A Machine Learning Approach for Power Allocation in HetNets Considering QoS
2018 IEEE International Conference on Communications (ICC)(2018)
摘要
There is an increase in usage of smaller cells or femtocells to improve performance and coverage of next-generation heterogeneous wireless networks (HetNets). However, the interference caused by femtocells to neighboring cells is a limiting performance factor in dense HetNets. This interference is being managed via distributed resource allocation methods. However, as the density of the network increases so does the complexity of such resource allocation methods. Yet, unplanned deployment of femtocells requires an adaptable and self-organizing algorithm to make HetNets viable. As such, we propose to use a machine learning approach based on Q-learning to solve the resource allocation problem in such complex networks. By defining each base station as an agent, a cellular network is modelled as a multi-agent network. Subsequently, cooperative Q-learning can be applied as an efficient approach to manage the resources of a multi-agent network. Furthermore, the proposed approach considers the quality of service (QoS) for each user and fairness in the network. In comparison with prior work, the proposed approach can bring more than a four-fold increase in the number of supported femtocells while using cooperative Q-learning to reduce resource allocation overhead.
更多查看译文
关键词
machine learning approach,power allocation,QoS,coverage,next-generation heterogeneous wireless networks,neighboring cells,limiting performance factor,dense HetNets,distributed resource allocation methods,resource allocation problem,complex networks,base station,cellular network,multiagent network,adaptable algorithm,self-organizing algorithm,cooperative Q-learning,resource allocation overhead reduction,femtocells unplanned deployment
AI 理解论文
溯源树
样例
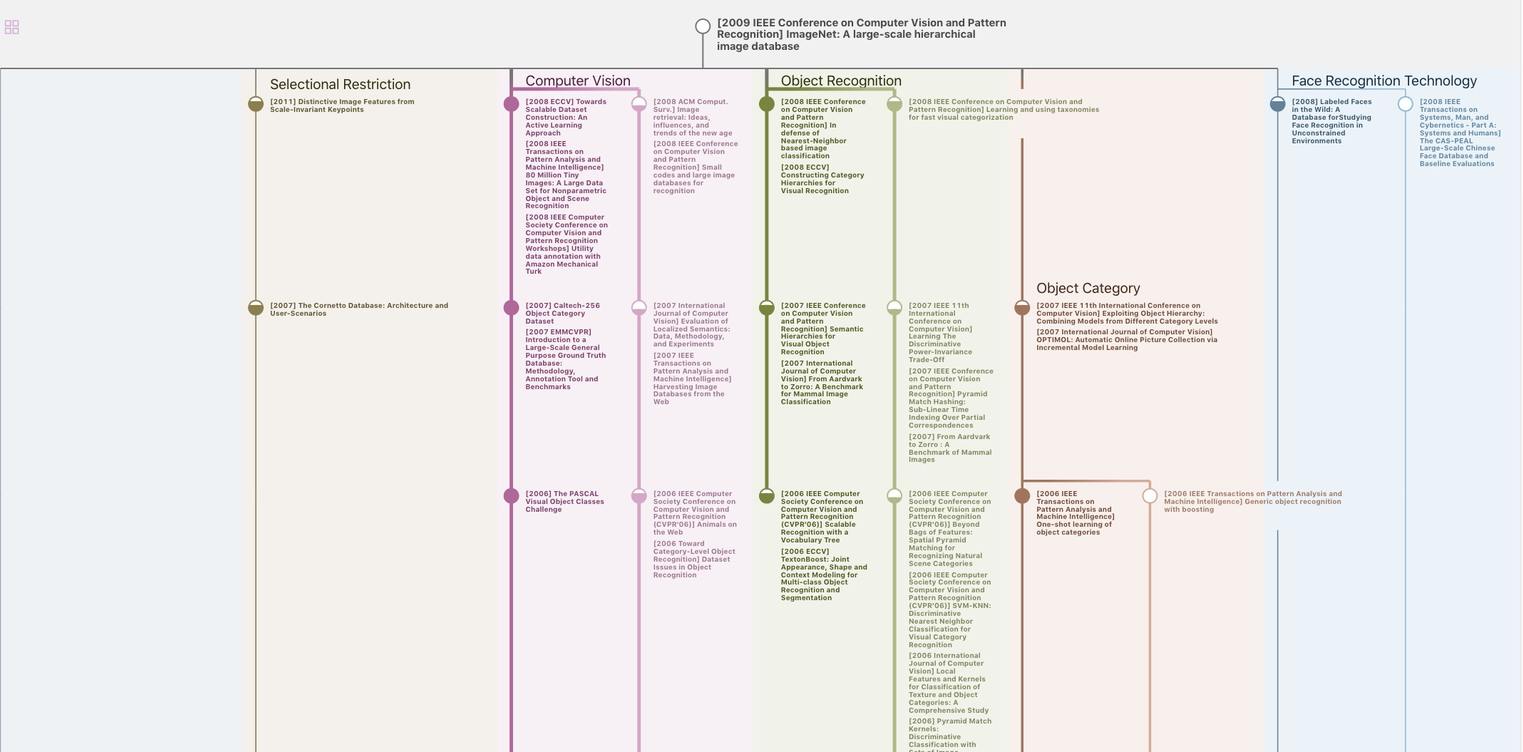
生成溯源树,研究论文发展脉络
Chat Paper
正在生成论文摘要