Learning task hierarchies using statistical semantics and goal reasoning.
AI COMMUNICATIONS(2018)
摘要
This paper describes WORD2HTN, a new approach for learning hierarchical tasks and goals from plan traces in planning domains. Our approach combines semantic text analysis techniques and subgoal learning in order to produce Hierarchical Task Networks (HTNs). Unlike existing HTN learning algorithms, our system uses semantics and similarities of the atoms and actions in the plan traces. WORD2HTN first learns vector representations that represent the semantics and similarities of the atoms occurring in the plan traces. Then the system uses those representations to group atoms occurring in the traces into clusters. Clusters are grouped together into larger clusters based on their similarity. These groupings define a hierarchy of atom clusters. These atom clusters help to define task and goal hierarchies, which can then be transformed into HTN methods and used by an HTN planner for automated planning. We describe our algorithm and present our experimental evaluation.
更多查看译文
关键词
Goal reasoning,learning goal structures,learning HTNs,word embeddings,statistical semantics,clustering
AI 理解论文
溯源树
样例
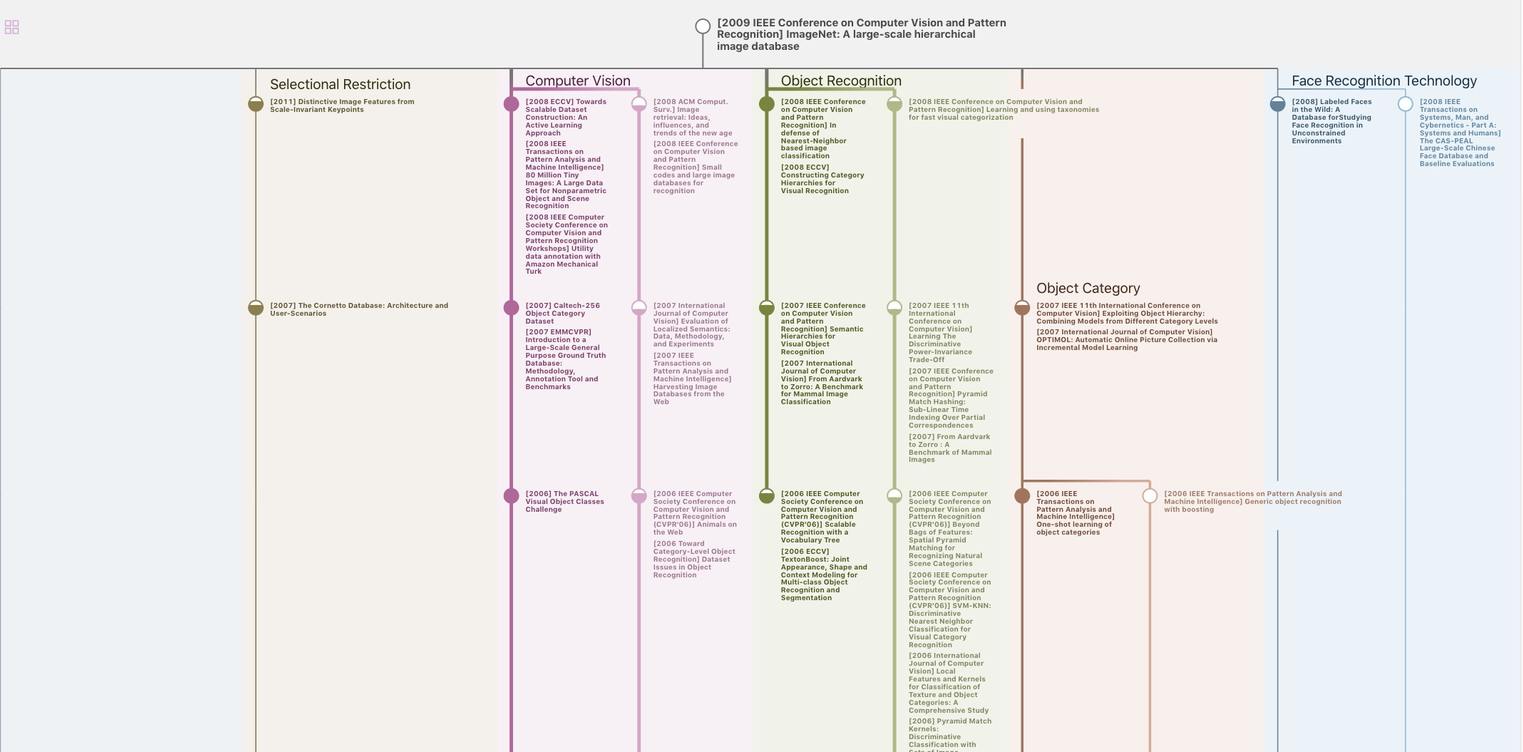
生成溯源树,研究论文发展脉络
Chat Paper
正在生成论文摘要