Direct Arrhythmia Classification from Compressive ECG Signals in Wearable Health Monitoring System.
JOURNAL OF CIRCUITS SYSTEMS AND COMPUTERS(2018)
摘要
Due to the capacity of processing signal with low energy consumption, compressive sensing (CS) has been widely used in wearable health monitoring system for arrhythmia classification of electrocardiogram (ECG) signals. However, most existing works focus on compressive sensing reconstruction, in other words, the ECG signals must be reconstructed before use. Hence, these methods have high computational complexity. In this paper, the authors propose a cardiac arrhythmia classification scheme that performs classification task directly in the compressed domain, skipping the reconstruction stage. The proposed scheme first employs the Pan-Tompkins algorithm to preprocess the ECG signals, including denoising and QRS detection, and then compresses the ECG signals by CS to obtain the compressive measurements. The features are extracted directly from these measurements based on principal component analysis (PCA), and are used to classify the ECG signals into different types by the proposed semi-supervised learning algorithm based on support vector machine (SVM). Extensive simulations have been performed to validate the effectiveness of the proposed scheme. Experimental results have shown that the proposed scheme achieves an average accuracy of 98.0531% at a sensing rate of 0.7, compared to an accuracy of 98.5841% for noncompressive ECG data.
更多查看译文
关键词
Compressive sensing,cardiac arrhythmia,direct classification,semi-supervised learning
AI 理解论文
溯源树
样例
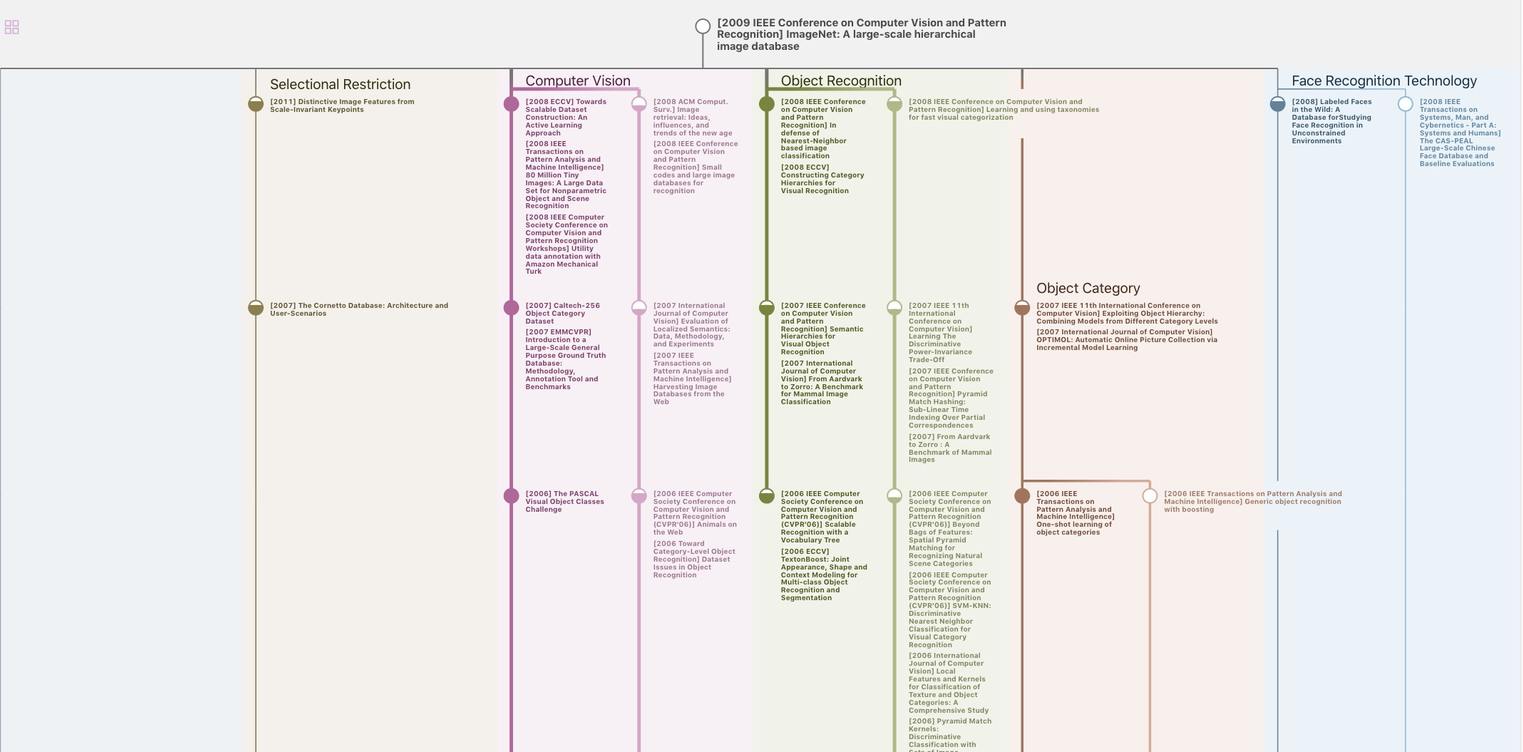
生成溯源树,研究论文发展脉络
Chat Paper
正在生成论文摘要