Action Recognition Using Low-Rank Sparse Representation
IEICE TRANSACTIONS ON INFORMATION AND SYSTEMS(2018)
摘要
Human action recognition in videos draws huge research interests in computer vision. The Bag-of-Word model is quite commonly used to obtain the video level representations, however, BoW model roughly assigns each feature vector to its nearest visual word and the collection of unordered words ignores the interest points' spatial information, inevitably causing nontrivial quantization errors and impairing improvements on classification rates. To address these drawbacks, we propose an approach for action recognition by encoding spatio-temporal log Euclidean covariance matrix (ST-LECM) features within the low-rank and sparse representation framework. Motivated by low rank matrix recovery, local descriptors in a spatial temporal neighborhood have similar representation and should be approximately low rank. The learned coefficients can not only capture the global data structures, but also preserve consistent. Experimental results showed that the proposed approach yields excellent recognition performance on synthetic video datasets and are robust to action variability, view variations and partial occlusion.
更多查看译文
关键词
human action recognition, low-rank sparse representation, bag of word model, sparse coding representation, low-rank representation
AI 理解论文
溯源树
样例
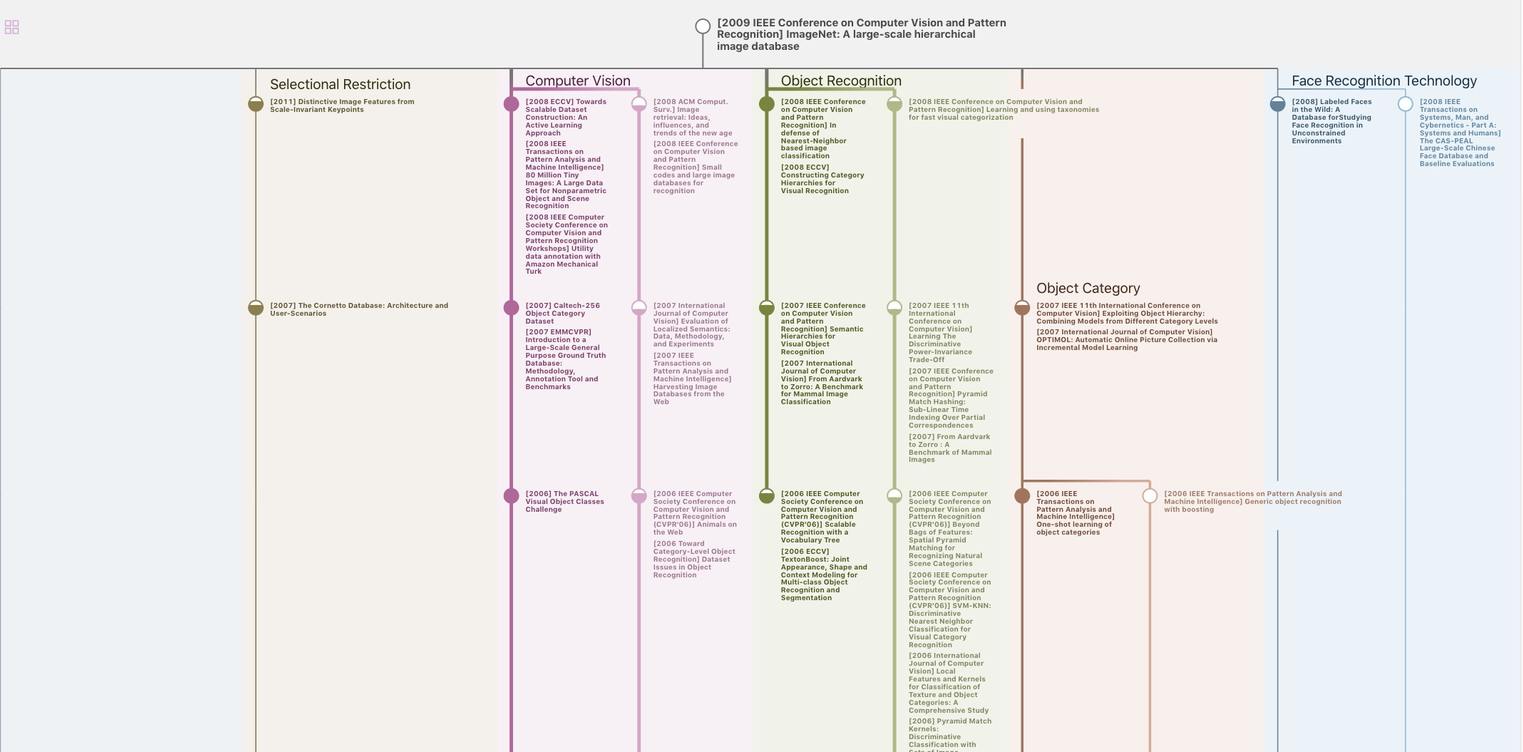
生成溯源树,研究论文发展脉络
Chat Paper
正在生成论文摘要