Coresets For Monotonic Functions with Applications to Deep Learning.
arXiv: Learning(2018)
摘要
Coreset (or core-set) in this paper is a small weighted emph{subset} $Q$ of the input set $P$ with respect to a given emph{monotonic} function $f:mathbb{R}tomathbb{R}$ that emph{provably} approximates its fitting loss $sum_{pin P}f(pcdot x)$ to emph{any} given $xinmathbb{R}^d$. Using $Q$ we can obtain approximation to $x^*$ that minimizes this loss, by running emph{existing} optimization algorithms on $Q$. We provide: (i) a lower bound that proves that there are sets with no coresets smaller than $n=|P|$ , (ii) a proof that a small coreset of size near-logarithmic in $n$ exists for emph{any} input $P$, under natural assumption that holds e.g. for logistic regression and the sigmoid activation function. (iii) a generic algorithm that computes $Q$ in $O(nd+nlog n)$ expected time, (iv) novel technique for improving existing deep networks using such coresets, (v) extensive experimental results with open code.oving existing deep networks using such coresets, (v) extensive experimental results with open code.
更多查看译文
AI 理解论文
溯源树
样例
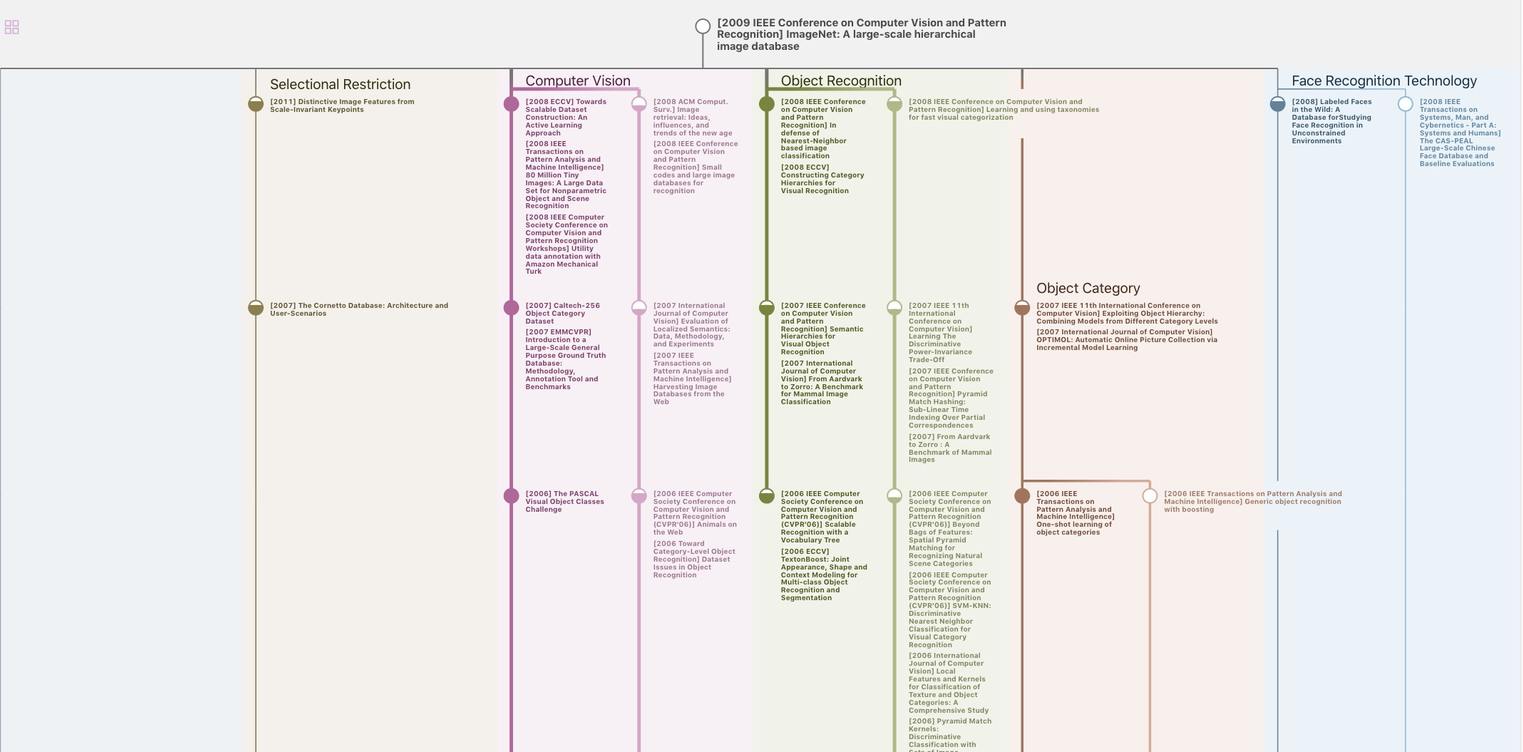
生成溯源树,研究论文发展脉络
Chat Paper
正在生成论文摘要