Online Adaptation and Energy Minimization for Hardware Recurrent Spiking Neural Networks
ACM Journal on Emerging Technologies in Computing Systems(2018)
摘要
The Liquid State Machine (LSM) is a promising model of recurrent spiking neural networks that provides an appealing brain-inspired computing paradigm for machine-learning applications such as pattern recognition. Moreover, processing information directly on spiking events makes the LSM well suited for cost- and energy-efficient hardware implementation. In this article, we systematically present three techniques for optimizing energy efficiency while maintaining good performance of the proposed LSM neural processors from both an algorithmic and hardware implementation point of view. First, to realize adaptive LSM neural processors, thus boost learning performance, we propose a hardware-friendly Spike-Timing Dependent Plastic (STDP) mechanism for on-chip tuning. Then, the LSM processor incorporates a novel runtime correlation-based neuron gating scheme to minimize the power dissipated by reservoir neurons. Furthermore, an activity-dependent clock gating approach is presented to address the energy inefficiency due to the memory-intensive nature of the proposed neural processors. Using two different real-world tasks of speech and image recognition to benchmark, we demonstrate that the proposed architecture boosts the average learning performance by up to 2.0% while reducing energy dissipation by up to 29% compared to a baseline LSM with little extra hardware overhead on a Xilinx Virtex-6 FPGA.
更多查看译文
关键词
Liquid state machine,online adaptation,spike-timing dependent plasticity,energy efficiency
AI 理解论文
溯源树
样例
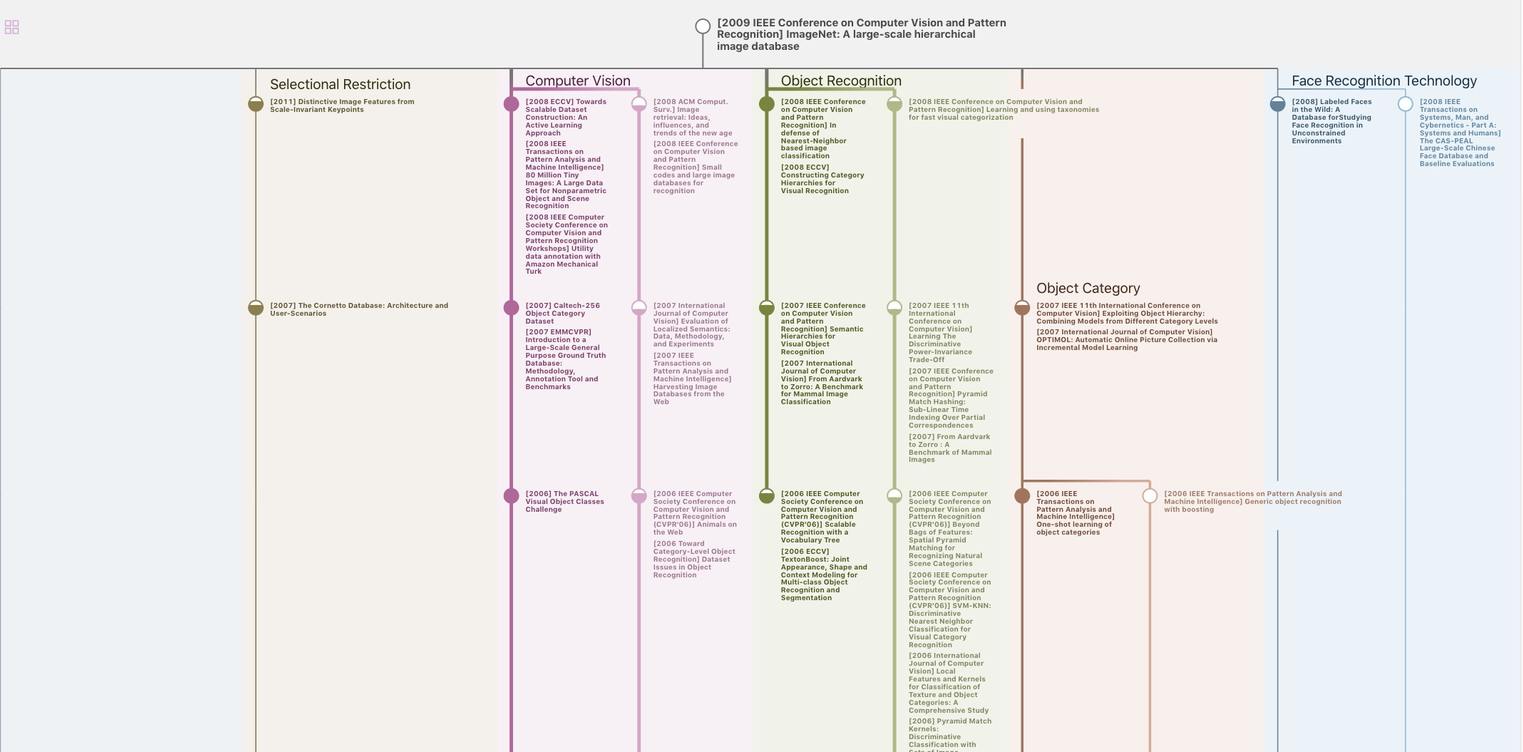
生成溯源树,研究论文发展脉络
Chat Paper
正在生成论文摘要