Attention Convolutional Neural Network for Advertiser-level Click-through Rate Forecasting.
WWW '18: The Web Conference 2018 Lyon France April, 2018(2018)
摘要
Click-through rate (CTR) is a critical problem in online advertising. Most existing researches only focus on the user-level CTR prediction. However, advertiser-level CTR forecasting also plays a very important role because advertisers typically decide how much they would like to bid for advertisements to achieve the maximum clicks given their budget based on CTR forecasting. Over-forecasting will make the advertiser to pay more than necessary but get less return on investment (ROI). Under-forecasting will make the advertiser to spend less money on campaigns but they cannot achieve the desired ROI goals. In this paper, we focus on the advertiser-level CTR forecasting and formulate it as a time series forecasting problem based on the historical CTR record. This is a very challenging problem due to the heavy fluctuation and highly non-linearity of time series. Furthermore, advertisers usually provide useful contextual information for their campaigns, such as text descriptions, targeting locations and devices, which has high correlation with CTR but has not yet been used for CTR forecasting. Thus, we propose a novel context-aware attention convolutional neural network (CACNN), which can capture the high non-linearity and local information of the time series, as well as the underlying correlation between the time series of CTR and the contextual information. To the best of our knowledge, this is the first work employing convolutional neural network and incorporating heterogeneous information to perform CTR forecasting at advertiser level. We implement the system on Yahoo TensorFlowOnSpark platform which enables distributed deep learning on a cluster of GPU and CPU servers, and achieves faster learning speed and data access on HDFS when available. The effectiveness of CACNN model has been demonstrated in real-world Yahoo advertising dataset, and therefore deployed in production with daily rolling of the model.
更多查看译文
关键词
Advertising, Forecasting, Click-Through Rate, Time Series, Attention, Convolutional Neural Network, Sponsored Search
AI 理解论文
溯源树
样例
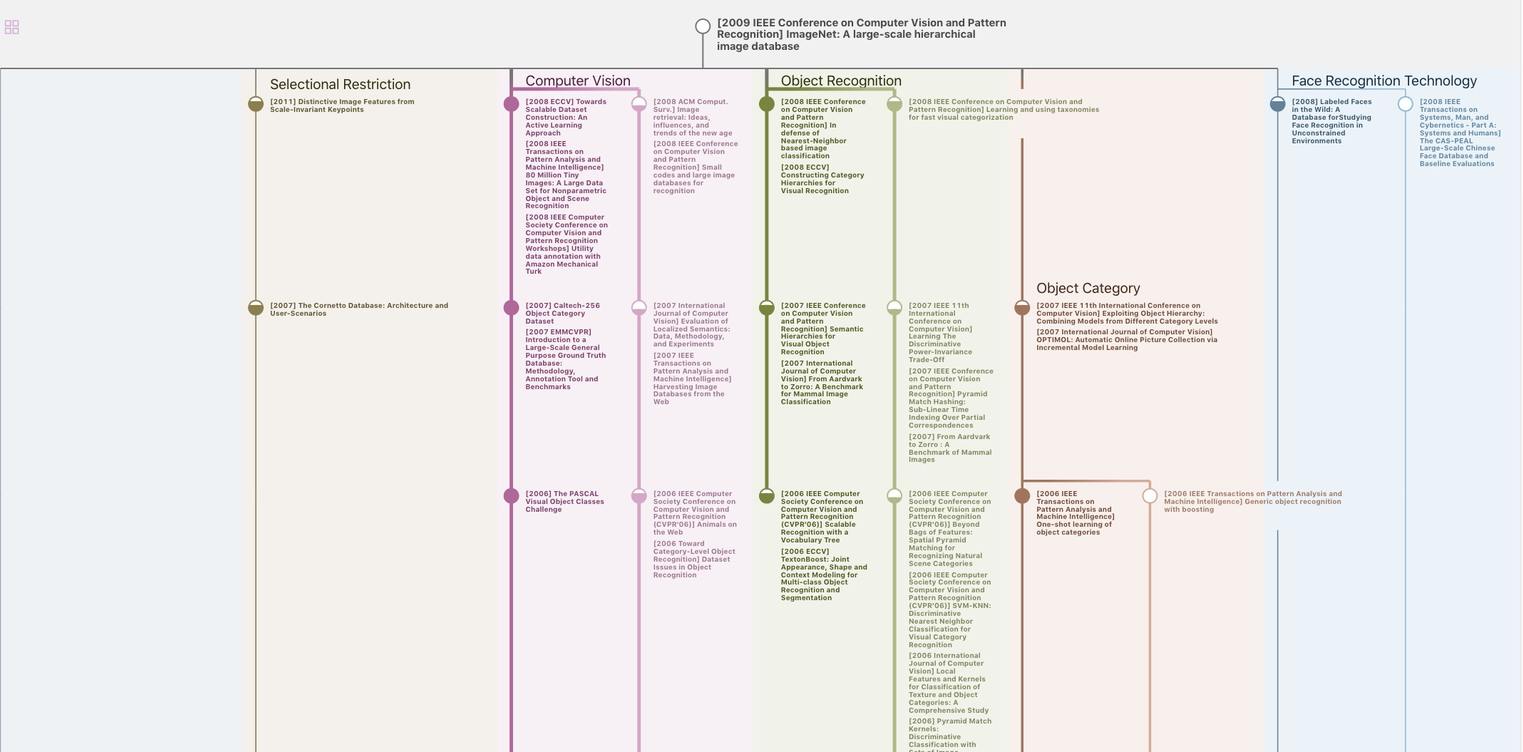
生成溯源树,研究论文发展脉络
Chat Paper
正在生成论文摘要