Combating Adversarial Attacks Using Sparse Representations.
ICLR(2018)
摘要
It is by now well-known that small adversarial perturbations can induce classification errors in deep neural networks (DNNs). In this paper, we make the case that sparse representations of the input data are a crucial tool for combating such attacks. For classifiers, we show that a sparsifying front end is provably effective against $ell_{infty}$-bounded attacks, reducing output distortion due to the attack by a factor of roughly $K / N$ where $N$ is the data dimension and $K$ is the sparsity level. We then extend this concept to DNNs, showing that a locally linear model can be used to develop a theoretical foundation for crafting attacks and defenses. Experimental results for the MNIST dataset show the efficacy of the proposed sparsifying front end.
更多查看译文
AI 理解论文
溯源树
样例
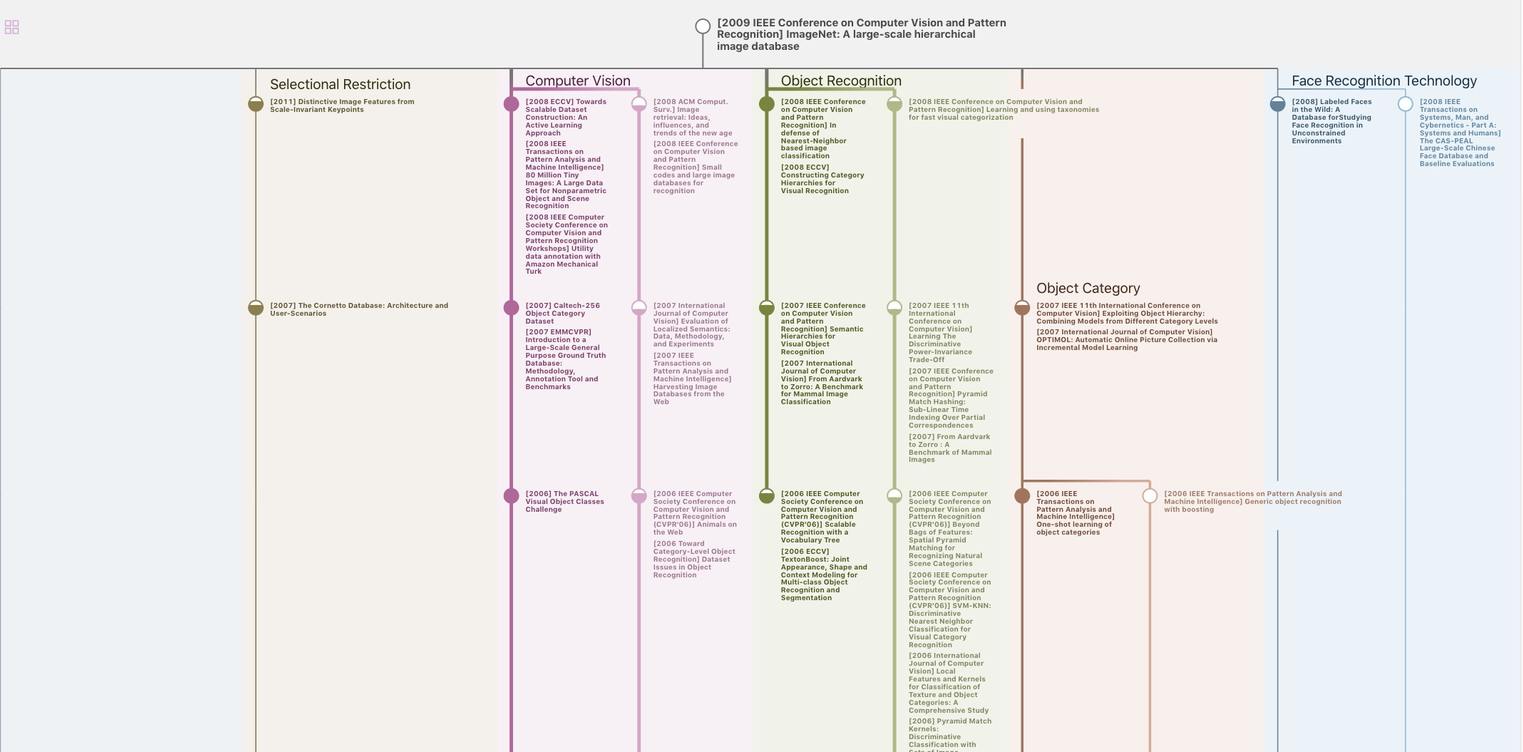
生成溯源树,研究论文发展脉络
Chat Paper
正在生成论文摘要