Asymptotic Confidence Regions for High-Dimensional Structured Sparsity
IEEE transactions on signal processing(2018)
摘要
In the setting of high-dimensional linear regression models, we propose two frameworks for constructing pointwise and group confidence sets for penalized estimators, which incorporate prior knowledge about the organization of the nonzero coefficients. This is done by desparsifying the estimator by S. van de Geer and B. Stucky and S. van de Geer et al., then using an appropriate estimator for the precision matrixT. In order to estimate the precision matrix a corresponding structured matrix norm penalty has to be introduced. After normalization the result is an asymptotic pivot. The asymptotic behavior is studied and simulations are added to study the differences between the two schemes.
更多查看译文
关键词
Asymptotic confidence regions,structured sparsity,high-dimensional linear regression,penalization
AI 理解论文
溯源树
样例
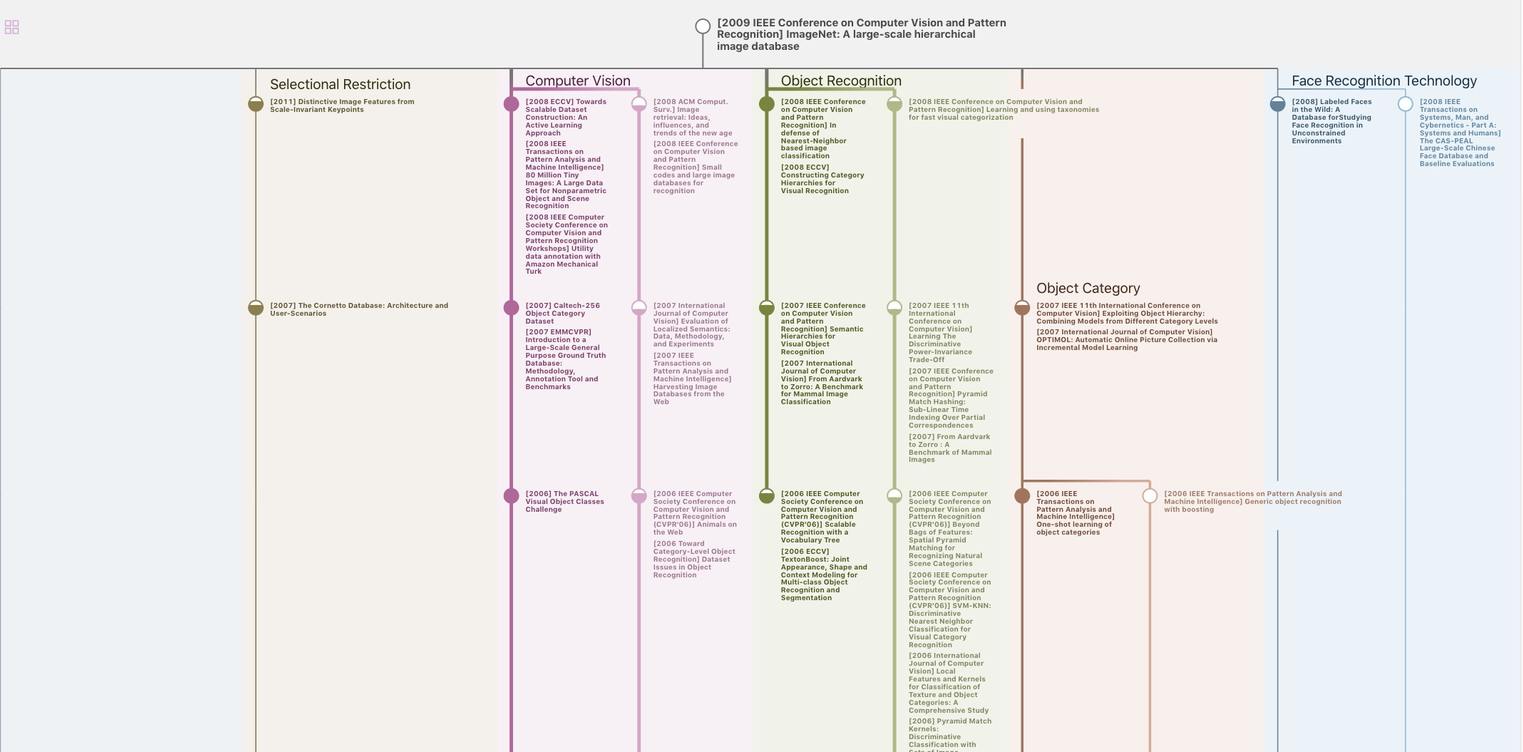
生成溯源树,研究论文发展脉络
Chat Paper
正在生成论文摘要