Scalable Breadth-First Search on a GPU Cluster
2018 IEEE International Parallel and Distributed Processing Symposium (IPDPS)(2018)
摘要
On a GPU cluster, the ratio of high computing power to communication bandwidth makes scaling breadth-first search (BFS) on a scale-free graph extremely challenging. By separating high and low out-degree vertices, we present an implementation with scalable computation and a model for scalable communication for BFS and direction-optimized BFS. Our communication model uses global reduction for high-degree vertices, and point-to-point transmission for low-degree vertices. Leveraging the characteristics of degree separation, we reduce the graph size to one third of the conventional edge list representation. With several other optimizations, we observe linear weak scaling as we increase the number of GPUs, and achieve 259.8 GTEPS on a scale-33 Graph500 RMAT graph with 124 GPUs on the latest CORAL early access system.
更多查看译文
关键词
multi GPU,distributed graph processing,BFS
AI 理解论文
溯源树
样例
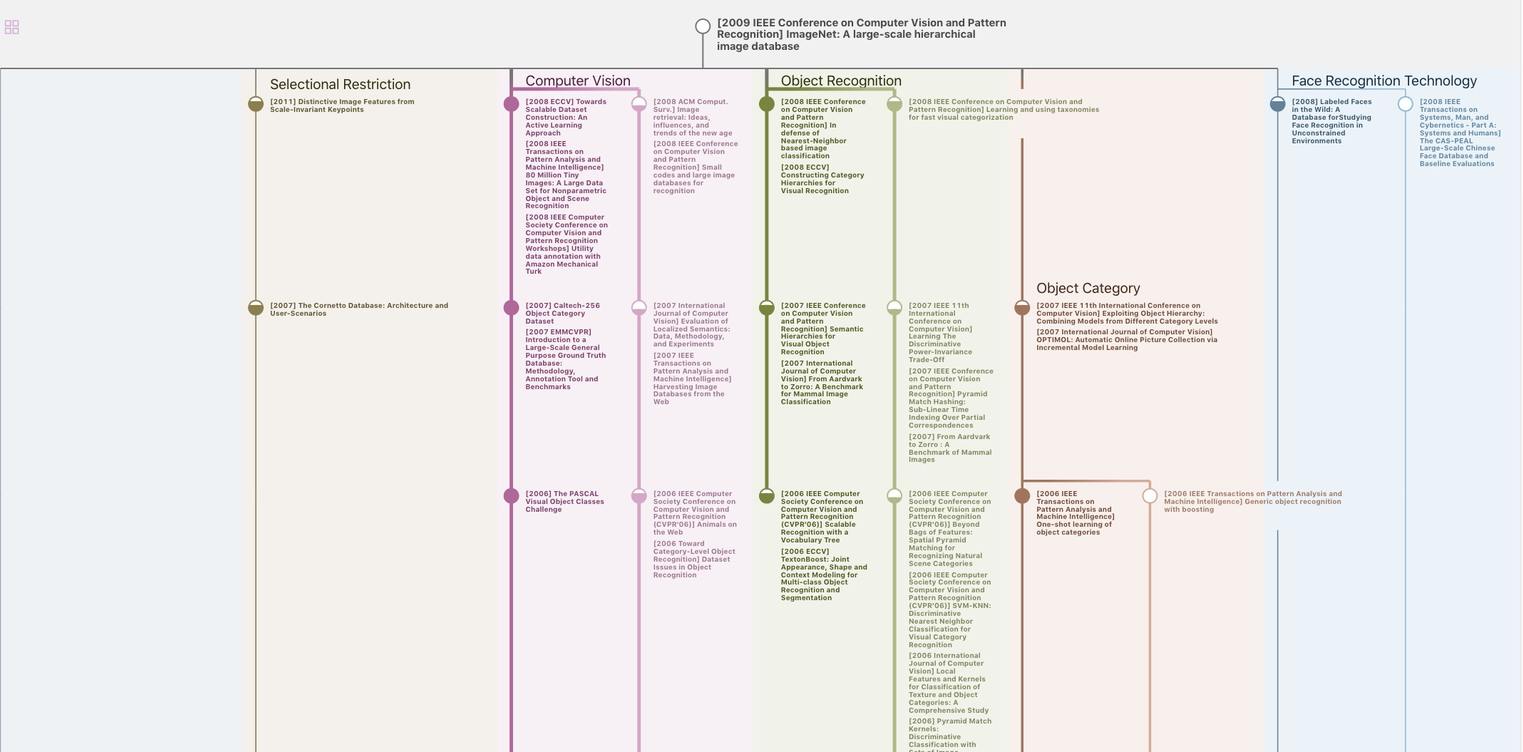
生成溯源树,研究论文发展脉络
Chat Paper
正在生成论文摘要