Reactive NaN Repair for Applying Approximate Memory to Numerical Applications.
arXiv: Distributed, Parallel, and Cluster Computing(2018)
摘要
Applications in the AI and HPC fields require much memory capacity, and the amount of energy consumed by main memory of server machines is ever increasing. Energy consumption of main memory can be greatly reduced by applying approximate computing in exchange for increased bit error rates. AI and HPC applications are to some extent robust to bit errors because small numerical errors are amortized by their iterative nature. However, a single occurrence of a NaN due to bit-flips corrupts the whole calculation result. The issue is that fixing every bit-flip using ECC incurs too much overhead because the bit error rate is much higher than in normal environments. We propose a low-overhead method to fix NaNs when approximate computing is applied to main memory. The main idea is to reactively repair NaNs while leaving other non-fatal numerical errors as-is to reduce the overhead. We implemented a prototype by leveraging floating-point exceptions of x86 CPUs, and the preliminary evaluations showed that our method incurs negligible overhead.
更多查看译文
AI 理解论文
溯源树
样例
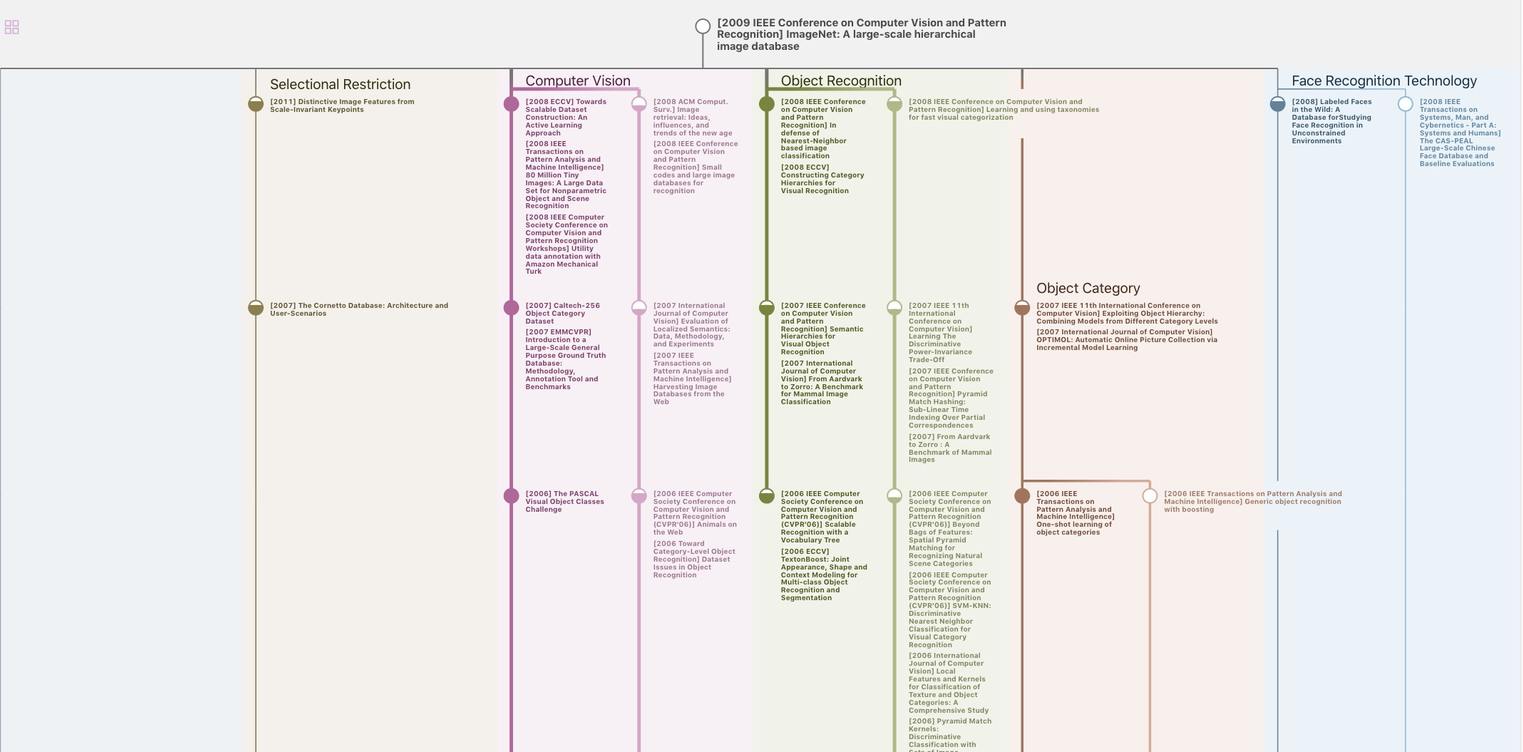
生成溯源树,研究论文发展脉络
Chat Paper
正在生成论文摘要