Fréchet ChemblNet Distance: A metric for generative models for molecules.
arXiv: Learning(2018)
摘要
The new wave of successful generative models in machine learning has increased the interest in deep learning driven de novo drug design. However, assessing the performance of such generative models is notoriously difficult. Metrics that are typically used to assess the performance of such generative models are the percentage of chemically valid molecules or the similarity to real molecules in terms of particular descriptors, such as the partition coefficient (logP) or druglikeness. However, method comparison is difficult because of the inconsistent use of evaluation metrics, the necessity for multiple metrics, and the fact that some of these measures can easily be tricked by simple rule-based systems. We propose a novel distance measure between two sets of molecules, called Fru0027echet ChemblNet distance (FCD), that can be used as an evaluation metric for generative models. The FCD is similar to a recently established performance metric for comparing image generation methods, the Fru0027echet Inception Distance (FID). Whereas the FID uses one of the hidden layers of InceptionNet, the FCD utilizes the penultimate layer of a deep neural network called ChemblNet, which was trained to predict drug activities. Thus, the FCD metric takes into account chemically and biologically relevant information about molecules, and also measures the diversity of the set via the distribution of generated molecules. The FCDu0027s advantage over previous metrics is that it can detect if generated molecules are a) diverse and have similar b) chemical and c) biological properties as real molecules. We further provide an easy-to-use implementation that only requires the SMILES representation of the generated molecules as input to calculate the FCD. Implementations are available at: this https URL
更多查看译文
AI 理解论文
溯源树
样例
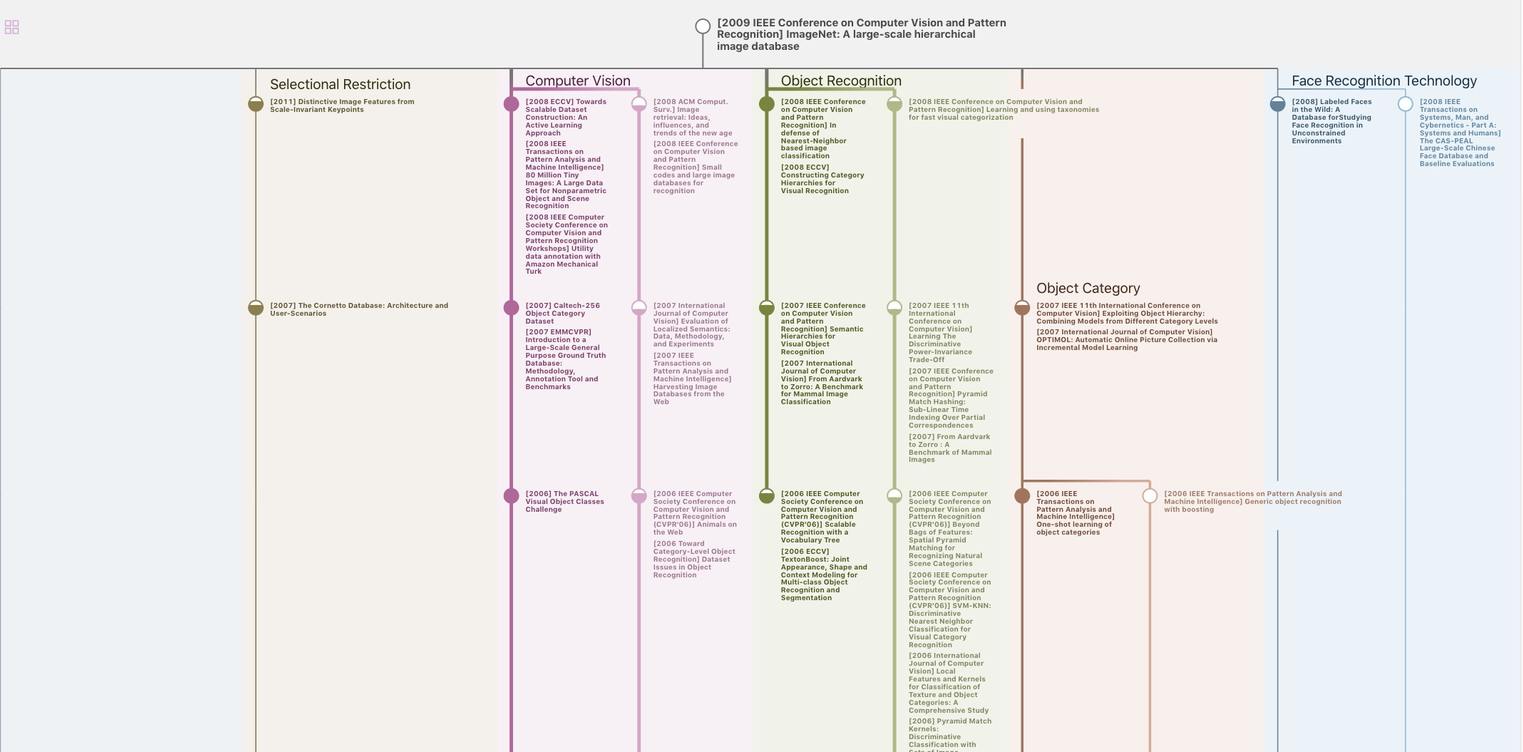
生成溯源树,研究论文发展脉络
Chat Paper
正在生成论文摘要