Discovering pan-correlation patterns from time course data sets by efficient mining algorithms
Computing(2018)
摘要
Time-course correlation patterns can be positive or negative, and time-lagged with gaps. Mining all these correlation patterns help to gain broad insights on variable dependencies. Here, we prove that diverse types of correlation patterns can be represented by a generalized form of positive correlation patterns. We prove a correspondence between positive correlation patterns and sequential patterns, and present an efficient single-scan algorithm for mining the correlations. Evaluations on synthetic time course data sets, and yeast cell cycle gene expression data sets indicate that: (1) the algorithm has linear time increment in terms of increasing number of variables; (2) negative correlation patterns are abundant in real-world data sets; and (3) correlation patterns with time lags and gaps are abundant. Existing methods have only discovered incomplete forms of many of these patterns, and have missed some important patterns completely.
更多查看译文
关键词
Pan-correlation pattern,Time-course data,Positive correlation patterns,Negative correlation patterns,Time-lagged positive correlation patterns,Time-lagged negative correlation patterns,68R01 (General)
AI 理解论文
溯源树
样例
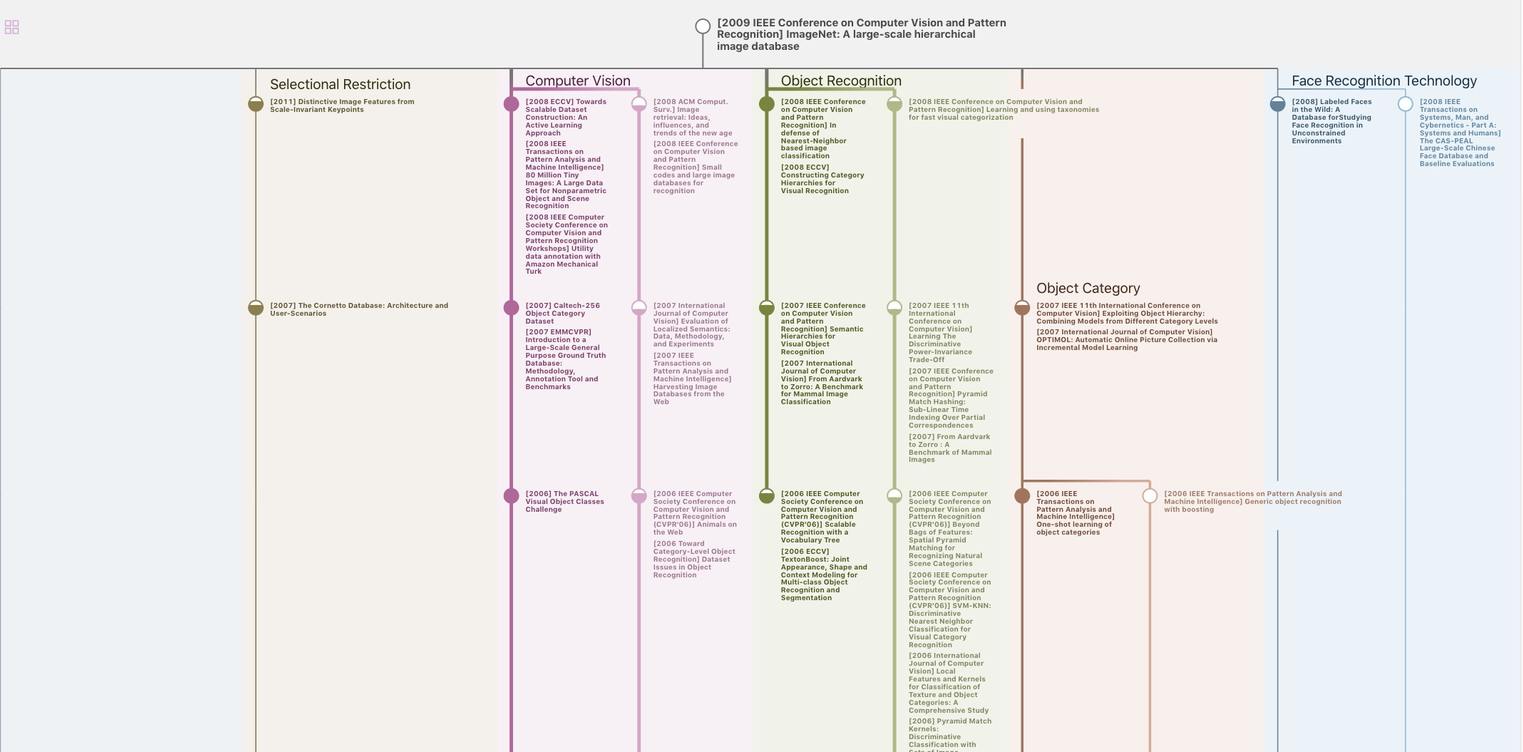
生成溯源树,研究论文发展脉络
Chat Paper
正在生成论文摘要