Anomaly Detection with Partially Observed Anomalies.
WWW '18: The Web Conference 2018 Lyon France April, 2018(2018)
摘要
In this paper, we consider the problem of anomaly detection. Previous studies mostly deal with this task in either supervised or unsupervised manner according to whether label information is available. However, there always exists settings which are different from the two standard manners. In this paper, we address the scenario when anomalies are partially observed, i.e., we are given a large amount of unlabeled instances as well as a handful labeled anomalies. We refer to this problem as anomaly detection with partially observed anomalies, and proposed a two-stage method ADOA to solve it. Firstly, by addressing the difference between the anomalies, the observed anomalies are clustered, while the unlabeled instances are filtered to get potential anomalies and reliable normal instances. Then, with the above instances, a weight is attached to each instance according to the confidence of its label, and a weighted multi-class model is built, which will be further used to distinguish different anomalies to the normal instances. Experimental results show that in the aforementioned setting, existing methods behave unsatisfactorily and the proposed method performs significantly better than all these methods, which validates the effectiveness of the proposed approach.
更多查看译文
关键词
Anomaly Detection, Observed Anomalies, Two-Stage Method
AI 理解论文
溯源树
样例
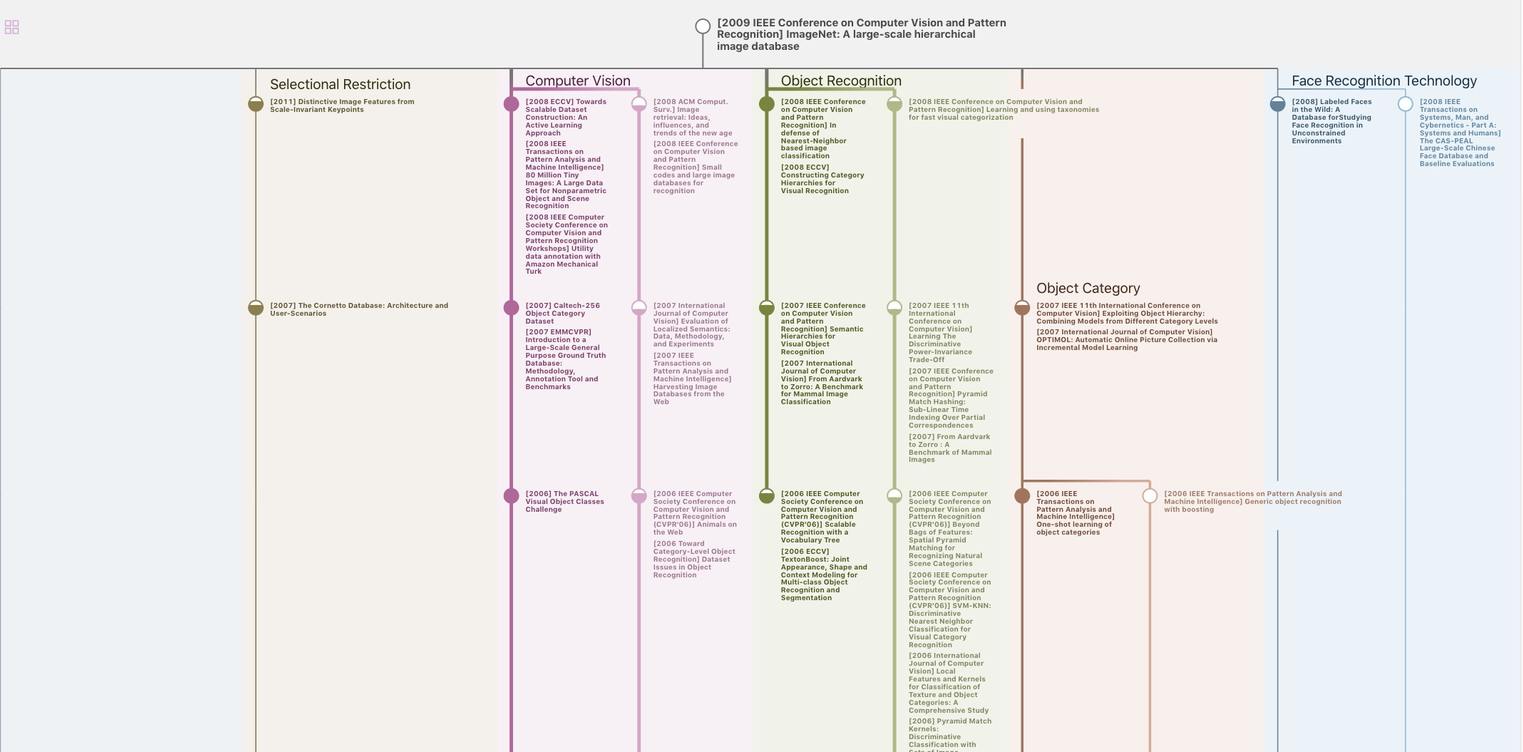
生成溯源树,研究论文发展脉络
Chat Paper
正在生成论文摘要