Human Activity Recognition from Sensor-Based Large-Scale Continuous Monitoring of Parkinson's Disease Patients.
CHASE(2017)
摘要
Smartphone-based assessments have been considered a potential solution to passively monitor gait and mobility in early-stage Parkinsonu0027s disease (PD) patients. In the Multiple Ascending Dose clinical trial of PRX002/RG7935, 44 PD patients and 35 age- and gender-matched healthy individuals performed smartphone-based assessments for up to 24 weeks and up to 6 weeks respectively. For monitoring, subjects carried the smartphone with them as part of their daily routine, while sensors in the smartphone recording movement data continuously. In total, over 30,000 hours of passive monitoring data were collected. To classify the sensor signal into activity profiles, we built a Human Activity Recognition (HAR) model using Deep Neural Networks (DNN) trained on previously published data. The activity profiles of the participants determined by the HAR model showed significant differences between PD patients and healthy controls in the percentage of time walking and frequency in which subjects changed positions (sitting and standing). This combination of sensor data and machine learning-based activity profiling was shown to hold great promise for use in future clinical practice and drug development.
更多查看译文
关键词
sensors,activity recognition,wearable,cell phone,accelerometer,deep learning,Parkinson's disease,clinical trial
AI 理解论文
溯源树
样例
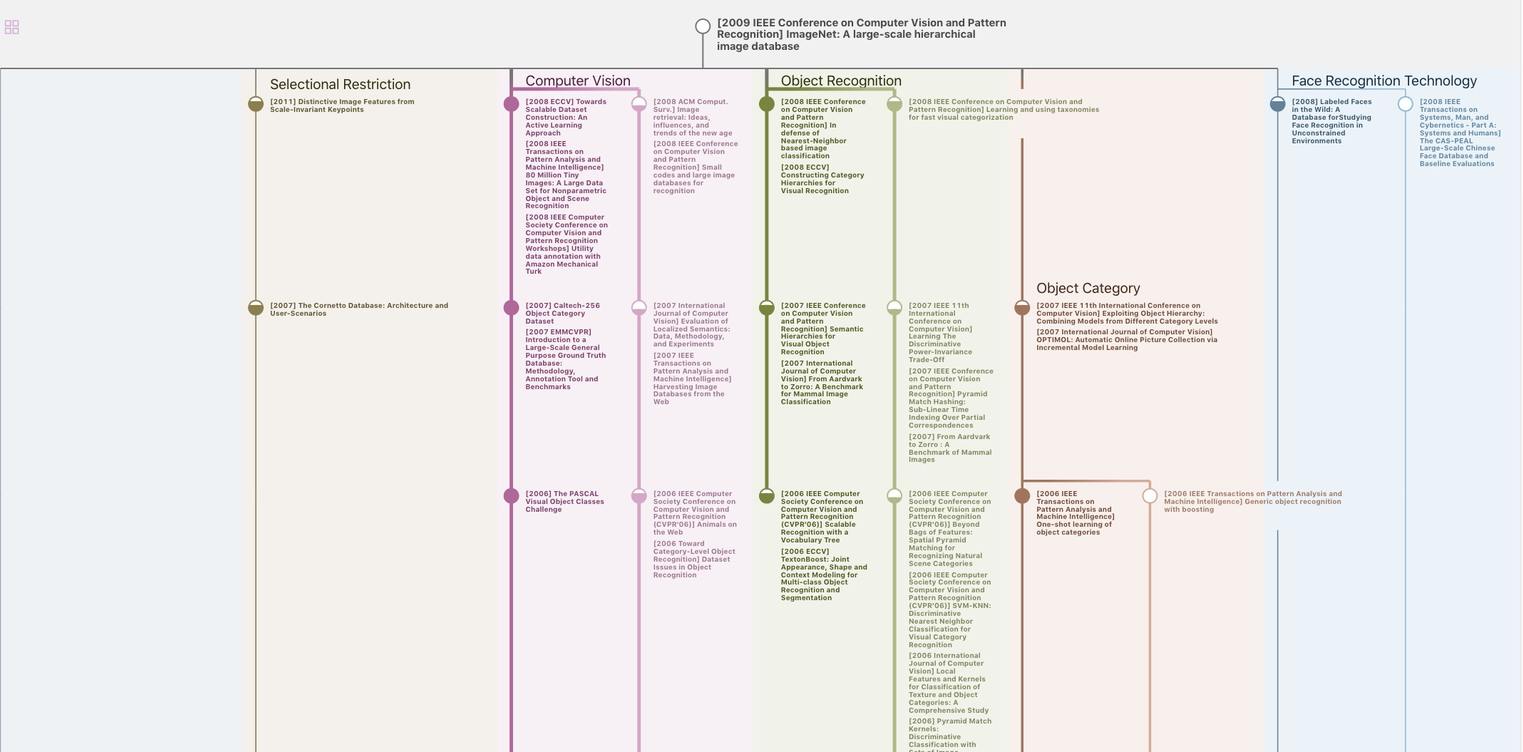
生成溯源树,研究论文发展脉络
Chat Paper
正在生成论文摘要