Impact Of Training Lstm-Rnn With Fuzzy Ground Truth
PROCEEDINGS OF THE 7TH INTERNATIONAL CONFERENCE ON PATTERN RECOGNITION APPLICATIONS AND METHODS (ICPRAM 2018)(2018)
摘要
Most machine learning algorithms follow the supervised learning approach and therefore require annotated training data. The large amount of training data required to train state of the art deep neural networks changed the methods of acquiring the required annotations. User annotations or completely synthetic annotations are becoming more and more prevalent replacing careful manual annotations by experts. In the field of OCR recent work has shown that synthetic ground truth acquired through clustering with minimal manual annotation yields good results when combined with bidirectional LSTM-RNN. Similarly we propose a change to standard LSTM training to handle imperfect manual annotation. When annotating historical documents or low quality scans deciding on the correct annotation is difficult especially for non-experts. Providing all possible annotations in such cases, instead of just one, is what we call fuzzy ground truth. Finally we show that training an LSTM-RNN on fuzzy ground truth achieves a similar performance.
更多查看译文
关键词
Document Analysis, OCR, LSTM, Fuzzy Ground Truth
AI 理解论文
溯源树
样例
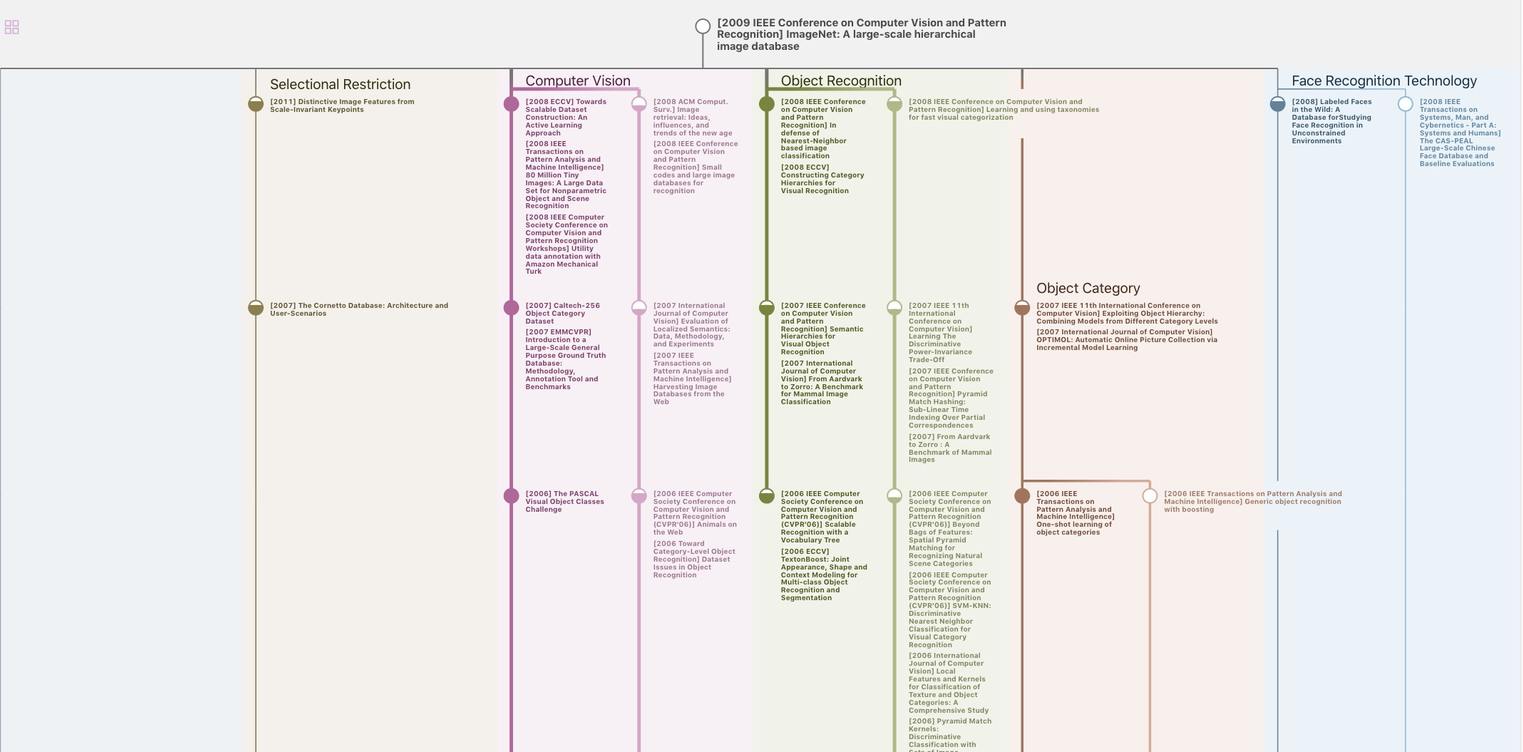
生成溯源树,研究论文发展脉络
Chat Paper
正在生成论文摘要