Efficient And Robust Classification Of Seismic Data Using Nonlinear Support Vector Machines
2017 51st Asilomar Conference on Signals, Systems, and Computers(2017)
摘要
We characterize the robustness and scalability of nonlinear Support Vector Machines (SVM) combined with kernel Principal Component Analysis (kPCA) for the classification of nonlinearly correlated data within the context of geo-structure identification using seismic data. Classification through pattern recognition using supervised learning algorithms such as SVM is popular in many fields. However, the suitability of such methods for classifying seismic data is severely hampered by assumptions of linearity (linear SVM), which affects accuracy, or computational limitations with increases in data dimension (nonlinear SVM). We propose an alternate approach to overcome this limitation, performing nonlinear SVM in a reduced dimensional space determined using kPCA. The utility of the method is demonstrated by characterizing the geologic structure using synthetically generated seismograms. We observe that our method produced a more efficient and robust classifier for seismic data than standard nonlinear SVM. Optimal SVM performance occurs when a subspace that makes up only 10% of the entire feature space is used for the training set. We also observe a greater than five times speedup in computational time between the optimal performance and standard nonlinear SVM. The results indicate that performing kPCA dimension reduction prior to classification can significantly increase performance and robustness.
更多查看译文
关键词
data dimension,standard nonlinear SVM,optimal SVM performance,nonlinear support vector machines,geo-structure identification,linear SVM,kernel principal component analysis,robust seismic classification,nonlinearly correlated data classification,pattern recognition,supervised learning algorithms,kPCA dimension reduction
AI 理解论文
溯源树
样例
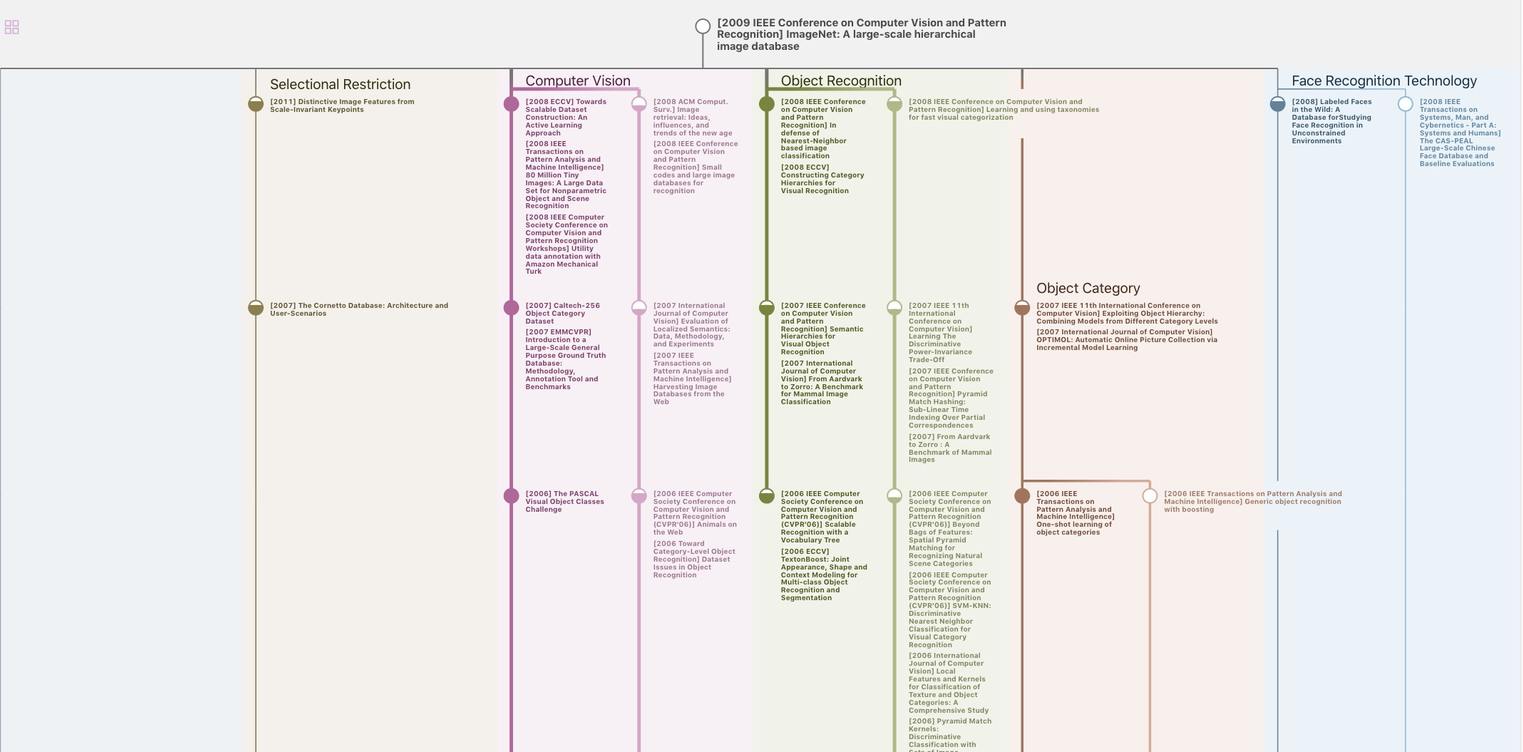
生成溯源树,研究论文发展脉络
Chat Paper
正在生成论文摘要