Domain Adaptation on Graphs by Learning Aligned Graph Bases
IEEE Transactions on Knowledge and Data Engineering(2022)
摘要
A common assumption in semi-supervised learning is that the class label function has a slow variation on the data graph, while in many problems, the label function may vary abruptly in certain graph regions, resulting in high-frequency components. Although semi-supervised learning is an ill-posed problem, it is often possible to find a source graph on which the label function has similar frequency content to the target graph where the actual classification problem is defined. In this paper, we propose a method for domain adaptation on graphs based on learning the spectrum of the label function in a source graph with many labels, and transferring the spectrum information to the target graph. When transferring the frequency content, it is not easy to share graph Fourier coefficients directly between the two independently constructed graphs, since no match exists between their Fourier bases. We solve this by learning a transformation between the Fourier bases of the two graphs that flexibly "aligns" them. The unknown class label function on the target graph is then reconstructed from the learnt spectrum while retaining consistency with the available labels. Comparative experiments suggest that the proposed algorithm often outperforms recent domain adaptation methods in various data classification applications.
更多查看译文
关键词
Face,Laplace equations,Semisupervised learning,Fourier transforms,Frequency-domain analysis,Manifolds,Social networking (online),Domain adaptation,data classification,graph Fourier transform,graph Laplacian,spectrum transfer
AI 理解论文
溯源树
样例
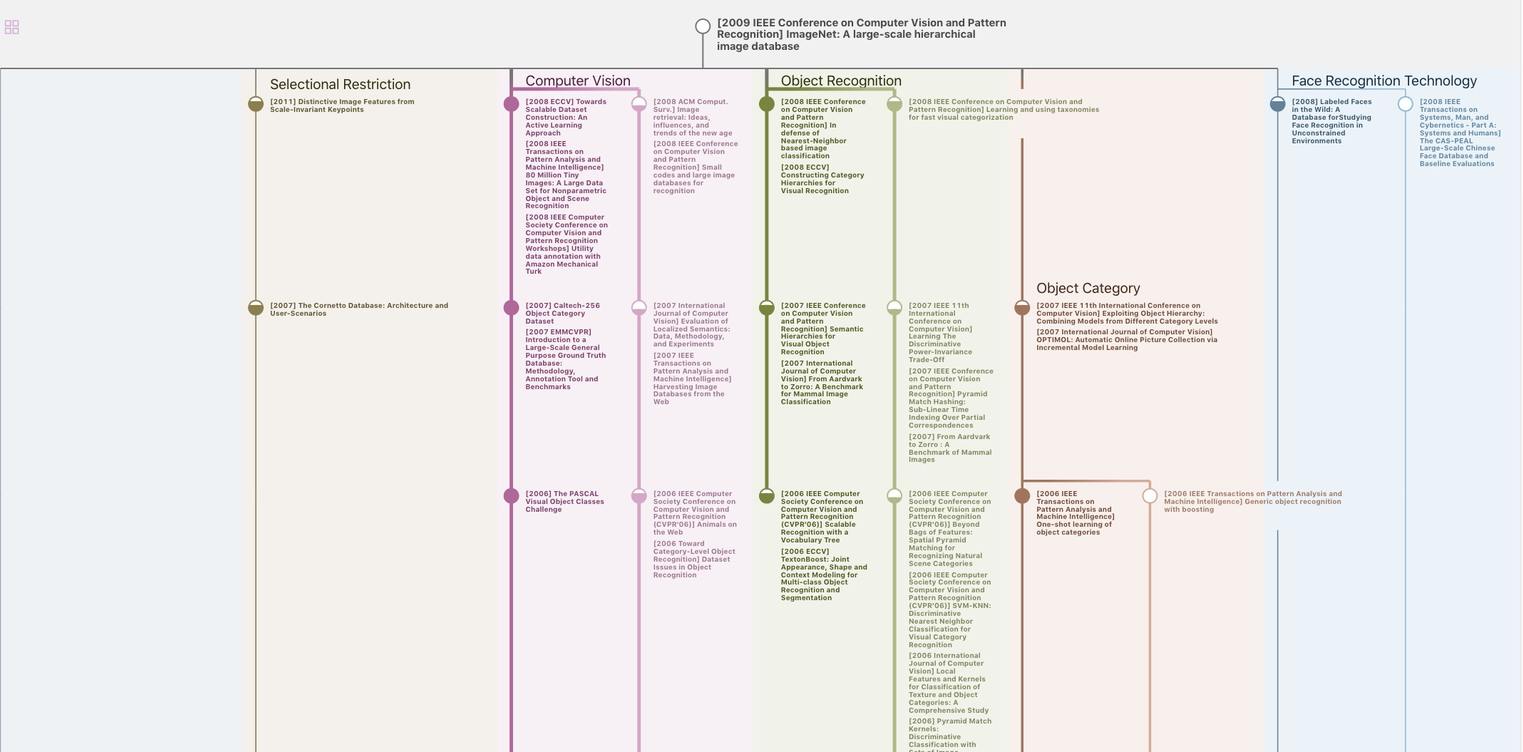
生成溯源树,研究论文发展脉络
Chat Paper
正在生成论文摘要