GPU-accelerated backtracking using CUDA Dynamic Parallelism: GPU-Accelerated Backtracking Using CUDA Dynamic Parallelism
Concurrency and Computation: Practice and Experience(2018)
摘要
New GPGPU technologies, such as CUDA Dynamic Parallelism (CDP), can help dealing with recursive patterns of computation, such as divide-and-conquer, used by backtracking algorithms. In this paper, we propose a GPU-accelerated backtracking algorithm using CDP that extends a well-known parallel backtracking model. The search starts on CPU, processing the search tree until a first cutoff depth. Based on this partial backtracking tree, the algorithm analyzes the memory requirements of subsequent kernel generations. The proposed algorithm performs no dynamic allocation of memory on GPU, unlike related works from the literature. The proposed algorithm has been extensively tested using the N-Queens Puzzle problem and instances of the Asymmetric Traveling Salesman Problem (ATSP) as test-cases. The proposed CDP algorithm may, under some conditions, outperform its non-CDP counterpart by a factor up to 25. But, it may also be up to twice slower. The CDP-based implementation has much better worst case execution times and makes algorithm's performance less dependent on the tuning of parameters. Compared to other CDP-based strategies from the literature, the proposed algorithm is on average 8x faster. The proposed algorithm is also hybridized with another CDP-based strategy from the literature. The combination of strategies is in average 4.5x faster than the related strategy. We also identify some difficulties, limitations, and bottlenecks concerning the CDP programming model which may be useful for helping potential users.
更多查看译文
关键词
CUDA dynamic parallelism,depth-first search,GPU computing,parallel backtracking
AI 理解论文
溯源树
样例
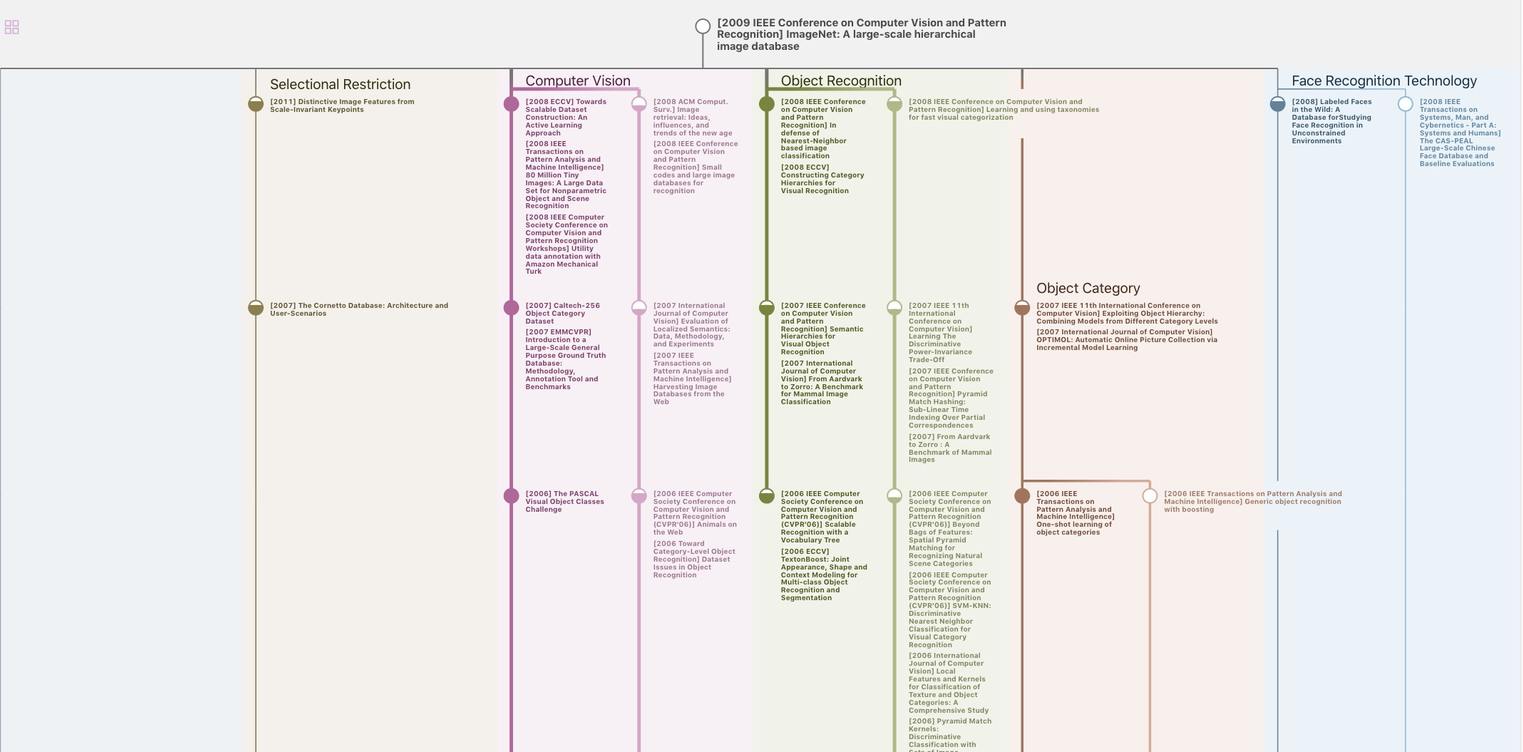
生成溯源树,研究论文发展脉络
Chat Paper
正在生成论文摘要