Hyperspectral Image Classification Using Convolutional Neural Networks and Multiple Feature Learning
Remote Sensing(2018)
摘要
Convolutional neural networks (CNNs) have been extended to hyperspectral imagery (HSI) classification due to its better feature representation and high performance, whereas multiple feature learning has shown its effectiveness in computer vision areas. This paper proposes a novel framework that takes advantage of both CNNs and multiple feature learning to better predict the class labels for HSI pixels. We built a novel CNN architecture with various features extracted from the raw imagery as input. The network generates the corresponding relevant feature maps for the input, and the generated feature maps are fed into a concatenating layer to form a joint feature map. The obtained joint feature map is then input to the subsequent layers to predict the final labels for each hyperspectral pixel. The proposed method not only takes advantage of enhanced feature extraction from CNNs, but also fully exploits the spectral and spatial information jointly. The effectiveness of the proposed method is tested with three benchmark data sets, and the results show that the CNN-based multi-feature learning framework improves the classification accuracy significantly.
更多查看译文
关键词
convolutional neural networks (CNNs),hyperspectral imagery (HSI),classification,multiple feature learning
AI 理解论文
溯源树
样例
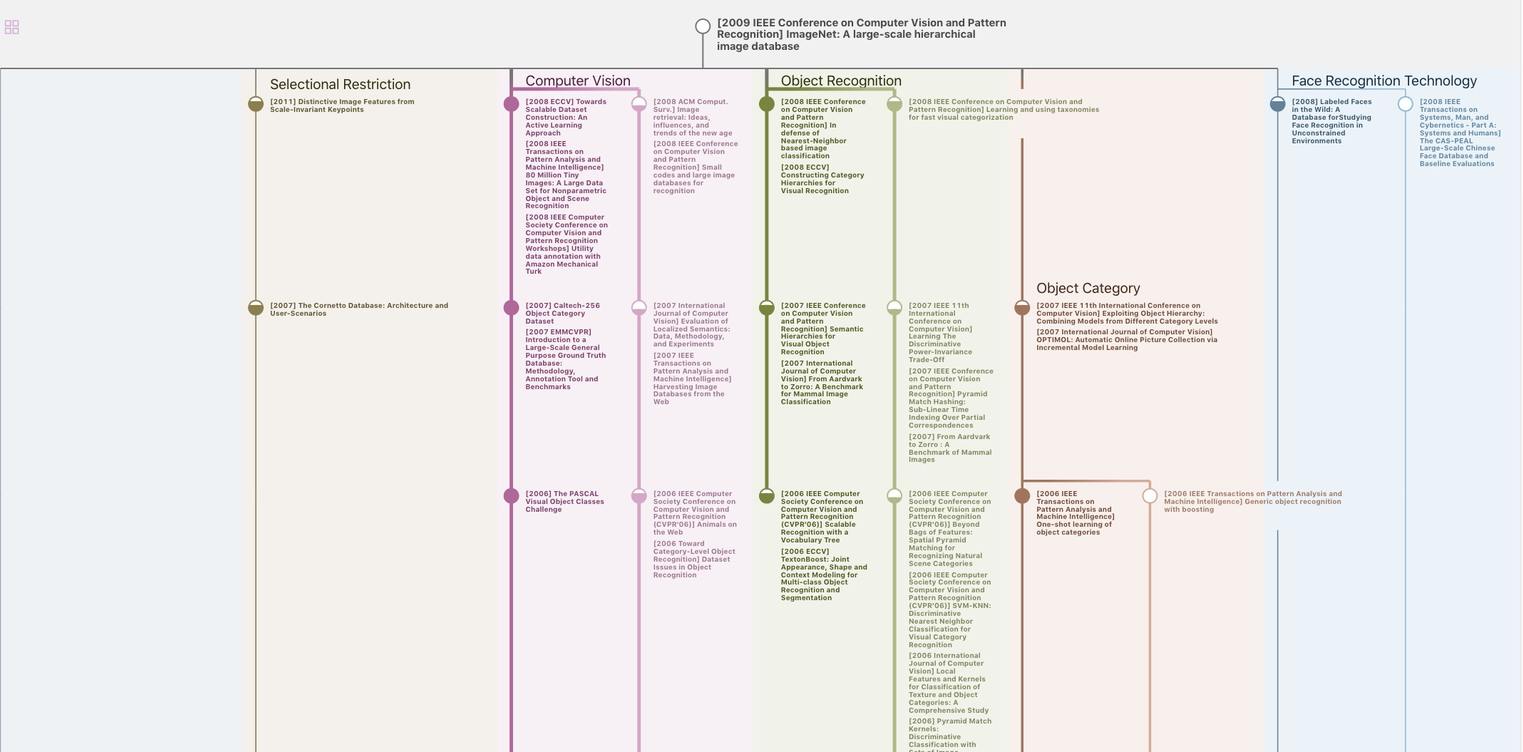
生成溯源树,研究论文发展脉络
Chat Paper
正在生成论文摘要