Online Learning: Sufficient Statistics and the Burkholder Method.
COLT(2018)
摘要
We uncover a fairly general principle: If regret can be (approximately) expressed as a function of certain for the data sequence, then there exists a special Burkholder function that only depends on these sufficient statistics, not the entire data sequence. This function can be used algorithmically to achieve the regret bound, and the online strategy is only required to keep these sufficient statistics in memory. The characterization is achieved by bringing the full power of the Burkholder Method --- originally developed for certifying probabilistic martingale inequalities --- to bear on the online learning setting. To demonstrate the power of the proposed method, we develop a novel online strategy for matrix prediction that attains a regret bound corresponding to the variance term in matrix concentration inequalities. We present a linear-time/space prediction strategy for parameter free supervised learning with linear classes and general smooth norms.
更多查看译文
AI 理解论文
溯源树
样例
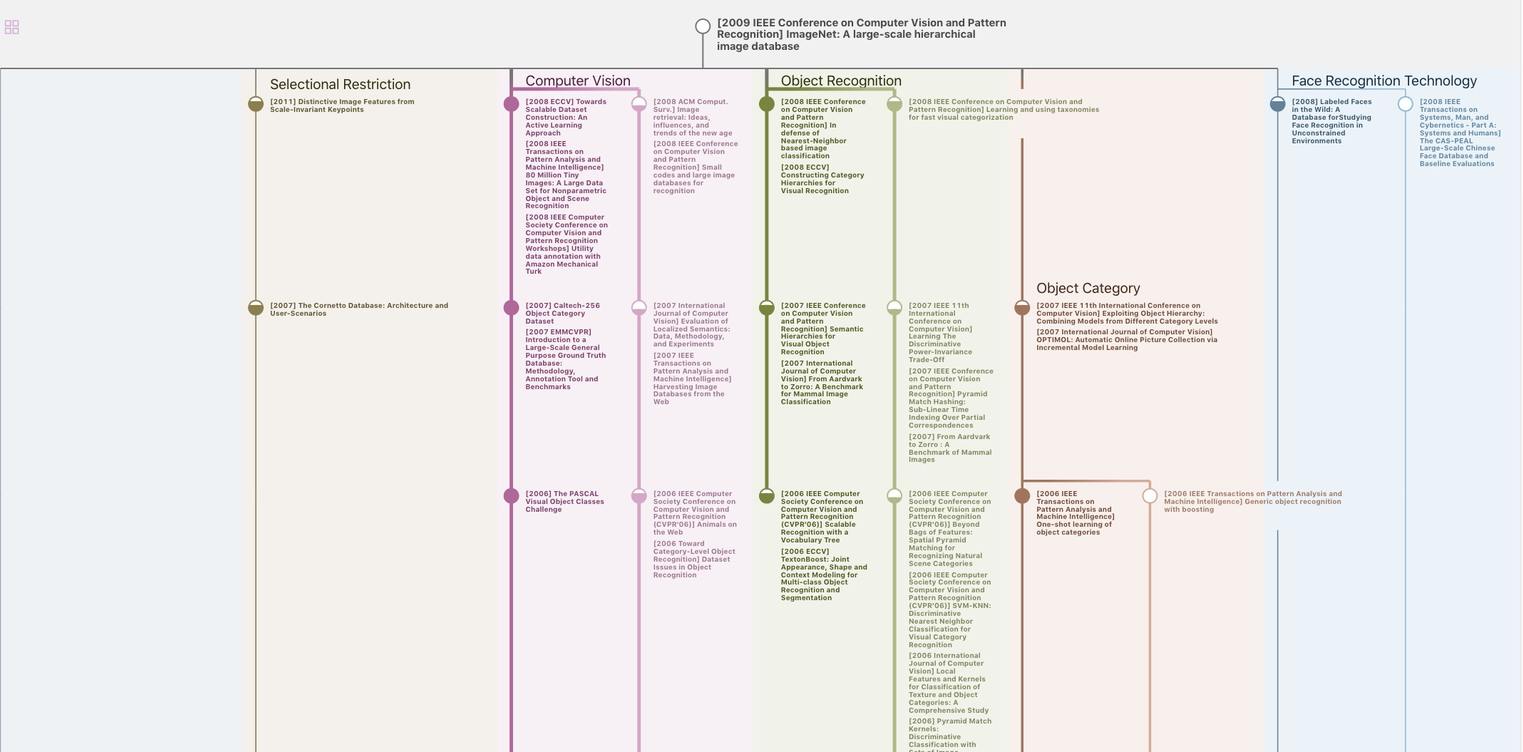
生成溯源树,研究论文发展脉络
Chat Paper
正在生成论文摘要