Automatic detection of kidney in 3D pediatric ultrasound images using deep neural networks.
Proceedings of SPIE(2018)
摘要
Ultrasound (US) imaging is the routine and safe diagnostic modality for detecting pediatric urology problems, such as hydronephrosis in the kidney. Hydronephrosis is the swelling of one or both kidneys because of the build-up of urine. Early detection of hydronephrosis can lead to a substantial improvement in kidney health outcomes. Generally, US imaging is a challenging modality for the evaluation of pediatric kidneys with different shape, size, and texture characteristics. The aim of this study is to present an automatic detection method to help kidney analysis in pediatric 3DUS images. The method localizes the kidney based on its minimum volume oriented bounding box using deep neural networks. Separate deep neural networks are trained to estimate the kidney position, orientation, and scale, making the method computationally efficient by avoiding full parameter training. The performance of the method was evaluated using a dataset of 45 kidneys (17 normal and 28 diseased kidneys diagnosed with hydronephrosis) through the leave-one out cross validation method. Quantitative results show the proposed detection method could extract the kidney position, orientation, and scale with root mean square values of 1.3 +/- 0.9 mm, 6.34 +/- 4.32 degrees, and 2.26 +/- 1.8 mm, respectively. This method could be helpful in automating kidney segmentation for routine clinical evaluation.
更多查看译文
关键词
Hydronephrosis,pediatric kidney,ultrasound imaging,localization,deep neural networks
AI 理解论文
溯源树
样例
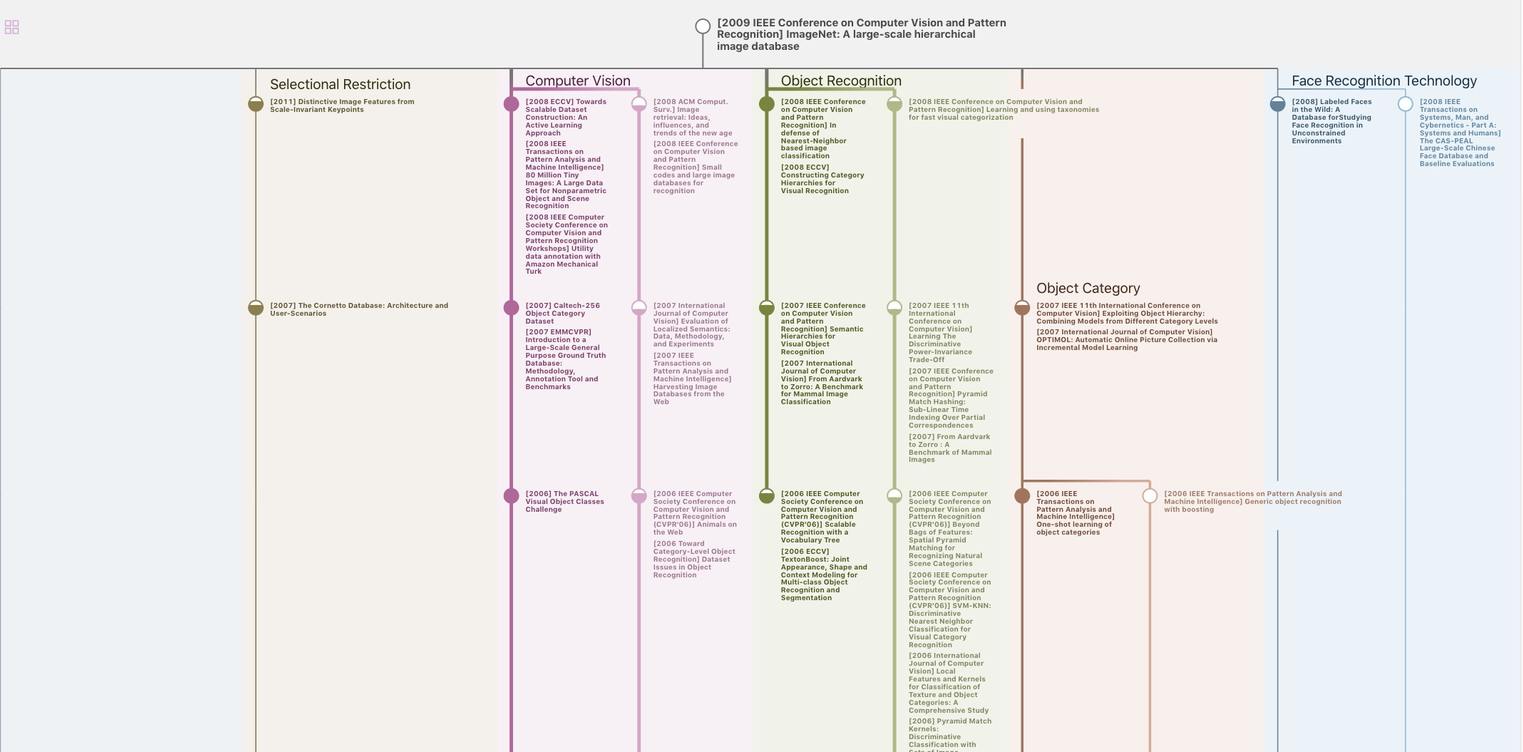
生成溯源树,研究论文发展脉络
Chat Paper
正在生成论文摘要