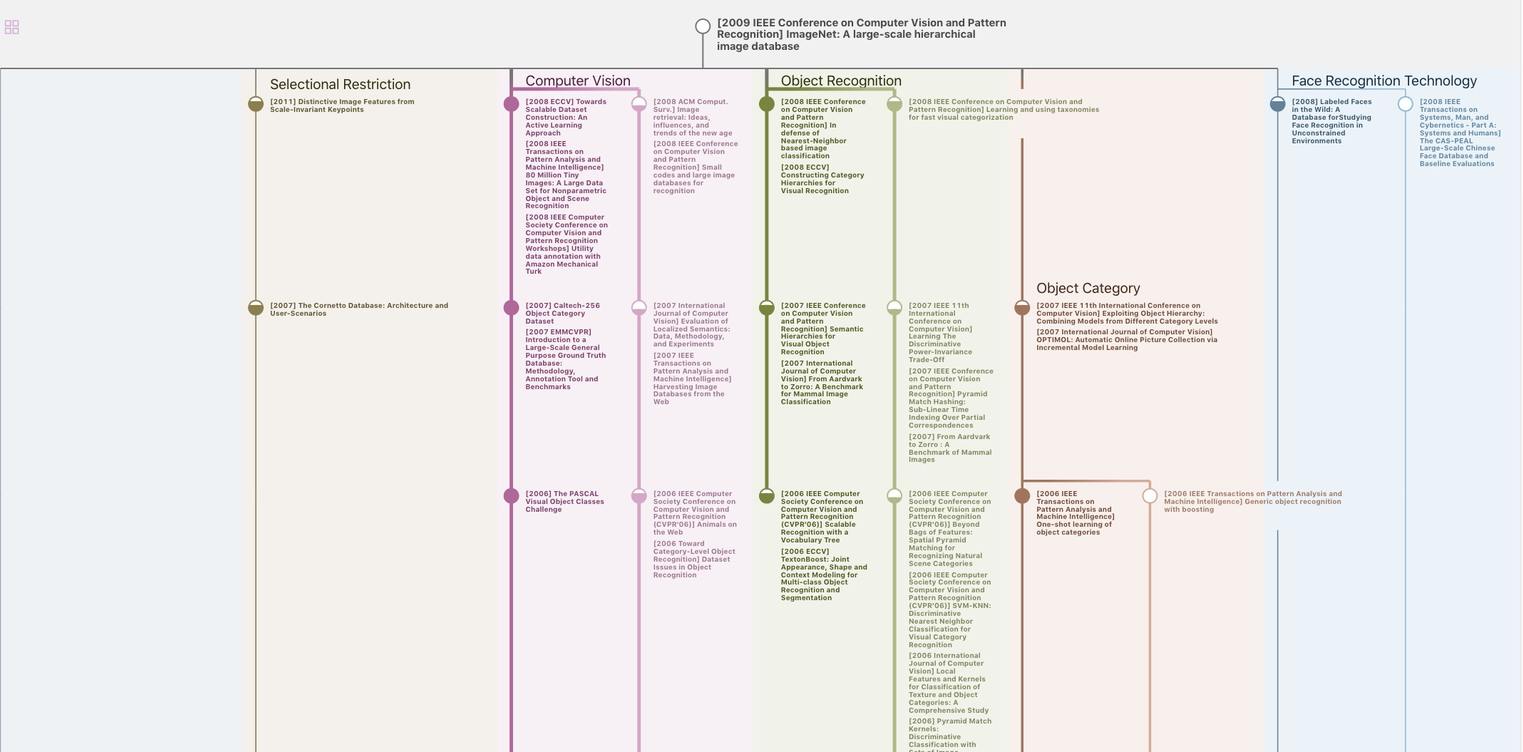
生成溯源树,研究论文发展脉络
Chat Paper
正在生成论文摘要