HATS: Histograms of Averaged Time Surfaces for Robust Event-based Object Classification
2018 IEEE/CVF Conference on Computer Vision and Pattern Recognition(2018)
摘要
Event-based cameras have recently drawn the attention of the Computer Vision community thanks to their advantages in terms of high temporal resolution, low power consumption and high dynamic range, compared to traditional frame-based cameras. These properties make event-based cameras an ideal choice for autonomous vehicles, robot navigation or UAV vision, among others. However, the accuracy of event-based object classification algorithms, which is of crucial importance for any reliable system working in real-world conditions, is still far behind their frame-based counterparts. Two main reasons for this performance gap are: 1. The lack of effective low-level representations and architectures for event-based object classification and 2. The absence of large real-world event-based datasets. In this paper we address both problems. First, we introduce a novel event-based feature representation together with a new machine learning architecture. Compared to previous approaches, we use local memory units to efficiently leverage past temporal information and build a robust event-based representation. Second, we release the first large real-world event-based dataset for object classification. We compare our method to the state-of-the-art with extensive experiments, showing better classification performance and real-time computation.
更多查看译文
关键词
robust event-based object classification,event-based cameras,high temporal resolution,low power consumption,high dynamic range,event-based object classification algorithms,frame-based counterparts,low-level representations,real-world event-based dataset,robust event-based representation,classification performance,event-based feature representation
AI 理解论文
溯源树
样例
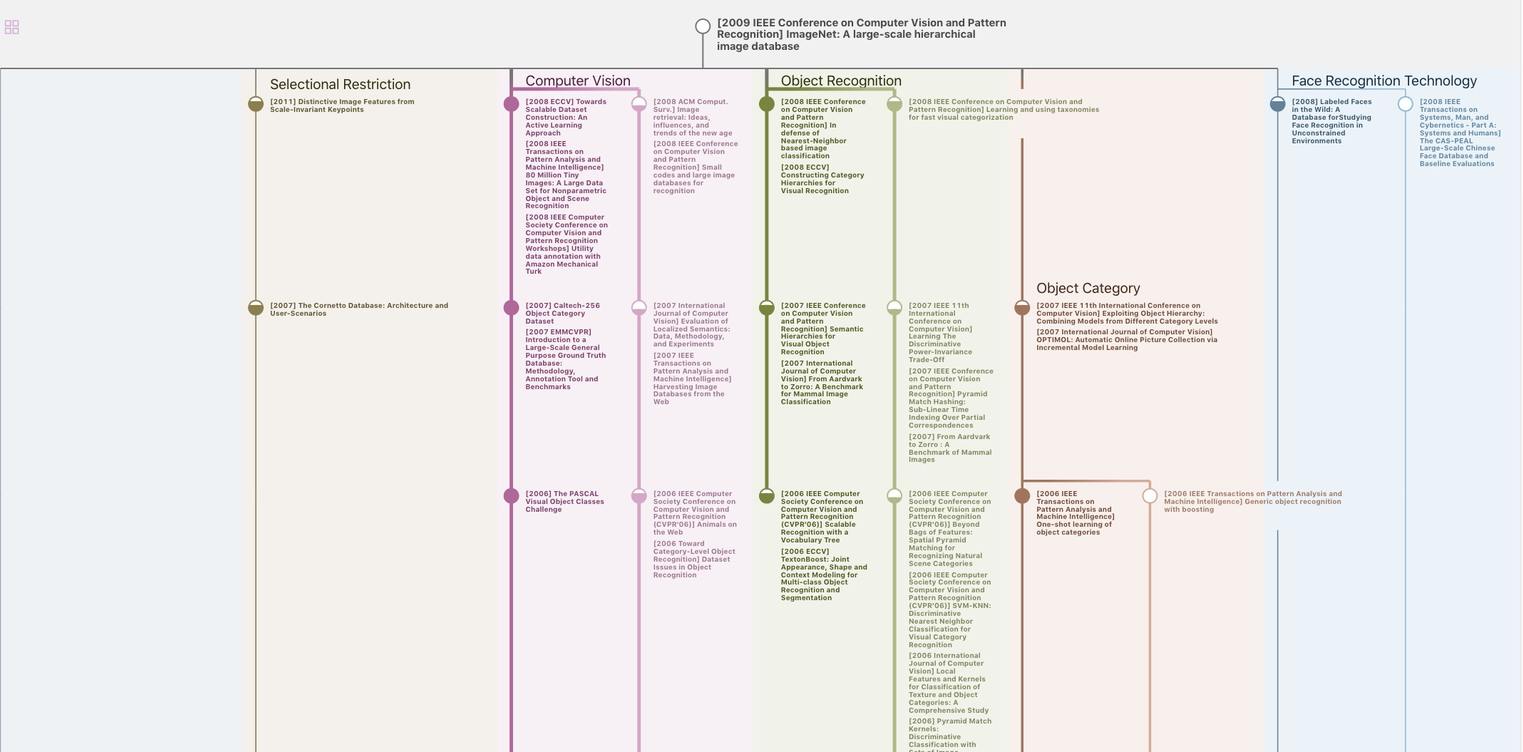
生成溯源树,研究论文发展脉络
Chat Paper
正在生成论文摘要