Elastic deformations for data augmentation in breast cancer mass detection
2018 IEEE EMBS International Conference on Biomedical & Health Informatics (BHI)(2018)
摘要
Two limitations hamper performance of deep architectures for classification and/or detection in medical imaging: (i) the small amount of available data, and (ii) the class imbalance scenario. While millions of labeled images are available today to build classification tools for natural scenes, the amount of available annotated data for automatic breast cancer screening is limited to a few thousand images, at best. We address these limitations with a method for data augmentation, based on the introduction of random elastic deformations on images of mammograms. We validate this method on three publicly available datasets. Our proposed Convolutional Neural Network (CNN) architecture is trained for mass classification - in a conventional way -, and then used in the more interesting problem of mass detection in full mammograms by transforming the CNN into a Fully Convolutional Network (FCN).
更多查看译文
关键词
data augmentation,breast cancer mass detection,deep architectures,medical imaging,labeled images,natural scenes,automatic breast cancer screening,random elastic deformations,mammograms,Convolutional Neural Network architecture,mass classification
AI 理解论文
溯源树
样例
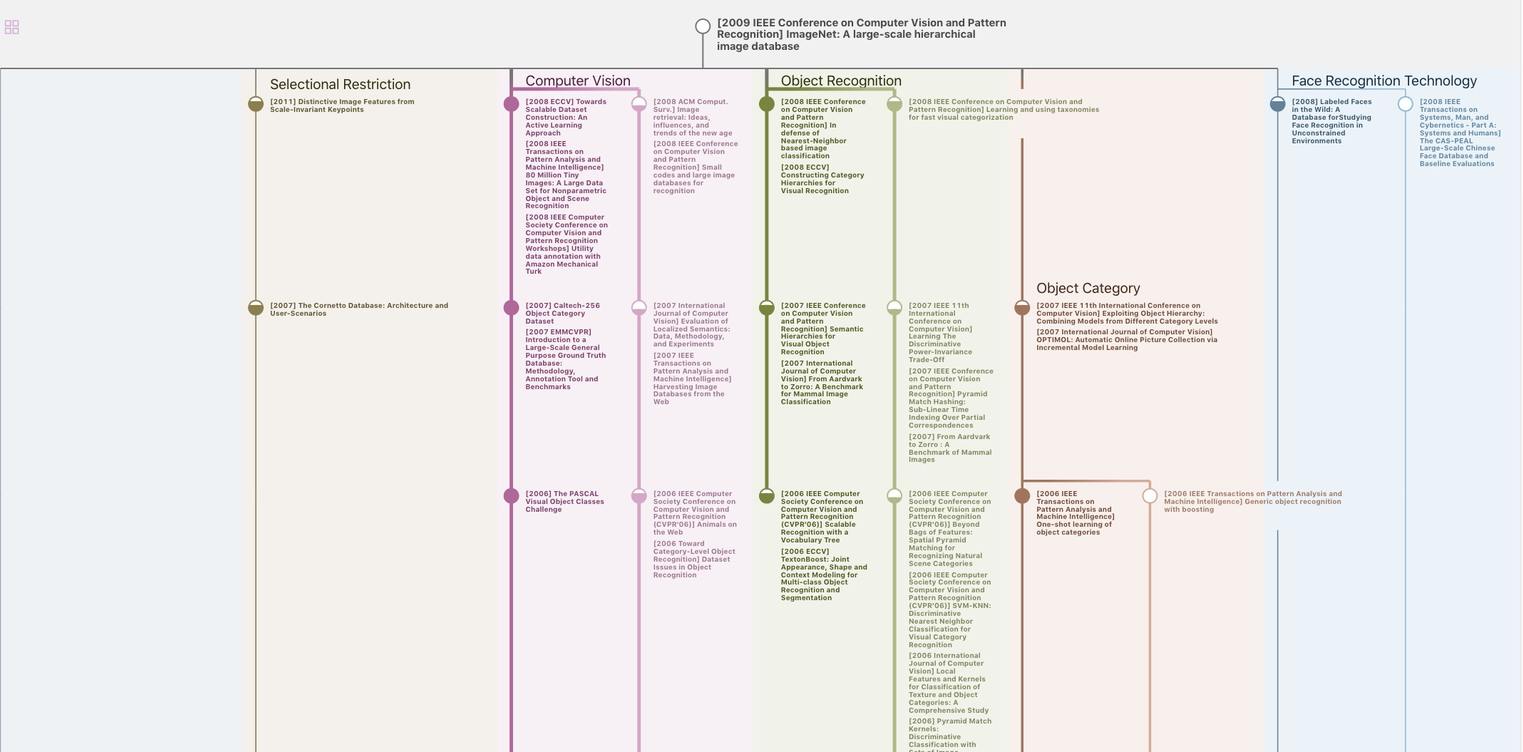
生成溯源树,研究论文发展脉络
Chat Paper
正在生成论文摘要