Generating Schema Labels through Dataset Content Analysis.
WWW '18: The Web Conference 2018 Lyon France April, 2018(2018)
摘要
Impoverished descriptions and convoluted schema labels are common challenges in data-centric tasks such as schema matching and data linking, especially when datasets can span domains. To address these issues, we consider the task of schema label generation. Typically, schema labels are created by dataset providers and are useful for users to understand a dataset. The motivation behind the task is that a lot of data linking systems require overlapping information between two datasets and rely on unique identifiers of schema labels. Moreover, it is common for schema labels in different datasets to have different identifiers even when they refer to the same concept. With no naming standard for schema labels, unintelligible labels are widely found in real-world datasets. For example, many schema labels contain abbreviations and compound nouns that hinder automated matching of attributes in corresponding datasets. Through schema label generation, more common (and thus understandable) schema labels can be provided to allow for broader schema matches in contexts such as dataset search and data linking. We develop a variety of features based on analysis of dataset content to enable machine learning methods to recommend useful labels. We test our approach on two real-world data collections and demonstrate that our method is able to outperform the alternative approach.
更多查看译文
关键词
data linking, text normalization
AI 理解论文
溯源树
样例
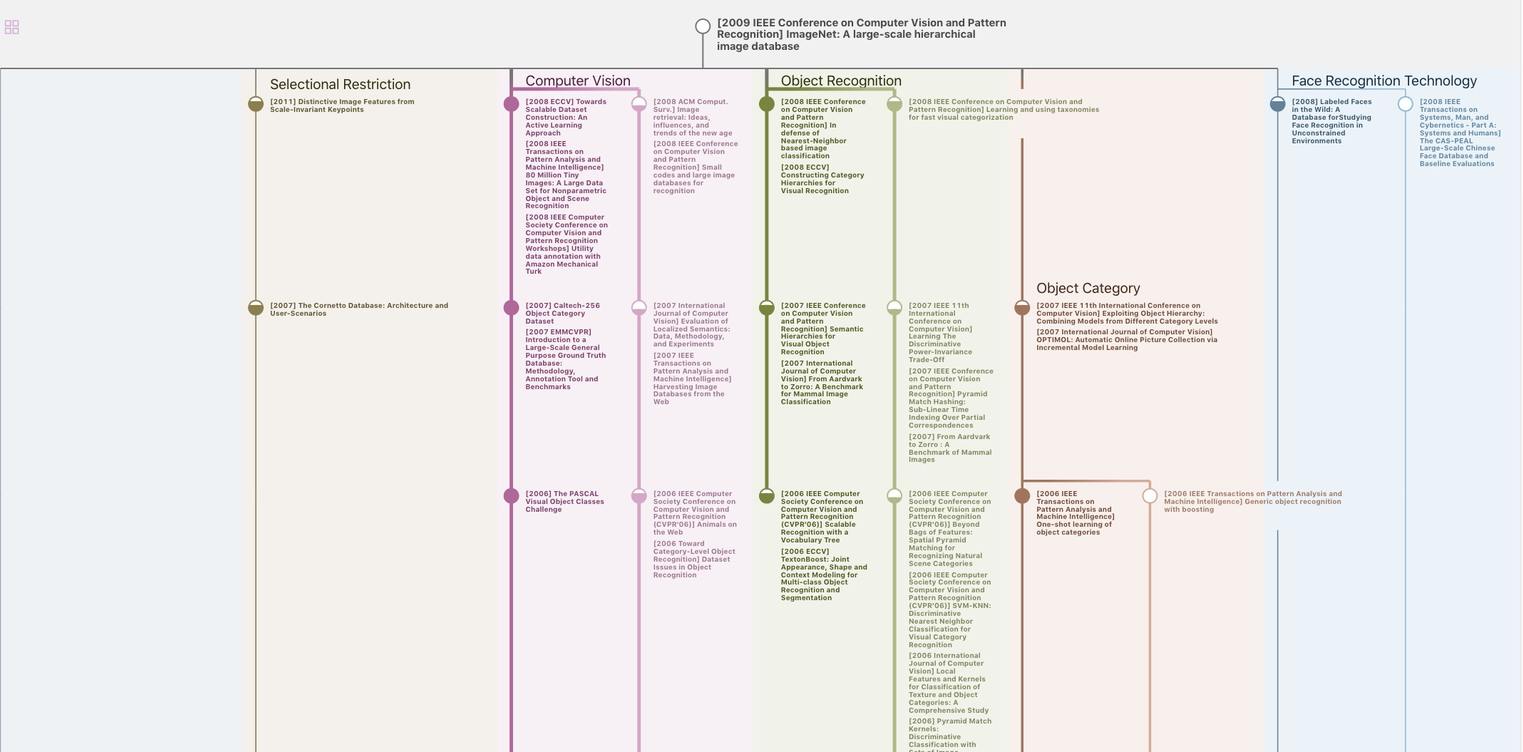
生成溯源树,研究论文发展脉络
Chat Paper
正在生成论文摘要