Compression and Aggregation for Optimizing Information Transmission in Distributed CNN
2017 Fifth International Symposium on Computing and Networking (CANDAR)(2017)
摘要
Modern deep learning has significantly improved the performance and has been used in a variety of applications. Due to the heavy processing cost, major platforms for deep learning have been migrated from commodity computers to the cloud where have huge amount of resources. However, the above situation leads to the slowdown of response time due to severe congestion of the network traffic. To alleviate the overconcentration of data traffic and power consumption, many researchers have paid attention to edge computing. We tackle with the parallel processing model using Deep Convolutional Neural Network (DCNN) employed on multiple devices, and the size reduction of network traffic among the devices. We propose a technique that compresses the intermediate data and aggregates common computation %used in the classification in AlexNet for video recognition. Our experiments demonstrate that Zip loss-less compression reduces the amount of data by up to 1/24, and HEVC lossy compression reduces the amount of data by 1/208 with only 3.5% degradation of the recognition accuracy. Moreover, aggregation of common calculation reduces the amount of computation for 30 DCNNs by 90%.
更多查看译文
关键词
Convolution Neural Network,Edge Computing,Model Parallel,Video Compression,Aggregation
AI 理解论文
溯源树
样例
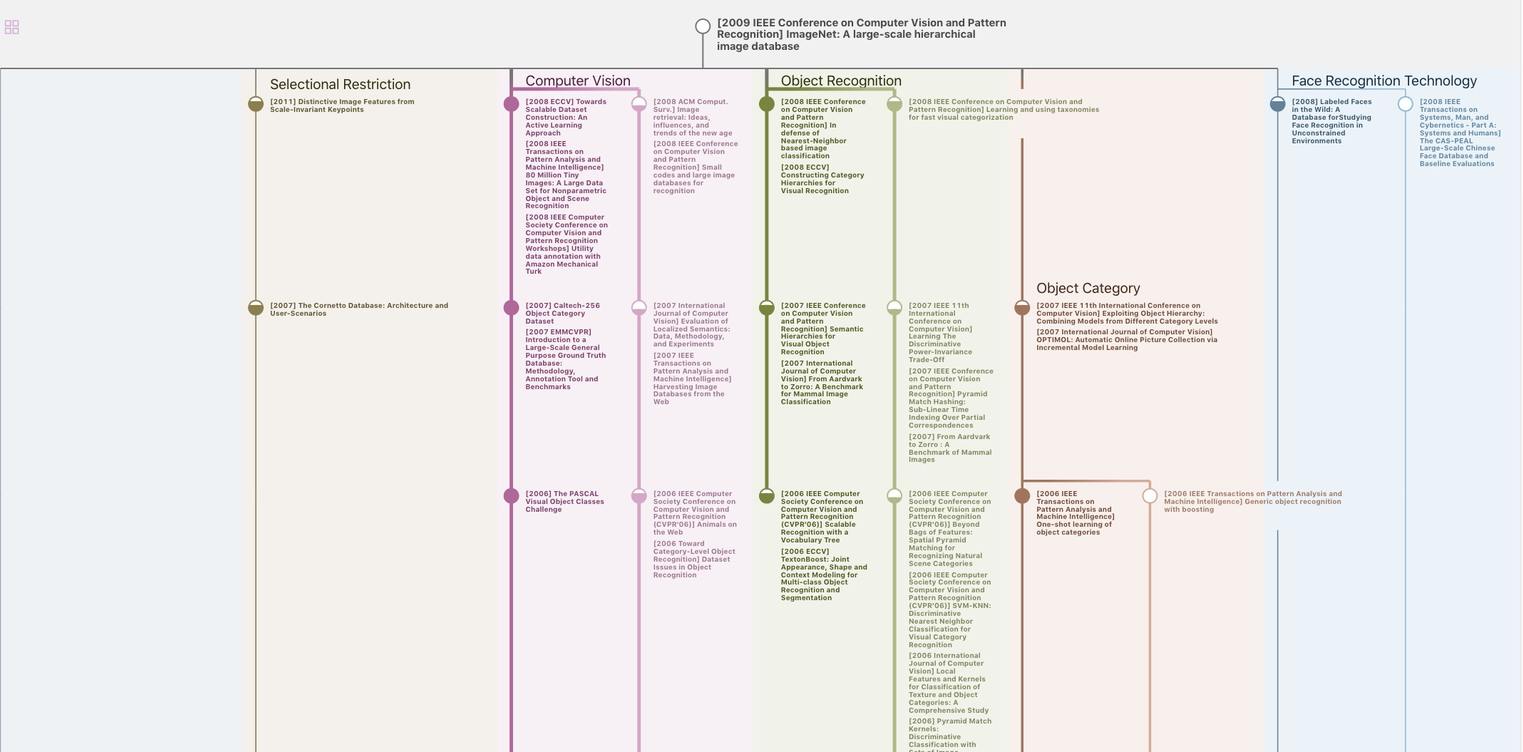
生成溯源树,研究论文发展脉络
Chat Paper
正在生成论文摘要