Geospatial Object Detection in High Resolution Satellite Images Based on Multi-Scale Convolutional Neural Network.
REMOTE SENSING(2018)
摘要
Daily acquisition of large amounts of aerial and satellite images has facilitated subsequent automatic interpretations of these images. One such interpretation is object detection. Despite the great progress made in this domain, the detection of multi-scale objects, especially small objects in high resolution satellite (HRS) images, has not been adequately explored. As a result, the detection performance turns out to be poor. To address this problem, we first propose a unified multi-scale convolutional neural network (CNN) for geospatial object detection in HRS images. It consists of a multi-scale object proposal network and a multi-scale object detection network, both of which share a multi-scale base network. The base network can produce feature maps with different receptive fields to be responsible for objects with different scales. Then, we use the multi-scale object proposal network to generate high quality object proposals from the feature maps. Finally, we use these object proposals with the multi-scale object detection network to train a good object detector. Comprehensive evaluations on a publicly available remote sensing object detection dataset and comparisons with several state-of-the-art approaches demonstrate the effectiveness of the presented method. The proposed method achieves the best mean average precision (mAP) value of 89.6%, runs at 10 frames per second (FPS) on a GTX 1080Ti GPU.
更多查看译文
关键词
high resolution satellite images,geospatial object detection,object proposal network,object detection network
AI 理解论文
溯源树
样例
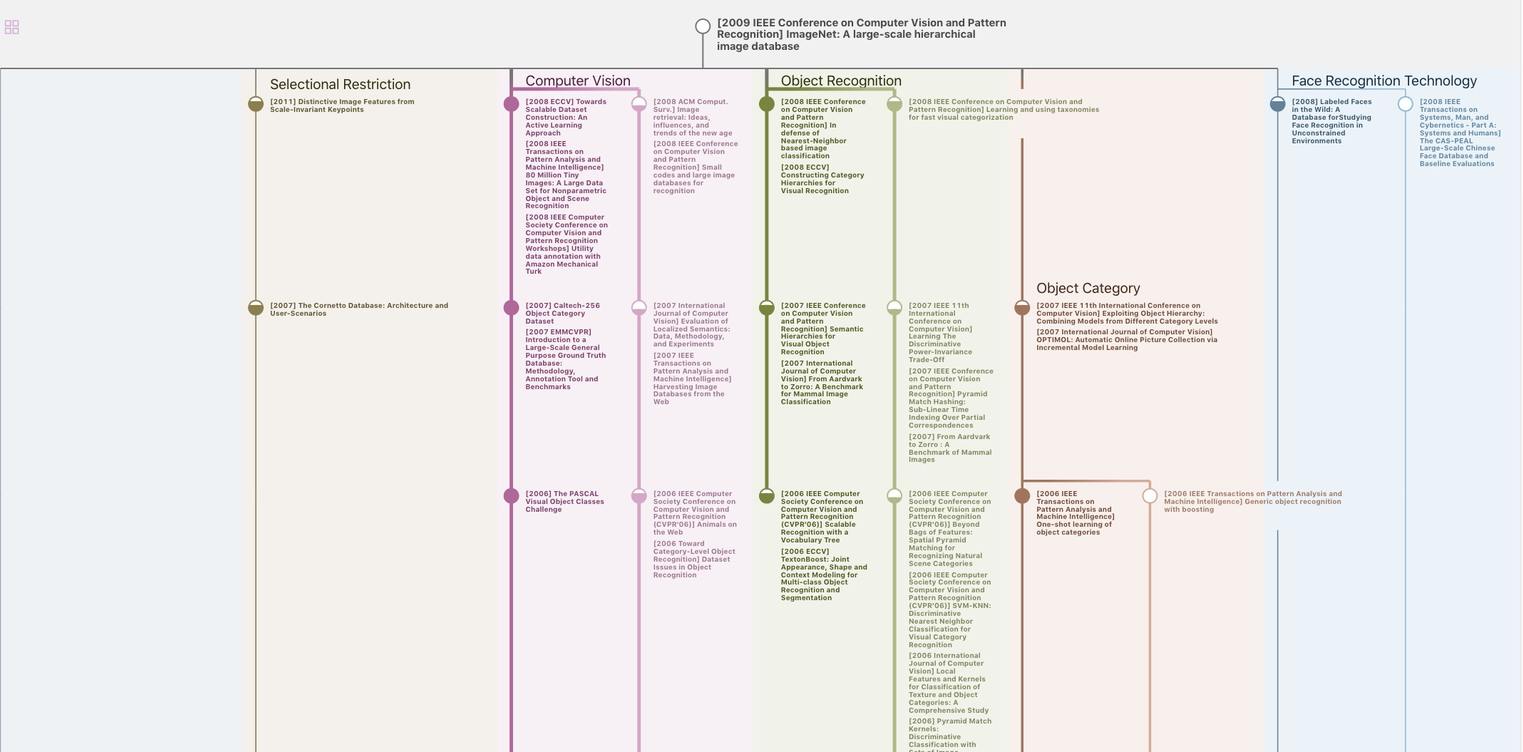
生成溯源树,研究论文发展脉络
Chat Paper
正在生成论文摘要