Quantitatively Evaluating GANs With Divergences Proposed for Training.
ICLR(2018)
摘要
Generative adversarial networks (GANs) have made enormous progress in terms of theory and application in the machine learning and computer vision communities. Even though substantial progress has been made, a lack of quantitative model assessment is still a serious issue. This has led to a huge number of GAN variants being proposed, with relatively little understanding of their relative abilities. In this paper, we evaluate the performance of various types of GANs using divergence and distance functions typically used only for training. We observe consistency across the various proposed metrics, and interestingly, the test-time metrics do not favour networks that use the same training-time criterion. Finally, we measure the statistically correlation between different metrics to human perceptual scores.
更多查看译文
AI 理解论文
溯源树
样例
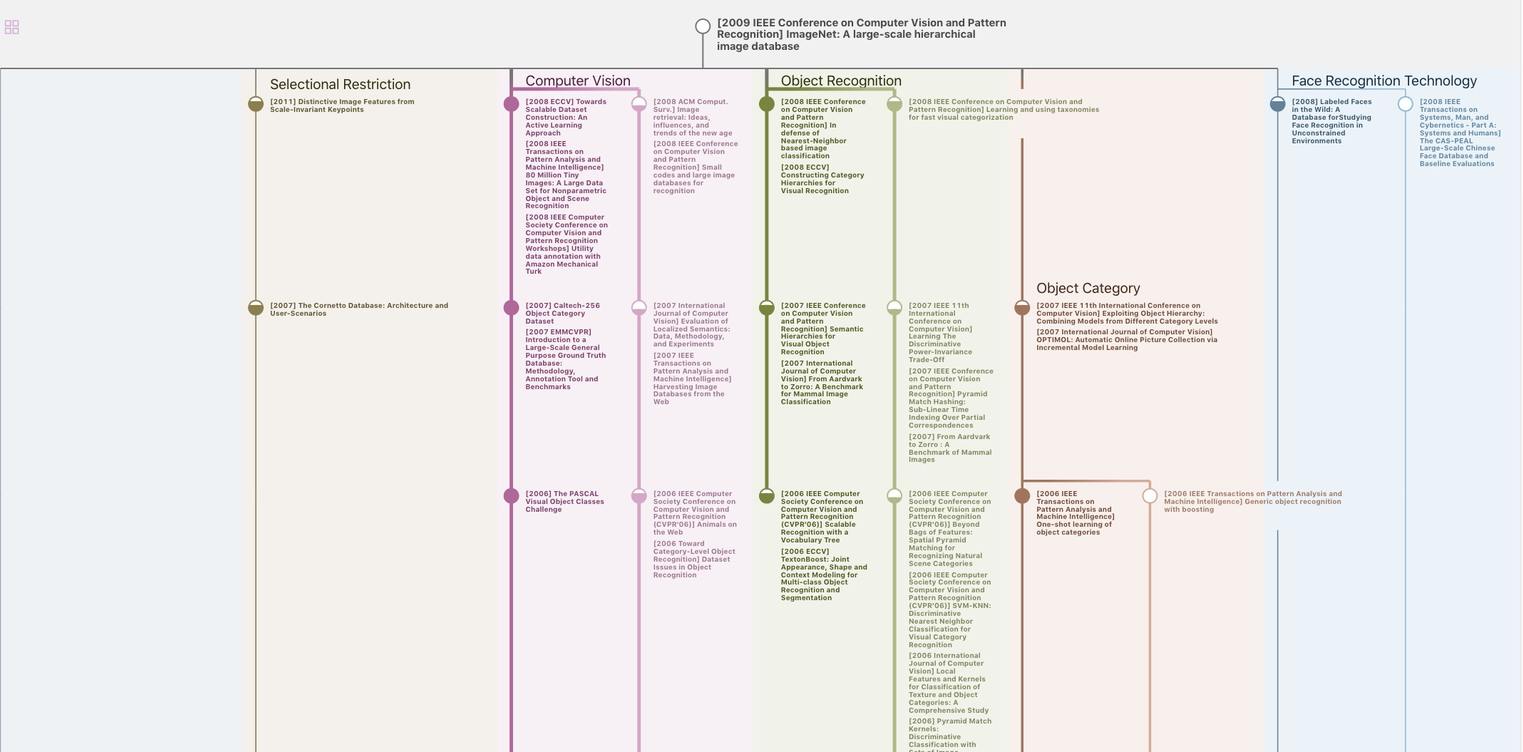
生成溯源树,研究论文发展脉络
Chat Paper
正在生成论文摘要