Multiple Event Detection And Recognition For Large-Scale Power Systems Through Cluster-Based Sparse Coding
IEEE TRANSACTIONS ON POWER SYSTEMS(2017)
摘要
Accurate event analysis in real time is of paramount importance for high-fidelity situational awareness such that proper actions can take place before any isolated faults escalate to cascading blackouts. Existing approaches are limited to detect only single or double events or a specified event type. Although some previous works can well distinguish multiple events in small-scale systems, the performance tends to degrade dramatically in large-scale systems. In this paper, we focus on multiple event detection, recognition, and temporal localization in large-scale power systems. We discover that there always exist "regions" where the reaction of all buses to certain event within each region demonstrates high degree similarity, and that the boundary of the "regions" generally remains the same regardless of the type of event(s). We further verify that, within each region, this reaction to multiple events can be approximated as a linear combination of reactions to each constituent event. Based on these findings, we propose a novel method, referred to as cluster-based sparse coding (CSC), to extract all the underlying single events involved in a multievent scenario. Multiple events of three typical disturbances (e.g., generator trip, line trip, and load shedding) can be detected and recognized. Specifically, the CSC algorithm can effectively distinguish line trip events from oscillation, which has been a very challenging task for event analysis. Experimental results based on simulated large-scale system model (i.e., NPCC) show that the proposed CSC algorithm presents high detection and recognition rate with low false alarms.
更多查看译文
关键词
Classification-based method, cluster-based sparse coding, frequency measurement, large-scale power systems, multievent analysis
AI 理解论文
溯源树
样例
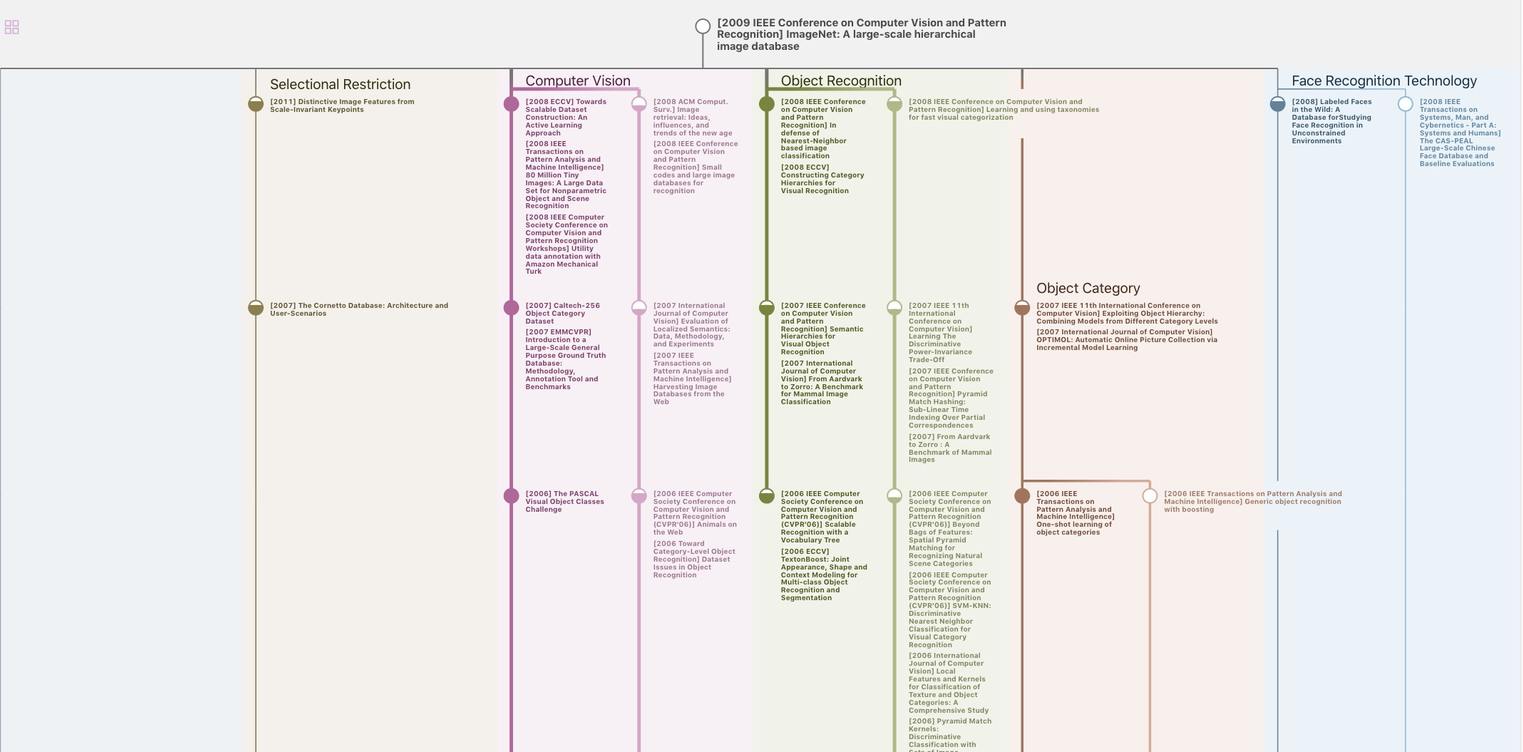
生成溯源树,研究论文发展脉络
Chat Paper
正在生成论文摘要