Are Two (Samples) Really Better Than One? On the Non-Asymptotic Performance of Empirical Revenue Maximization.
arXiv: Computer Science and Game Theory(2018)
摘要
The literature on "mechanism design from samples," which has flourished in recent years at the interface of economics and computer science, offers a bridge between the classic computer-science approach of worst-case analysis (corresponding to "no samples") and the classic economic approach of average-case analysis for a given Bayesian prior (conceptually corresponding to the number of samples tending to infinity). Nonetheless, the two directions studied so far are two extreme and almost diametrically opposed directions: that of asymptotic results where the number of samples grows large, and that where only a single sample is available. In this paper, we take a first step toward understanding the middle ground that bridges these two approaches: that of a fixed number of samples greater than one. In a variety of contexts, we ask what is possibly the most fundamental question in this direction: "are two samples really better than one sample?". We present a few surprising negative results, and complement them with our main result: showing that the worst-case, over all regular distributions, expected-revenue guarantee of the Empirical Revenue Maximization algorithm given two samples is greater than that of this algorithm given one sample. The proof is technically challenging, and provides the first result that shows that some deterministic mechanism constructed using two samples can guarantee more than one half of the optimal revenue.
更多查看译文
关键词
Revenue maximization,empirical revenue maximiation,samples
AI 理解论文
溯源树
样例
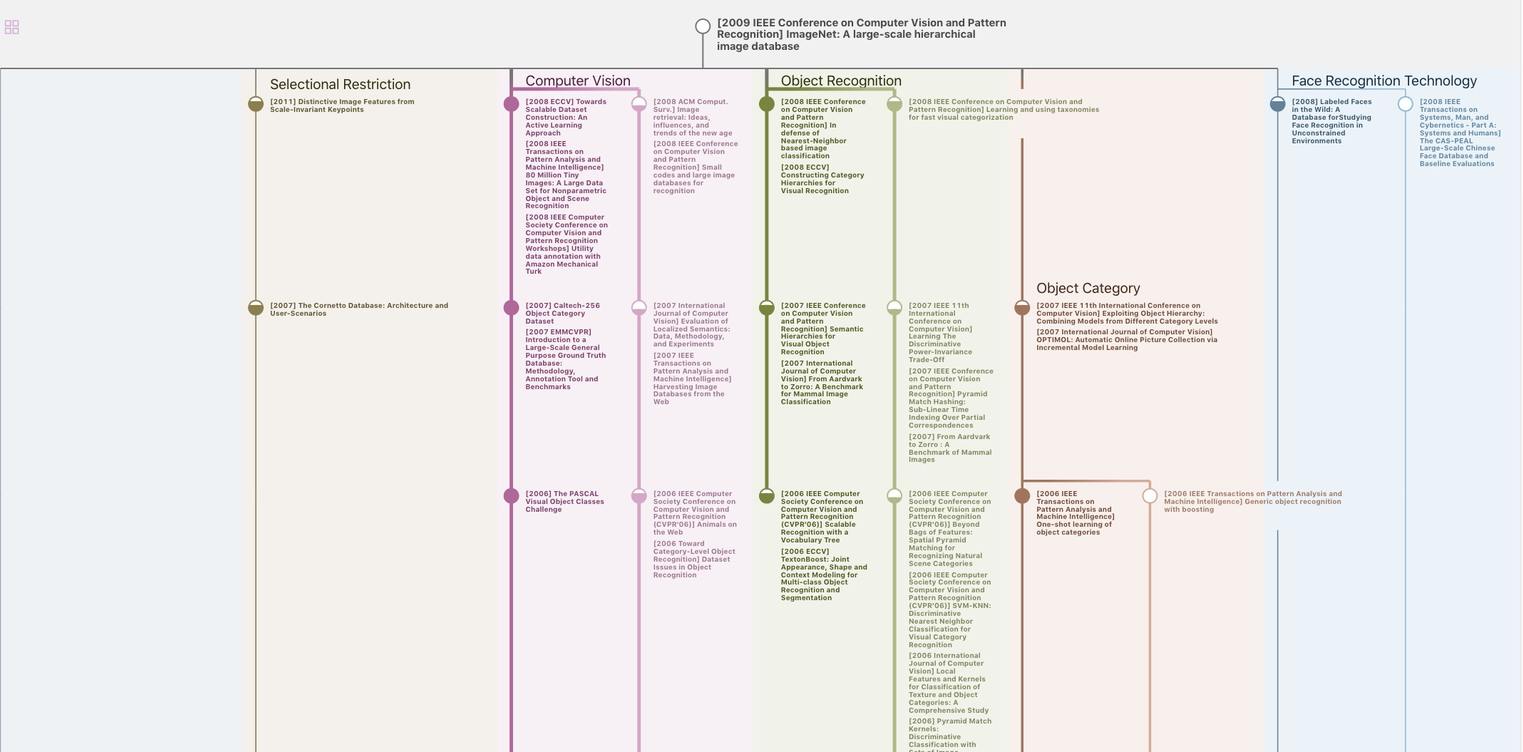
生成溯源树,研究论文发展脉络
Chat Paper
正在生成论文摘要